A Study on Data Generation Methods for Defect Diagnosis Accuracy Enhancement in the Directed Energy Deposition Process
TRANSACTIONS OF THE KOREAN SOCIETY OF MECHANICAL ENGINEERS A(2023)
摘要
To ensure the quality reliability of the directed energy deposition (DED) process, recent research has focused on artificial intelligence-based defect diagnosis algorithms that utilize sensor data. For these algorithms, a considerable number of normal/abnormal training data are required; however, acquiring a large amount of data is difficult owing to the lengthy processing time and expensive cost of metal powder required for the DED process. Thus, multiple process data generating methods for the DED process are proposed in this study. First, normal and abnormal data in class imbalance states are gathered by the multisensor-based DED process monitoring system, followed by the development of statistical and machine/deep learning-based data generating algorithms. Furthermore, the cluster distribution between the generated and real process data is compared using the t-SNE-based dimensional reduction technique and the validity of the generated data is confirmed using the diagnosis model. The possibility of overcoming the problem of poor diagnosis accuracy due to class imbalance is then confirmed.
更多查看译文
关键词
image intensifier tube,reliability test,illuminance,induced-photocurrent
AI 理解论文
溯源树
样例
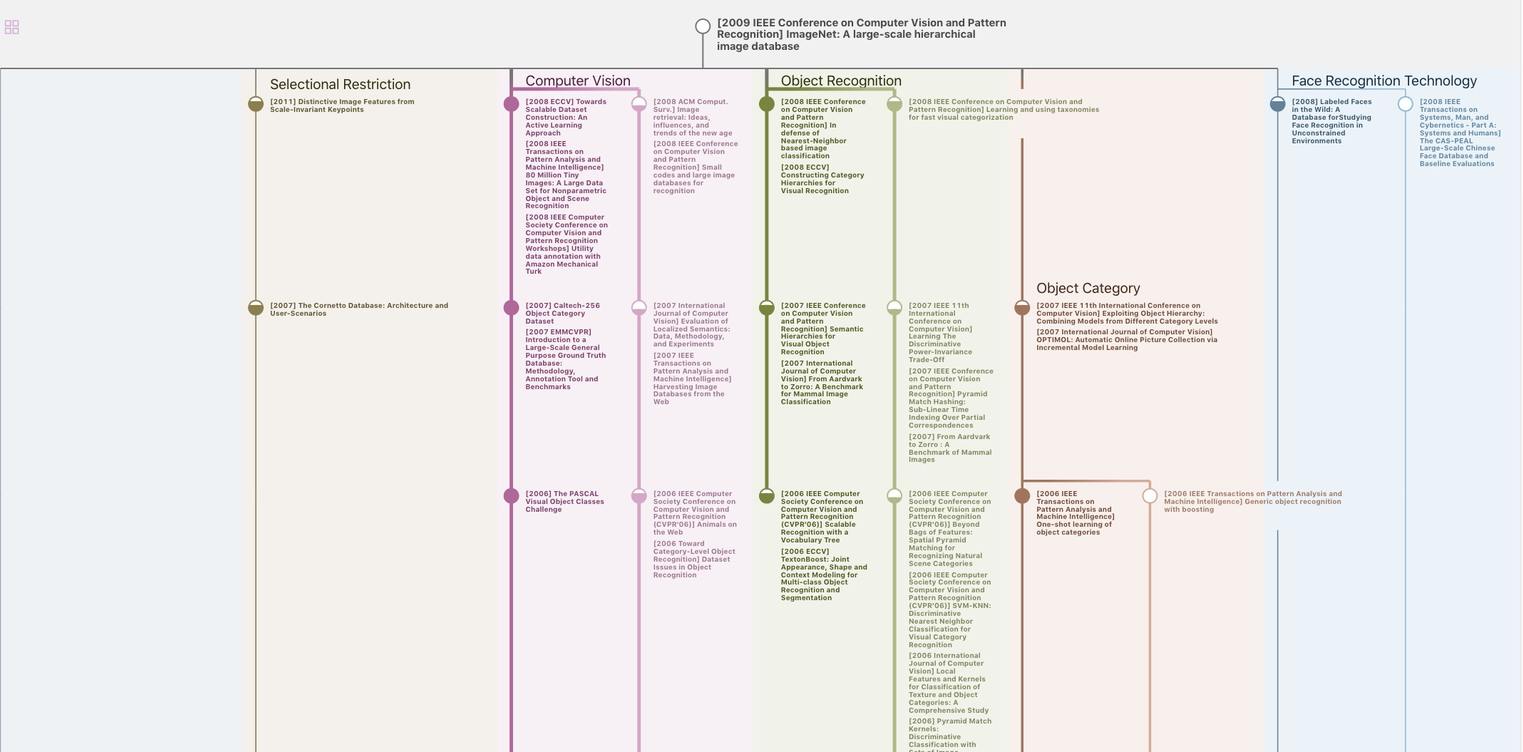
生成溯源树,研究论文发展脉络
Chat Paper
正在生成论文摘要