Classification of high mental workload and emotional statuses via machine learning feature extractions in gait
INTERNATIONAL JOURNAL OF INDUSTRIAL ERGONOMICS(2023)
摘要
Gait features could help to identify workers' mental workload and different emotional statuses. In the current study, thirty participants were recruited to walk after four emotional states were elicited and the gait data was collected using the optical motion capture system. The 111 posture and kinematic features were extracted from 2 min gait performances. The top 40 ranked features were selected for the Support Vector Machine (SVM) classifier to classify each emotional state. For individual emotional classification, the results revealed that the accuracy rate was 83.33% for neutral, 85.72% for happy, 85.71% for sad, and 89.29% for the high mental workload. For the model evaluation of feature weighting, the minimum value of the right foot in the x- and z-axis, cadence, and stride length were ranked as the top four features. The successful classification could help administrators be aware of employers’ mental and emotional statuses during work.
更多查看译文
关键词
Gait features,Support vector machine,Mental workload and emotional classification,Kinematics
AI 理解论文
溯源树
样例
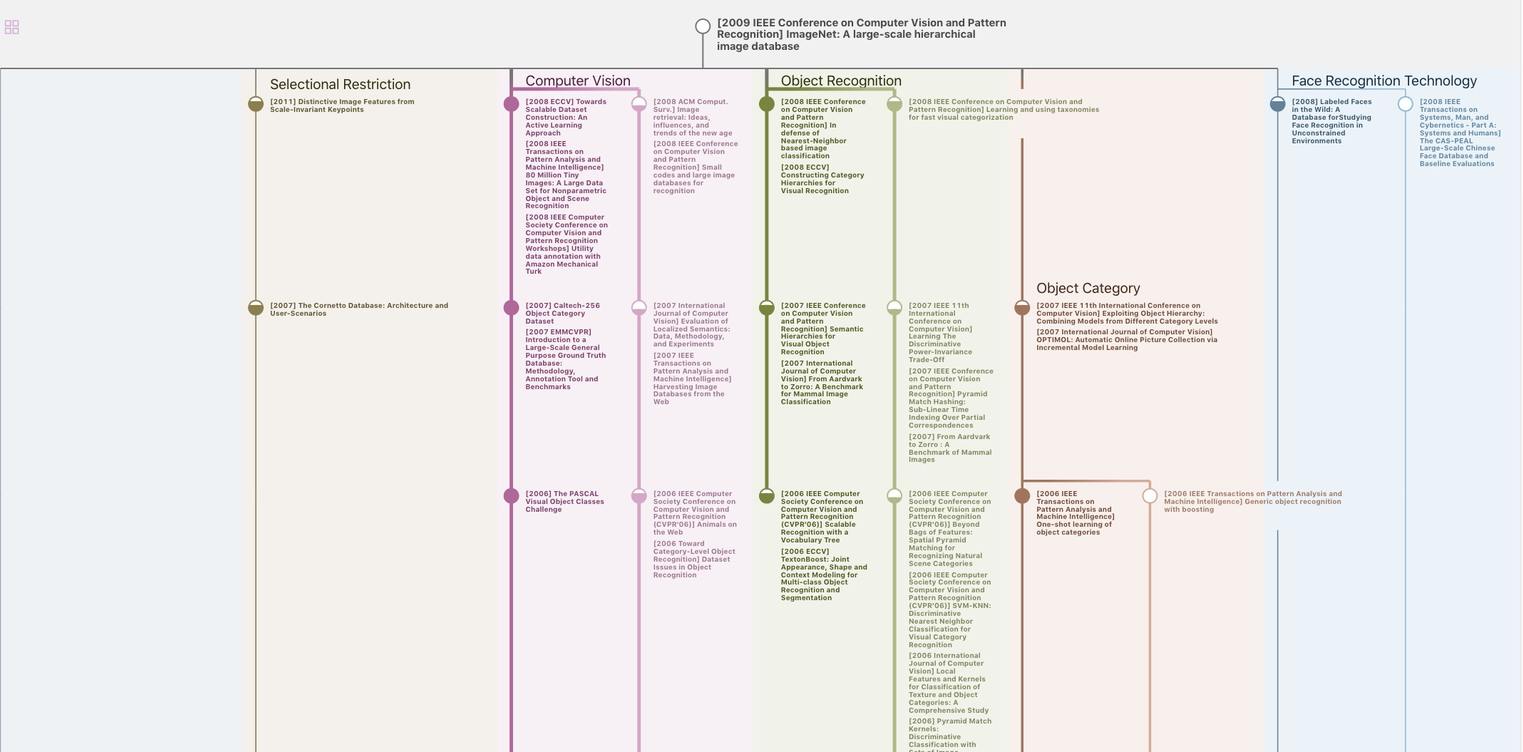
生成溯源树,研究论文发展脉络
Chat Paper
正在生成论文摘要