A change-point-based control chart for detecting sparse mean changes in high-dimensional heteroscedastic data
JOURNAL OF QUALITY TECHNOLOGY(2024)
摘要
Because of the "curse of dimensionality," high-dimensional processes present challenges to traditional multivariate statistical process monitoring (SPM) techniques. In addition, the unknown underlying distribution of and complicated dependency among variables such as heteroscedasticity increase the uncertainty of estimated parameters and decrease the effectiveness of control charts. In addition, the requirement of sufficient reference samples limits the application of traditional charts in high-dimension, low-sample-size scenarios (small n, large p). More difficulties appear when detecting and diagnosing abnormal behaviors caused by a small set of variables (i.e., sparse changes). In this article, we propose two change-point-based control charts to detect sparse shifts in the mean vector of high-dimensional heteroscedastic processes. Our proposed methods can start monitoring when the number of observations is a lot smaller than the dimensionality. The simulation results show that the proposed methods are robust to nonnormality and heteroscedasticity. Two real data examples are used to illustrate the effectiveness of the proposed control charts in high-dimensional applications. The R codes are provided online.
更多查看译文
关键词
change-point,heteroscedasticity,high-dimensional control chart,sparse changes,statistical process monitoring (SPM)
AI 理解论文
溯源树
样例
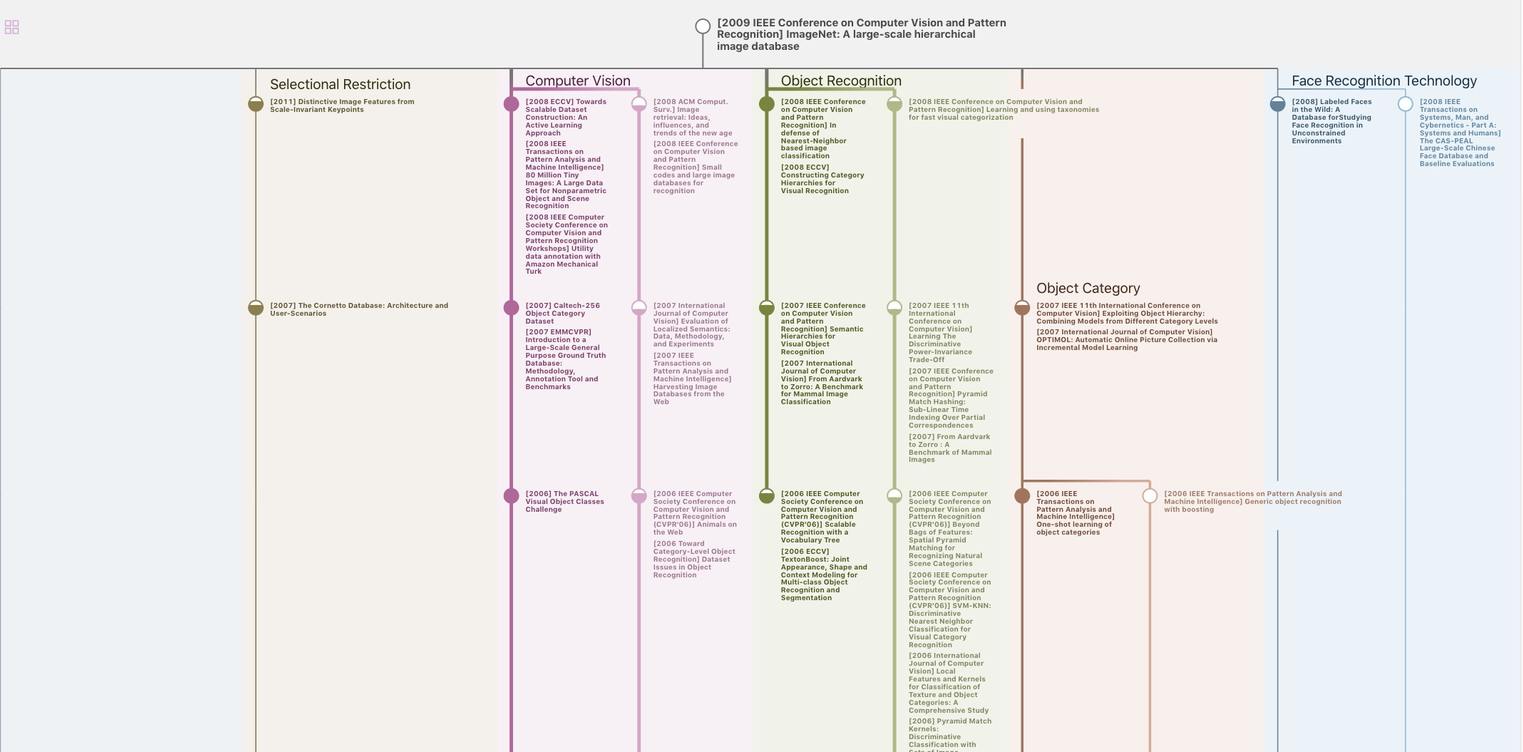
生成溯源树,研究论文发展脉络
Chat Paper
正在生成论文摘要