Image presentation and effective classification of odor intensity levels using multi-channel electronic nose technology combined with GASF and CNN
SENSORS AND ACTUATORS B-CHEMICAL(2023)
摘要
This paper proposes a novel data processing scheme for electronic noses that combines the gramian angular field (GAF) and convolutional neural network (CNN) to achieve high performance in classifying five levels of odor intensity. Specifically, a multi-channel e-nose was developed to detect various gases, including hydrogen sulfide, ammonia, sulfur dioxide, trimethylamine, and alkane gases, among others, in complex odor components. The sensor array was optimized through Spearman correlation analysis of the sensor signals and artificial olfactory odor intensity levels. Moreover, the one-dimensional temporal sensor data was converted into two-dimensional color images using the GAF (GASF/GADF) algorithm. This approach enables a more detailed presentation of deep features while retaining the time-domain dependence of the signals. To enhance the performance of classification, a multi-scale feature fusion network (MFFNet) was designed. Notably, GASF-converted images are more effective in characterizing sensor data for different odor intensity levels than GADF-converted images. Compared to classical CNN classification models such as AlexNet, GoogLeNet, and ResNet18, MFFNet achieved the highest accuracy and macro average F1-score on the test set, which were 93.75% and 93.34%, respectively. The results demonstrate the efficient classification of odor intensity levels by combining multi-channel e-nose technology, GASF, and CNN.
更多查看译文
关键词
Electronic nose,GAF(GASF/GADF),Odor intensity levels,CNN,Sensor data processing
AI 理解论文
溯源树
样例
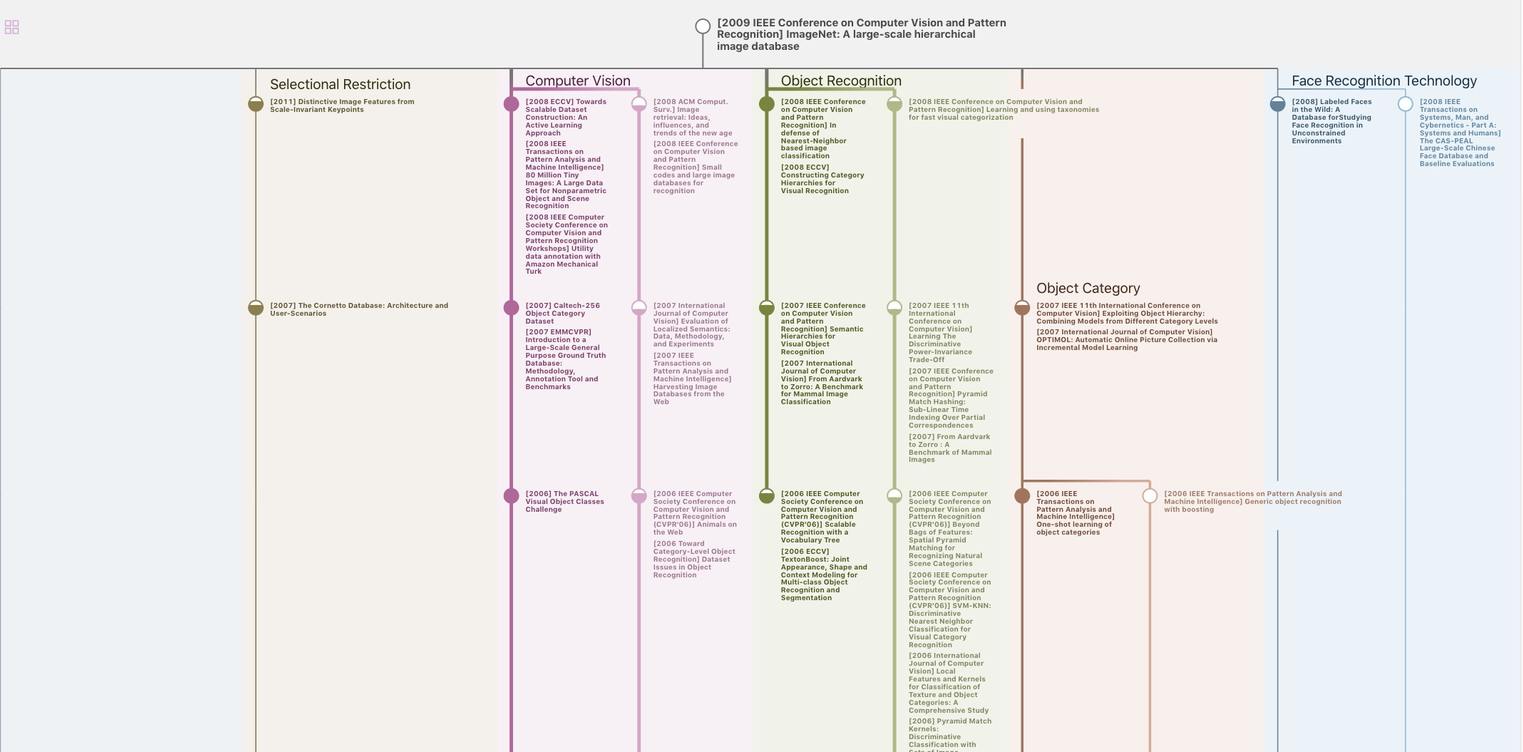
生成溯源树,研究论文发展脉络
Chat Paper
正在生成论文摘要