Temperature distribution prediction in longitudinal ballastless slab track with various neural network methods
SMART STRUCTURES AND SYSTEMS(2023)
摘要
The temperature prediction approaches of three important locations in an operational longitudinal slab track-bridge structure by using three typical neural network methods based on the field measuring platform of four meteorological factors and internal temperature. The measurement experiment of four meteorological factors (e.g., ambient temperature, solar radiation, wind speed, and humidity) temperature in the three locations of the longitudinal slab and base plate of three important locations (e.g., mid-span, beam end, and Wide-Narrow Joint) were conducted, and then their characteristics were analyzed, respectively. Furthermore, temperature prediction effects of three locations under five various meteorological conditions are tested by using three neural network methods, respectively, including the Artificial Neural Network (ANN), the Long Short-Term Memory (LSTM), and the Convolutional Neural Network (CNN). More importantly, the predicted effects of solar radiation in four meteorological factors could be identified with three indicators (e.g., Root Means Square Error, Mean Absolute Error, Correlation Coefficient of R2). In addition, the LSTM method shows the best performance, while the CNN method has the best prediction effect by only considering a single meteorological factor.
更多查看译文
关键词
ballastless track-bridge structure, field measuring platform, meteorological factors, neural network methods, temperature prediction
AI 理解论文
溯源树
样例
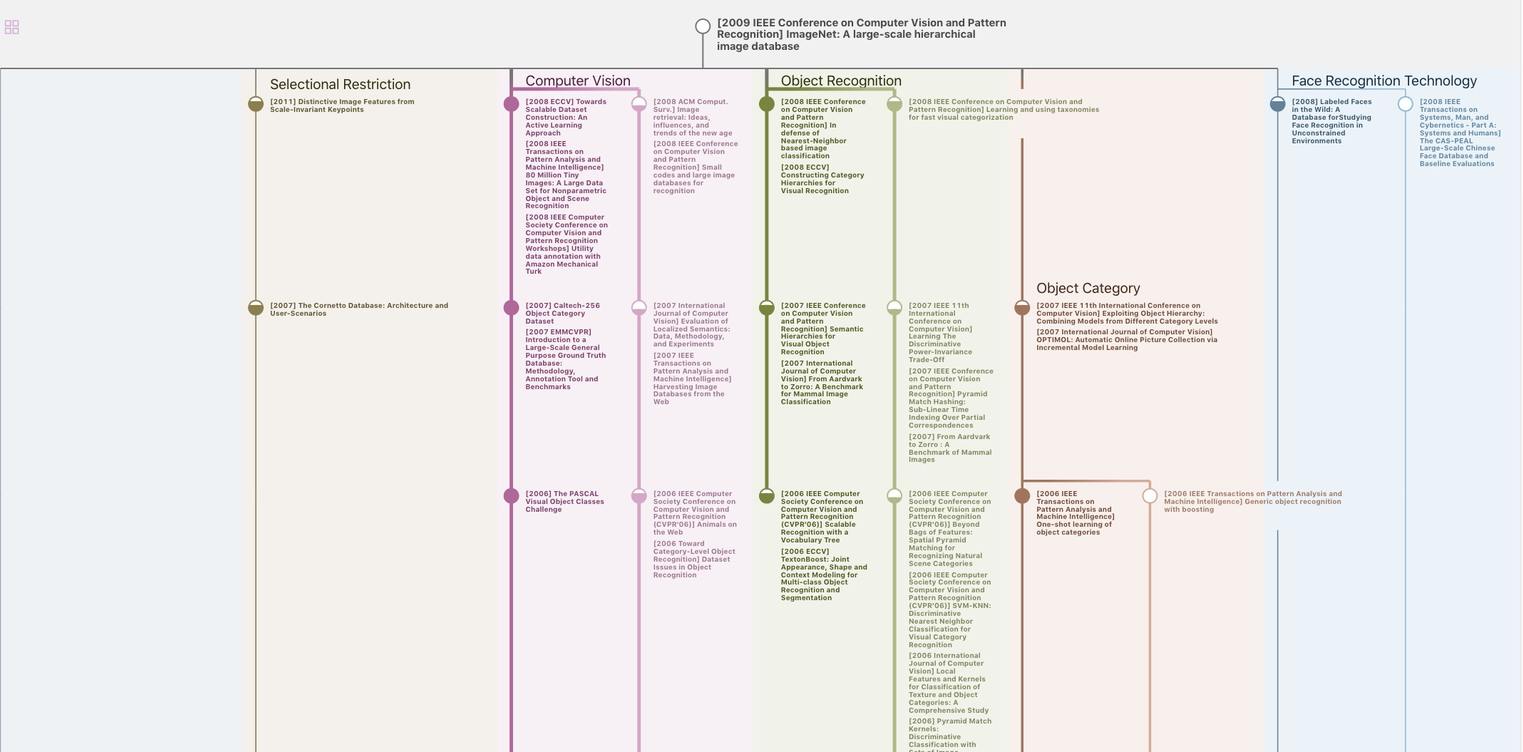
生成溯源树,研究论文发展脉络
Chat Paper
正在生成论文摘要