Bayesian inference of high-dimensional finite-strain visco-elastic-visco-plastic model parameters for additive manufactured polymers and neural network based material parameters generator
INTERNATIONAL JOURNAL OF SOLIDS AND STRUCTURES(2023)
摘要
In this work, the parameters of a finite-strain visco-elastic-visco-plastic formulation with pressure dependency in both the visco-elastic and visco-plastic parts are identified using as observations experimental data obtained from tension and compression tests at different strain rates ranging from 10-4 s-1to 103 s-1. Because of the high number of parameters of the model, a sequential Bayesian Inference (SBI) framework with data augmentation, which presents several advantages, is developed. First the sequential nature reduces the difficulty of selecting the appropriate prior distributions by considering only parts of the observations at a time. Second, the sequential nature prevents dealing with low likelihood values by considering only a part of the experimental observations at a time, but also subsets of the material parameters to be identified, improving the convergence of the Markov Chain Monte Carlo (MCMC) random walk. Third, the data augmentation allows considering different number of experimental tests in tension and in compression while preserving the identified model accuracy for both loading modes. This SBI is carried out to infer the properties of Polyamide 12 (PA12) processed by Selective Laser Sintering (SLS) for two different printing directions and it is shown that the models fed by their respective set of inferred parameters can reproduce the different experimental tests. Finally, in order for upcoming structural simulations to benefit from the information related to the uncertainties due to the measurement errors, the identification process and the model limitations, we introduce a Generative Adversarial Network (GAN), which is trained using the data obtained from the MCMC random walk. This generator can then serve to produce a synthetic data-set of arbitrary size of the material parameters to be used in finite-element simulations.
更多查看译文
关键词
Bayesian inference,Visco-elasticity–visco-plasticity,Selective laser sintering,Polyamide,Generative adversarial network
AI 理解论文
溯源树
样例
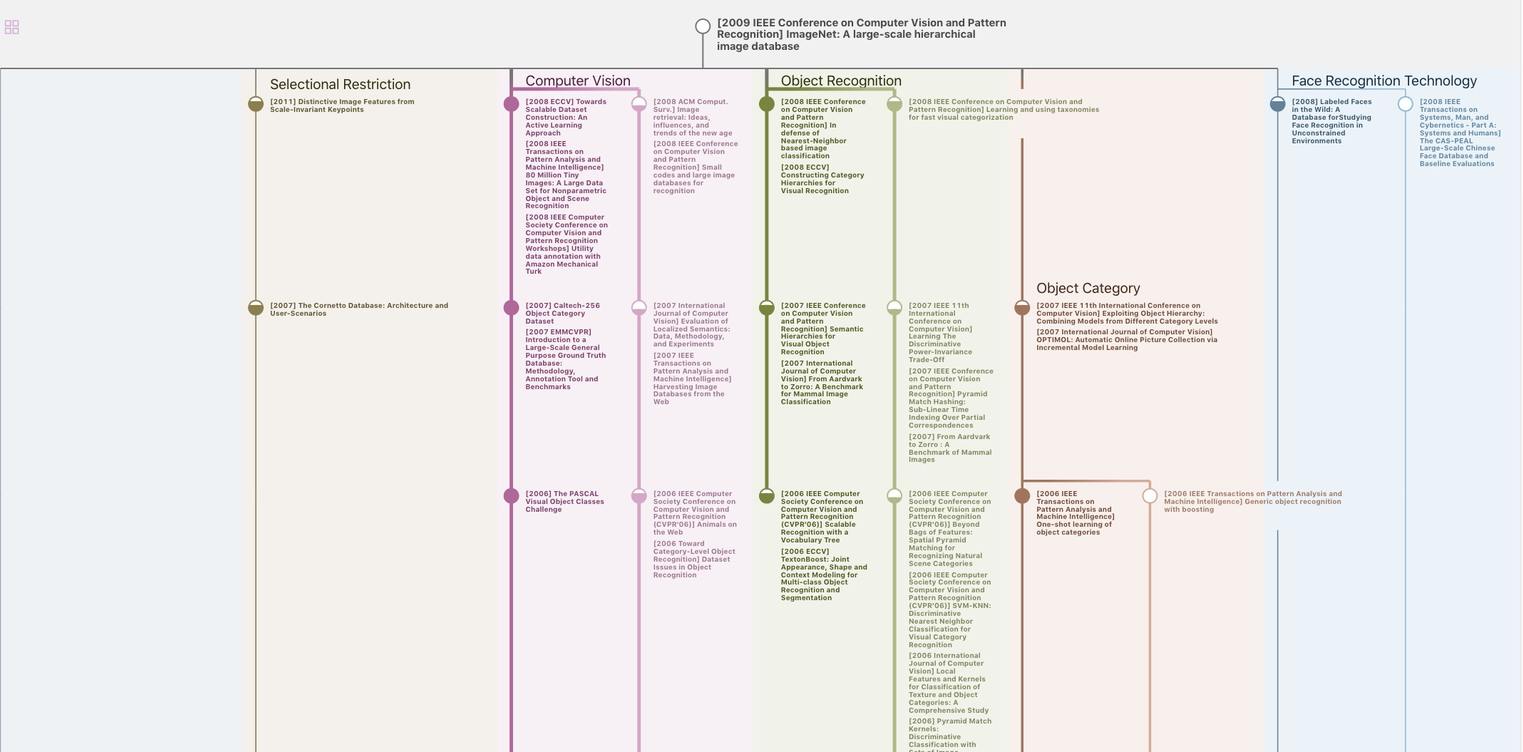
生成溯源树,研究论文发展脉络
Chat Paper
正在生成论文摘要