Comprehensive deep learning-based framework for automatic organs-at-risk segmentation in head-and-neck and pelvis for MR-guided radiation therapy planning
FRONTIERS IN PHYSICS(2023)
摘要
Introduction: The excellent soft-tissue contrast of magnetic resonance imaging (MRI) is appealing for delineation of organs-at-risk (OARs) as it is required for radiation therapy planning (RTP). In the last decade there has been an increasing interest in using deep-learning (DL) techniques to shorten the labor-intensive manual work and increase reproducibility. This paper focuses on the automatic segmentation of 27 head-and-neck and 10 male pelvis OARs with deep-learning methods based on T2-weighted MR images.Method: The proposed method uses 2D U-Nets for localization and 3D U-Net for segmentation of the various structures. The models were trained using public and private datasets and evaluated on private datasets only.Results and discussion: Evaluation with ground-truth contours demonstrated that the proposed method can accurately segment the majority of OARs and indicated similar or superior performance to state-of-the-art models. Furthermore, the auto-contours were visually rated by clinicians using Likert score and on average, 81% of them was found clinically acceptable.
更多查看译文
关键词
radiation therapy,learning-based,organs-at-risk,head-and-neck,mr-guided
AI 理解论文
溯源树
样例
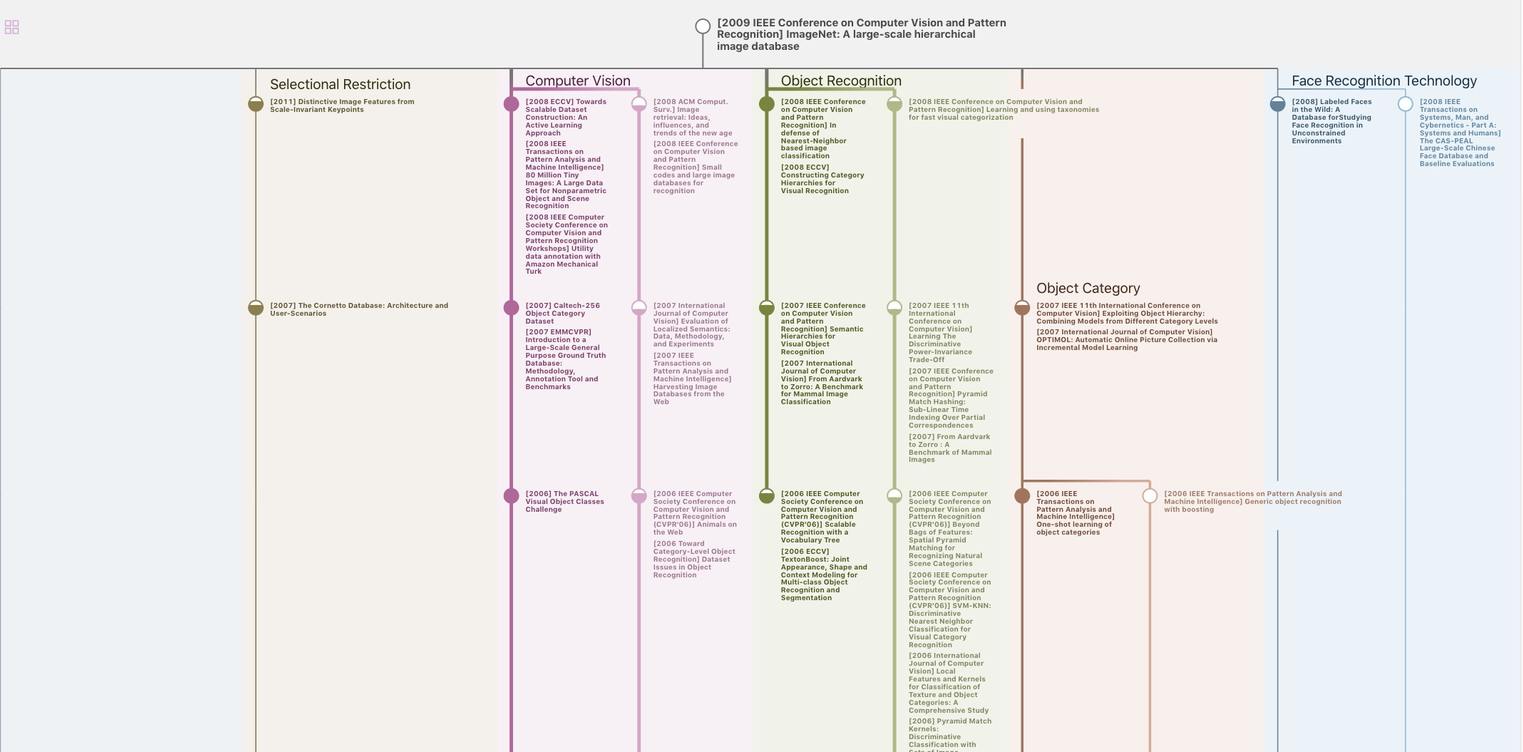
生成溯源树,研究论文发展脉络
Chat Paper
正在生成论文摘要