PRIME: a probabilistic neural network approach to solar wind propagation from L1
FRONTIERS IN ASTRONOMY AND SPACE SCIENCES(2023)
摘要
Introduction: For the last several decades, continuous monitoring of the solar wind has been carried out by spacecraft at the first Earth-Sun Lagrange point (L1). Due to computational expense or model limitations, those data often must be propagated to some point closer to the Earth in order to be usable by those studying the interaction between Earth's magnetosphere and the solar wind. The current most widely used tool to propagate measurements from L1 (roughly 235 RE upstream) to Earth is the planar propagation method, which includes a number of known limitations. Motivated by these limitations, this study introduces a new algorithm called the Probabilistic Regressor for Input to the Magnetosphere Estimation (PRIME).Methods: PRIME is based on a novel probabilistic recurrent neural network architecture, and is capable of incorporating solar wind time history from L1 monitors to generate predictions of near-Earth solar wind as well as estimate uncertainties for those predictions.Results: A statistical validation shows PRIME's predictions better match MMS magnetic field and plasma measurements just upstream of the bow shock than measurements from Wind propagated to MMS with a minimum variance analysis-based planar propagation technique. PRIME's continuous rank probability score (CRPS) is 0.214 sigma on average across all parameters, compared to the minimum variance algorithm's CRPS of 0.350 sigma. PRIME's performance improvement over minimum variance is dramatic in plasma parameters, with an improvement in CRPS from 2.155 cm-3 to 0.850 cm-3 in number density and 16.15 km/s to 9.226 km/s in flow velocity VX GSE.Discussion: Case studies of particularly difficult to predict or extreme conditions are presented to illustrate the benefits and limitations of PRIME. PRIME's uncertainties are shown to provide reasonably reliable predictions of the probability of particular solar wind conditions occurring.Conclusion: PRIME offers a simple solution to common limitations of solar wind propagation algorithms by generating accurate predictions of the solar wind at Earth with physically meaningful uncertainties attached.
更多查看译文
关键词
solar wind propagation,probabilistic neural network approach
AI 理解论文
溯源树
样例
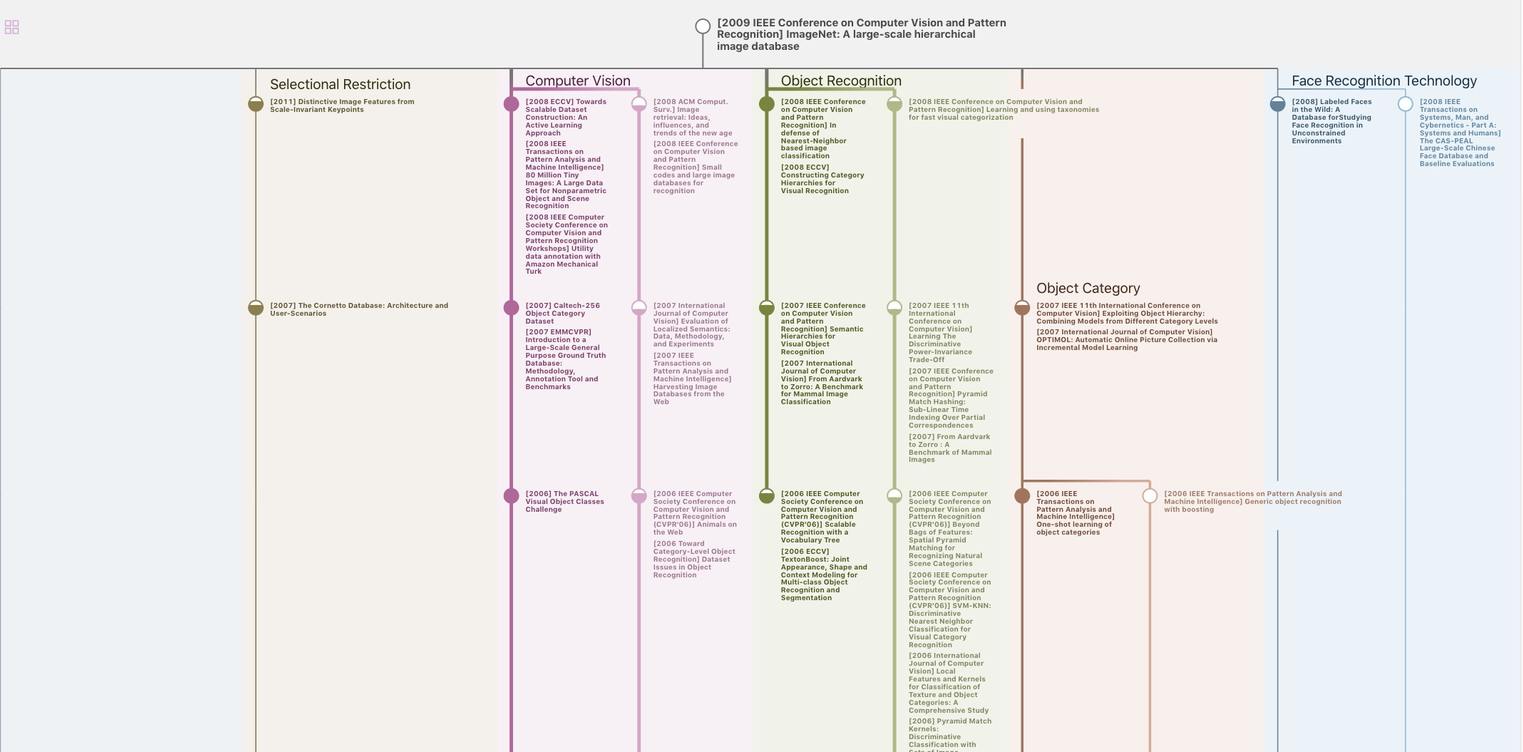
生成溯源树,研究论文发展脉络
Chat Paper
正在生成论文摘要