Prediction of Heat Transfer Coefficient and Pressure Drop of R1234yf and R134a Flow Condensation in Horizontal and Inclined Tubes Using Machine Learning Techniques
International journal of refrigeration(2023)
摘要
Machine learning techniques have great potential to predict two-phase flow characteristics instead of classic empirical correlations. In the present study, four different machine learning models, including multi-layer perceptron artificial neural network (ANNMLP), support vector regression (SVR), K nearest neighbors (KNN), and extreme gradient boosting (XGBoost), are employed to predict the heat transfer coefficient (HTC) and the frictional pressure drop (FPD) of R134a and R1234yf condensation flow in horizontal and inclined tubes. The dataset includes 348 points from previous works and the current research. To extend the data, an experimental study is also performed on the condensation of R134a in a horizontal tube for different mass velocities and vapor qualities. The results show that, in the best model, HTC can be estimated by ANNMLP with the mean absolute percentage error (MAPE) of 7.01%. The best prediction of FPD is achieved using XGBoost machine with MAPE of 10.87% on test data. Also, the feature importance procedure is implemented to recognize the most useful features. Based on the results, the mass velocity and the inclination angle are identified as the most influencing parameters on the prediction of HTC and FPD, respectively.
更多查看译文
关键词
Artificial neural network,Support vector regression,K nearest neighbors,Extreme gradient boosting,Two-phase flow
AI 理解论文
溯源树
样例
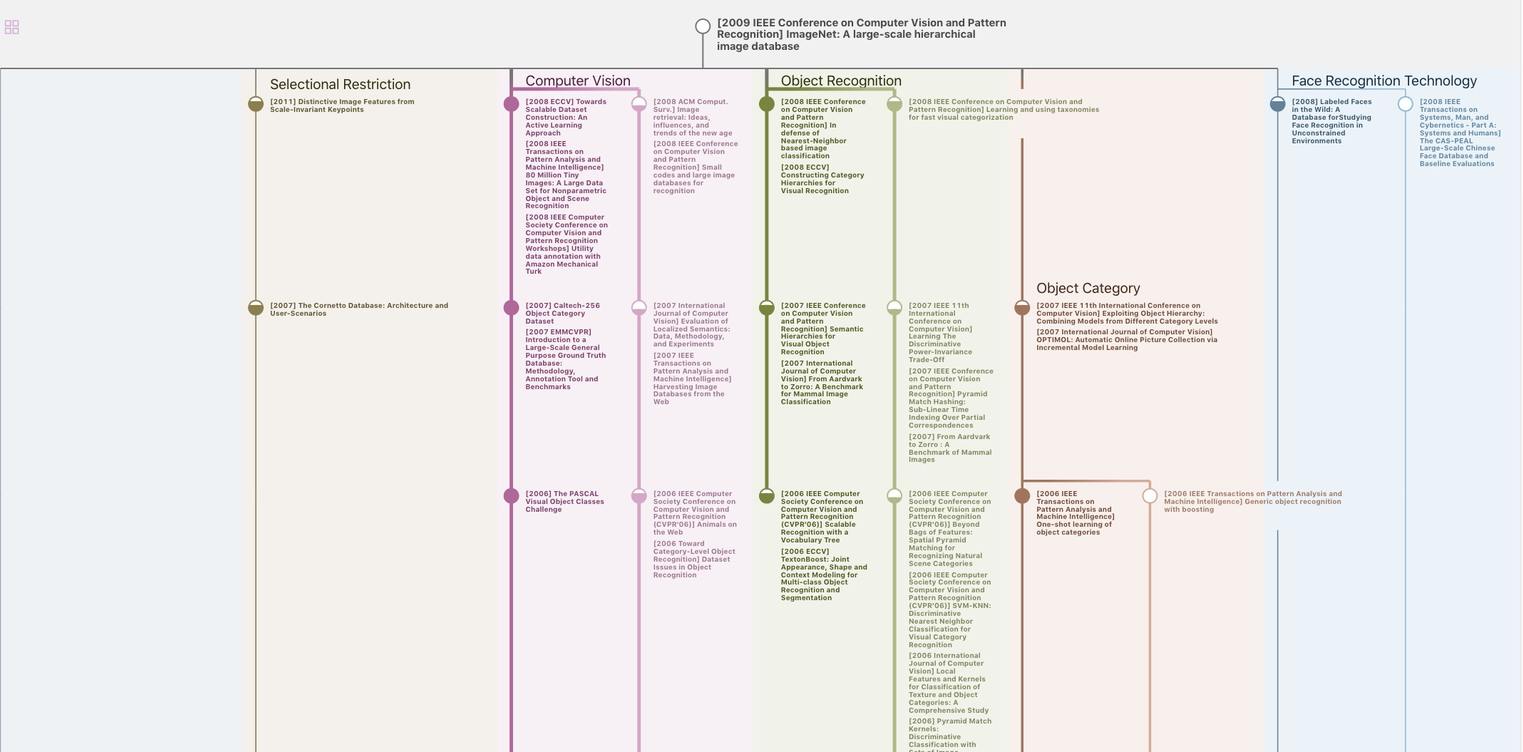
生成溯源树,研究论文发展脉络
Chat Paper
正在生成论文摘要