Data-driven framework for warranty claims forecasting with an application for automotive components
ENGINEERING REPORTS(2023)
摘要
Automakers spend billions of dollars annually towards warranty costs, and warranty reduction is typically high on their priorities. An accurate understanding of warranty performance plays a critical role in controlling and steering the business, and it is of crucial importance to fully understand the actual situation as well as be able to predict future performance, for example, to set up adequate financial reserves or to prioritize improvement actions based on expected forthcoming claims. Data maturation, a major nuisance causing changes in performance metrics with observation time, is one of the factors complicating warranty data analysis and typically leads to over-optimistic conclusions. In this paper, we propose a sequence of steps, decomposing and addressing the main reasons causing data maturation. We first compensate for reporting delay effects using a Cox regression model. For the compensation of heterogeneous build quality, sales delay, and warranty expiration rushes, a constrained quadratic optimization approach is presented, and finally, a sales pattern forecast is provided to properly weigh adjusted individual warranty key performance indicators. The results are shown to dramatically improve prior modeling approaches. Automakers budget for considerable warranty costs, making accurate forecasting vital for financial planning and prioritizing product improvements that lead to reduced costs in future production. This paper presents a data-driven framework for robust warranty forecasting. The framework compensates for reporting delays, heterogeneous build quality, sales delays, and warranty expiration rushes, ultimately improving decision-making and enhancing prior modeling approaches.image
更多查看译文
关键词
dissimilarity,forecasting,machine learning,predictive analytics,proportional hazards,reporting delay
AI 理解论文
溯源树
样例
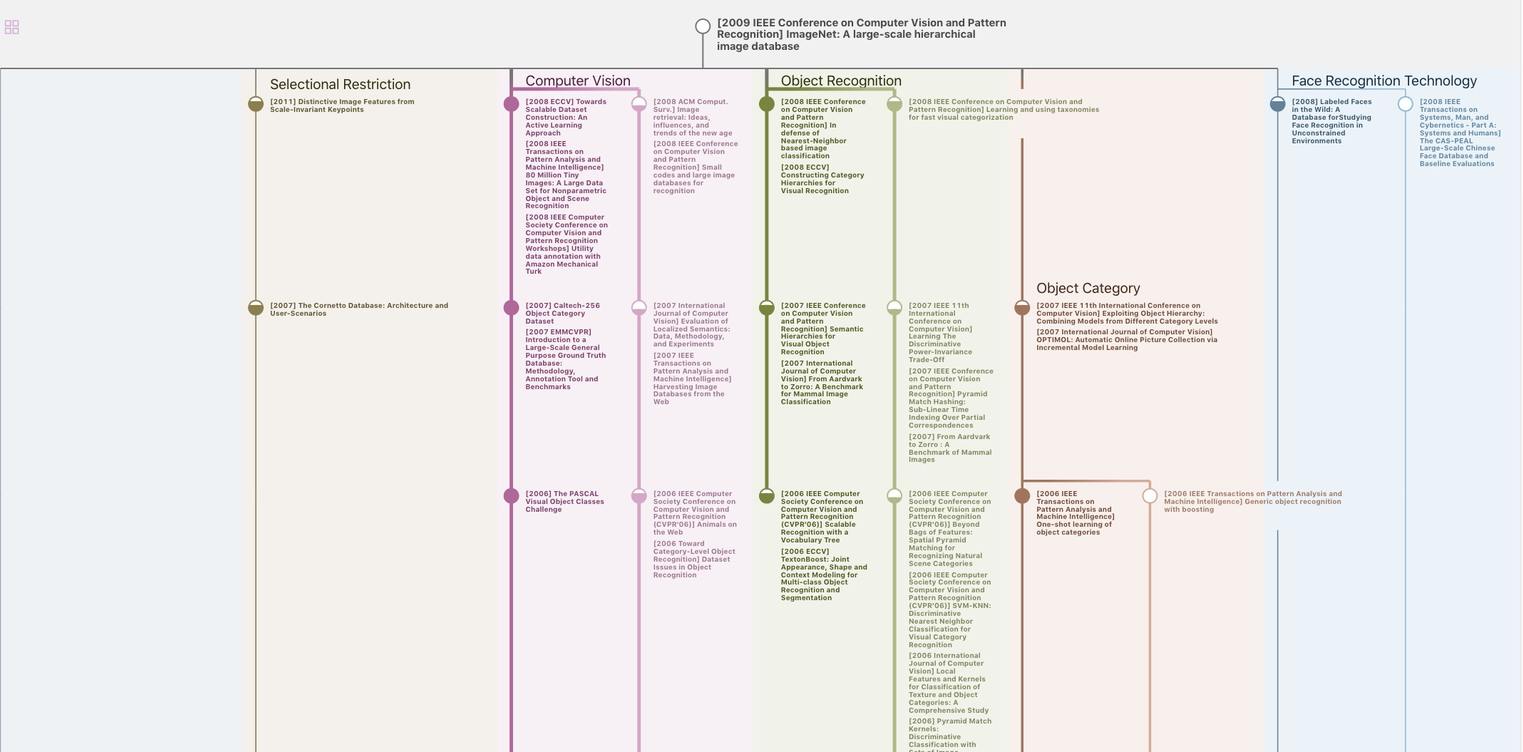
生成溯源树,研究论文发展脉络
Chat Paper
正在生成论文摘要