Track Unevenness Prediction Based on Static Track Inspection Data Matching
JOURNAL OF NANOELECTRONICS AND OPTOELECTRONICS(2023)
摘要
A track static misalignment prediction model based on track median deviation is created using an IGA-BP neural network in order to precisely predict the trend of ballastless track static misalignment. The historical static track median deviation detection data are matched using actual compensation edit distance (ERP) to finish the correspondence processing of the original data. The precisely matched data are used to train the model, forecast irregularities in the track median, and compare results with other traditional prediction techniques. The outcomes demonstrate that the IGA-BP neural network can more accurately predict the nonlinear time series data development trend. In comparison to other prediction models, the IGA-BP neural network model's average relative error and root mean square error are 0.091 and 0.110, respectively. The prediction accuracy is raised by between 43% and 60%, demonstrating the IGA-BP neural network model's efficacy in predicting static upsets on ballastless tracks and presenting a workable strategy for track predictive maintenance.
更多查看译文
关键词
Centerline, Vertical Deviation, Neural Network, Predictive Maintenance.
AI 理解论文
溯源树
样例
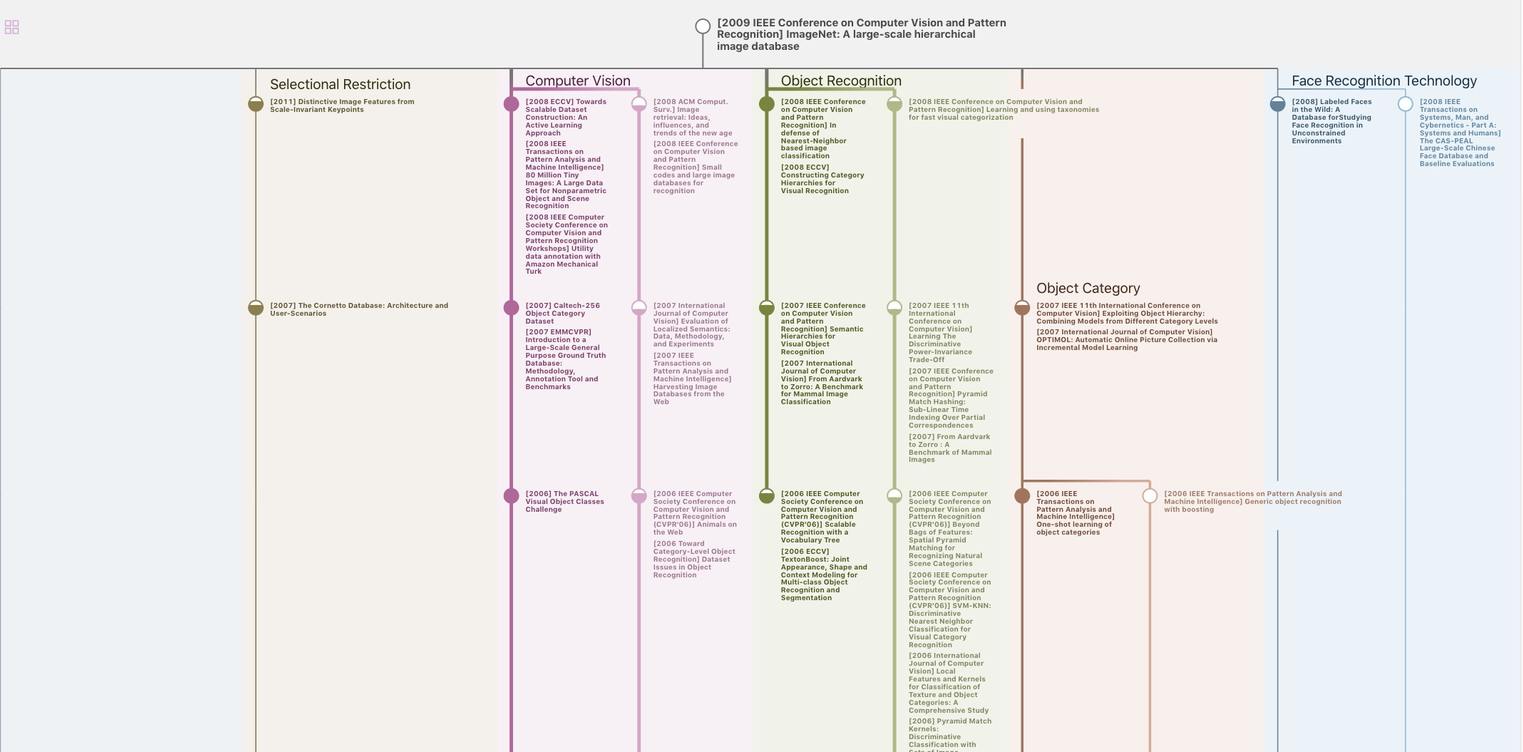
生成溯源树,研究论文发展脉络
Chat Paper
正在生成论文摘要