Random Forest Analysis of Combined Millimeter-Wave and Near-Infrared Sensing for Noninvasive Glucose Detection
IEEE SENSORS JOURNAL(2023)
摘要
Recently, great progress has been achieved in diabetes management through minimally invasive continuous glucose monitoring, which involves piercing the skin to identify changes in glucose levels in the interstitial fluid. Nevertheless, the development of accurate fully noninvasive (NI) glucose monitors remains a topic of great interest. This work examines the processing. data collected from a multi-sensor system, which combines millimeter-wave (mm-wave), near-infrared (NIR), and other auxiliary sensors' technology, coupled with a random forest machine learning (ML) model for prediction. We investigated possible correlations between mm-wave, NIR, skin temperature, pressure data, and blood glucose values and deployed an ML model for prediction. The model is based on the random forest algorithm that was trained and tested on data collected from a study involving five healthy human subjects undergoing an intravenous glucose tolerance test. Our model achieves a root mean square error of 21.06 mg/dl and a mean absolute relative difference of 7.31% for glucose prediction in the collected clinical subjects dataset. In addition, 96.1% of the model's predictions fall within the clinically acceptable zones according to the Clarke error grid analysis. The preliminary results suggest that combining multiple sensor data with ML techniques is promising for the development of NI glucose monitoring.
更多查看译文
关键词
glucose,sensing,forest,millimeter-wave,near-infrared,non-invasive
AI 理解论文
溯源树
样例
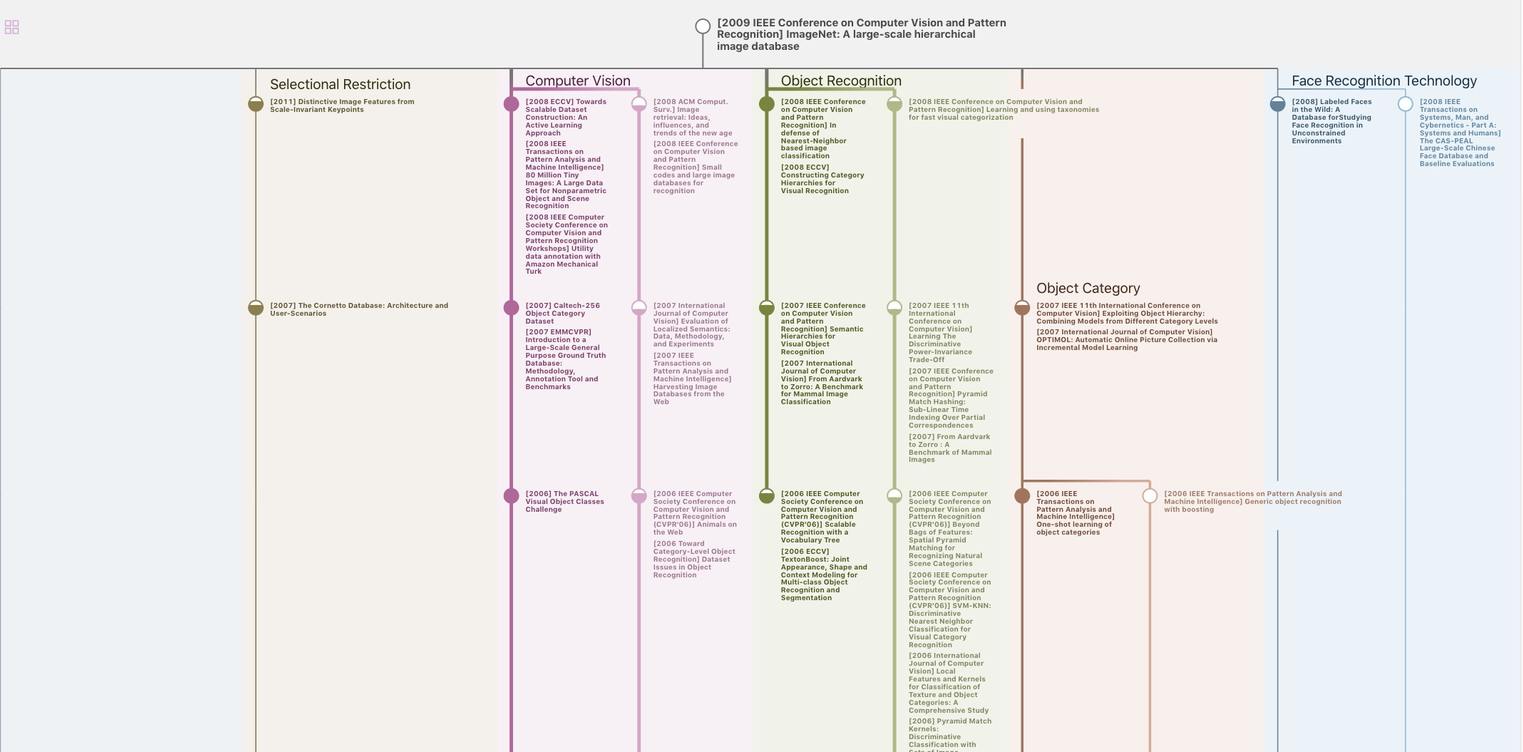
生成溯源树,研究论文发展脉络
Chat Paper
正在生成论文摘要