GPU-accelerated body-internal electric field exposure simulation using low-frequency magnetic field sampling points
COMPEL-THE INTERNATIONAL JOURNAL FOR COMPUTATION AND MATHEMATICS IN ELECTRICAL AND ELECTRONIC ENGINEERING(2023)
摘要
PurposeHigh resolution simulations of body-internal electric field strengths induced by magneto-quasistatic fields from wireless power transfer systems are computationally expensive. The exposure simulation can be split into two separate simulation steps allowing the calculation of the magnetic flux density distribution, which serves as input into the second simulation step to calculate the body-internal electric fields. In this work, the magnetic flux density is interpolated from in situ measurements in combination with the scalar-potential finite difference scheme to calculate the resulting body-internal field. These calculations are supposed to take less than 5 s to achieve a near real-time visualization of these fields on mobile devices. The purpose of this work is to present an implementation of the simulation on graphics processing units (GPUs), allowing for the calculation of the body-internal field strength in about 3 s.Design/methodology/approachThis work uses the co-simulation scalar-potential finite difference scheme to determine the body-internal electric field strength of human models with a voxel resolution of 2 x 2 x 2 mm3. The scheme is implemented on GPUs. This simulation scheme requires the magnetic flux density distribution as input, determined from radial basis functions.FindingsUsing NVIDIA A100 GPUs, the body-internal electric field strength with high-resolution models and 8.9 million degrees of freedom can be determined in about 2.3 s.Originality/valueThis paper describes in detail the used scheme and its implementation to make use of the computational performance of modern GPUs.
更多查看译文
关键词
Augmented reality, Graphics processing unit, Real-time, Scalar-potential finite difference, Wireless power transfer
AI 理解论文
溯源树
样例
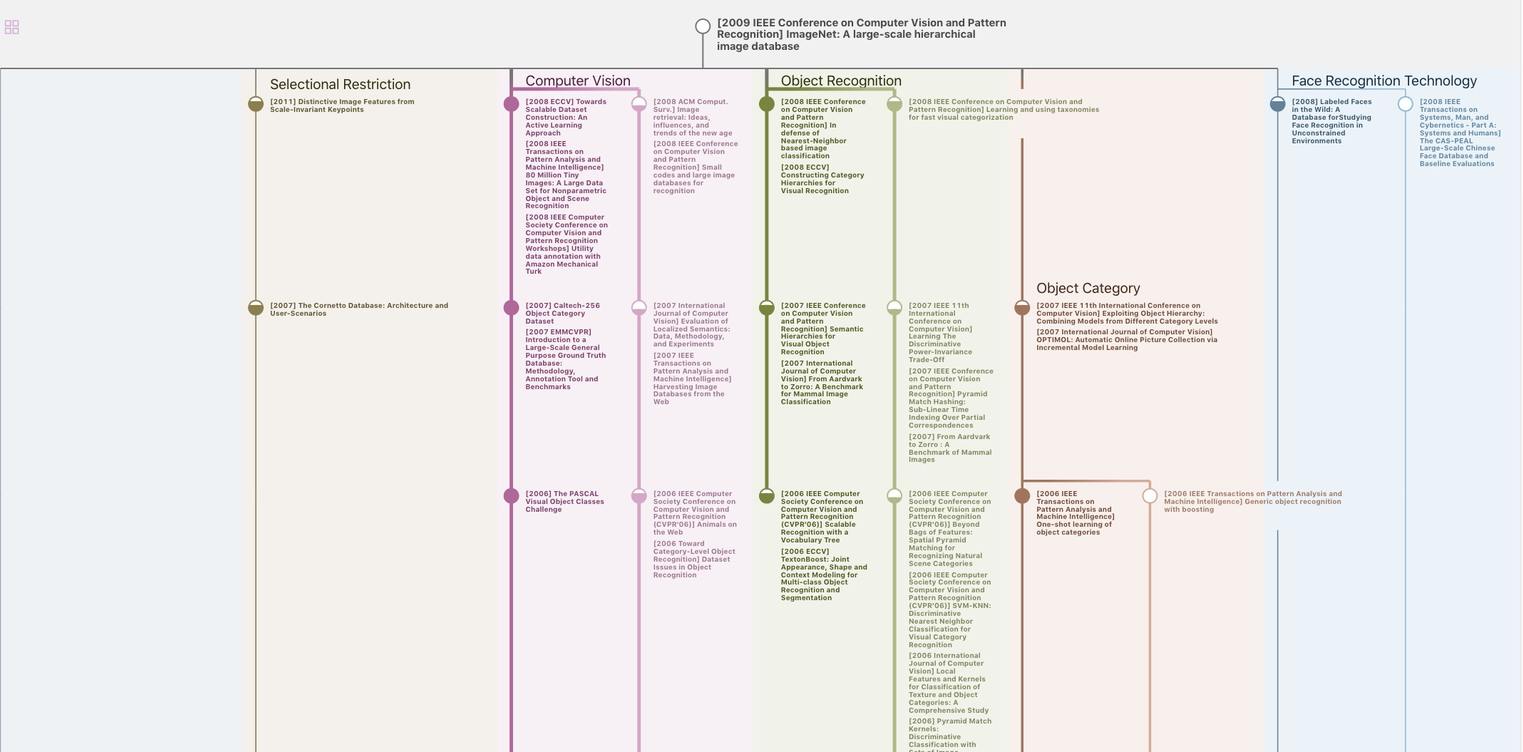
生成溯源树,研究论文发展脉络
Chat Paper
正在生成论文摘要