Deciphering flow clusters from large-scale free-floating bike sharing journey data: a two-stage flow clustering method
TRANSPORTATION(2023)
摘要
Extracting flow clusters consisting of many similar origin-destination (OD) trips is essential to uncover the spatio-temporal interactions and mobility patterns in the free-floating bike sharing (FFBS) system. However, due to occlusion and display clutter issues, efforts to identify inhomogeneous flow clusters from large journey data have been hampered to some extent. In this study, we present a two-stage flow clustering method, which integrates the Leiden community detection algorithm and the shared nearest-neighbor-based flow (SNN_flow) clustering method to efficiently identify flow clusters with arbitrary shapes and uneven densities. The applicability and performance of the method in detecting flow clusters are investigated empirically using the FFBS system of Nanjing, China as a case study. Some interesting findings can be drawn from the spatio-temporal patterns. For instance, the share of flow clusters used to meet the "first-/last-mile" demand at metro stations is reasonably high, both during the morning (71.85%) and evening (65.79%) peaks. Compared with the "first-/last-mile" flow clusters between metro stations and adjacent workplaces, the solution of the "first-/last-mile" flow clusters between metro stations and adjacent residences is more dependent on the FFBS system. In addition, we explored the shape and density distribution of flow clusters from the perspective of origin and destination points. The endpoint distribution characteristics demonstrate that the shape distribution of metro station point clusters is generally flatter and the spatial points within them are more concentrated than other sorts of point clusters. Our findings could help to better understand human movement patterns and home-work commute, thereby providing more rational and targeted decisions for allocating FFBS infrastructure resources.
更多查看译文
关键词
Free-floating bike sharing,Origin-destination flow,Clustering,Commuting trips,Spatio-temporal pattern
AI 理解论文
溯源树
样例
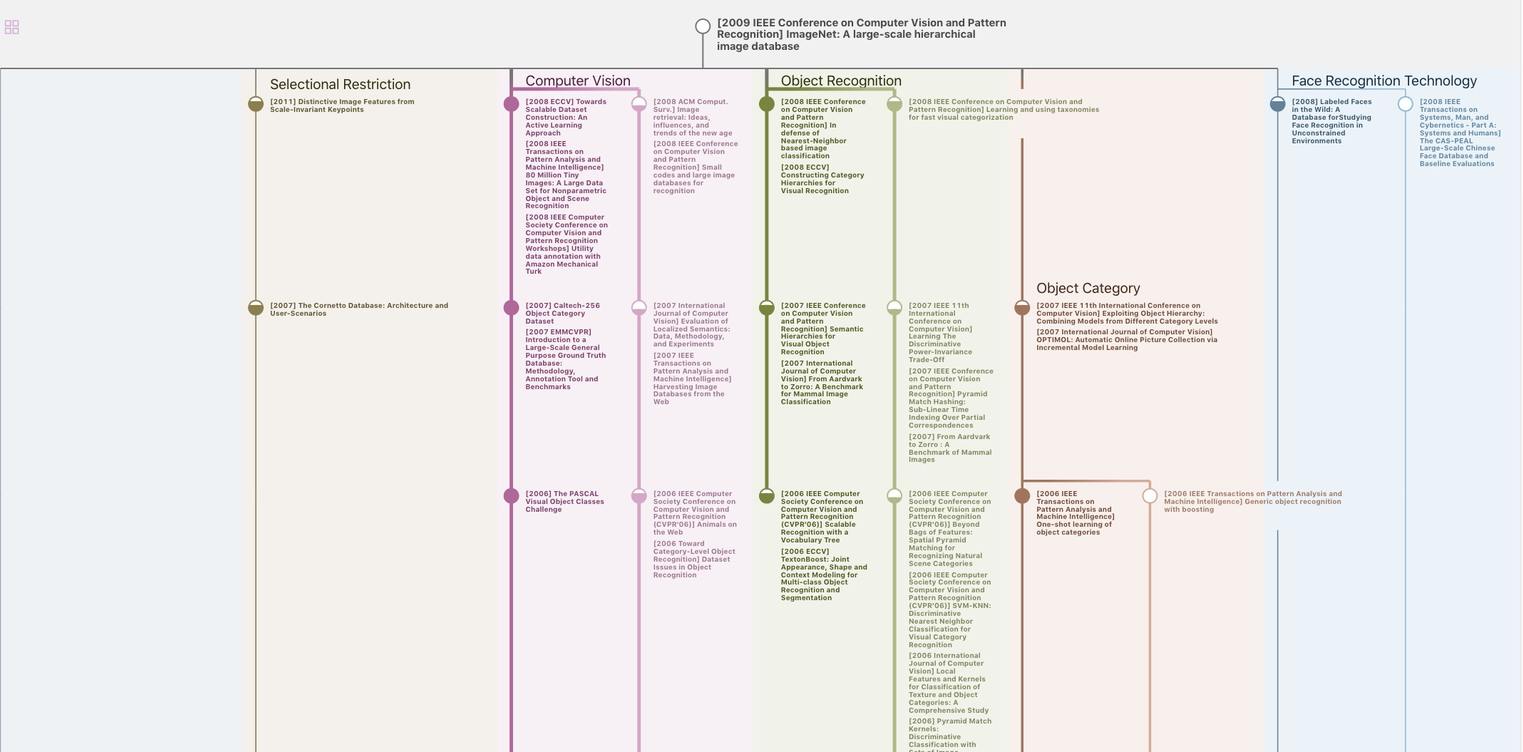
生成溯源树,研究论文发展脉络
Chat Paper
正在生成论文摘要