TAU: A neural network based telluric correction framework
ASTRONOMY & ASTROPHYSICS(2023)
摘要
Context. Telluric correction is one of the critically important outstanding issues for extreme precision radial velocities and exoplanet atmosphere observations. Thorough removal of small so-called micro tellurics across the entire wavelength range of optical spectrographs is necessary in order to reach the extreme radial velocity precision required to detect Earth-analog exoplanets orbiting in the habitable zone of solar-type stars. Likewise, proper treatment of telluric absorption will be important for exoplanetary atmosphere observations with high-resolution spectrographs on future extremely large telescopes (ELTs). Aims. In this work, we introduce the Telluric AUtoencoder (TAU). TAU is an accurate high-speed telluric correction framework built to extract the telluric spectrum with previously unobtained precision in a computationally efficient manner. Methods. TAU is built on a neural network autoencoder trained to extract a highly detailed telluric transmission spectrum from a large set of high-precision observed solar spectra. We accomplished this by reducing the data into a compressed representation, allowing us to unveil the underlying solar spectrum and simultaneously uncover the different modes of variation in the observed spectra relating to the absorption from H2O and O-2 in the atmosphere of Earth. Results. We demonstrate the approach on data from the HARPS-N spectrograph and show how the extracted components can be scaled to remove H2O and O-2 telluric contamination with improved accuracy and at a significantly lower computational expense than the current state of the art synthetic approach molecfit. We also demonstrate the capabilities of TAU to remove telluric contamination from observations of the ultra-hot Jupiter HAT-P-70b allowing for the retrieval of the atmospheric signal. We publish the extracted components and an open-source code base allowing scholars to apply TAU on their own data.
更多查看译文
关键词
neural network
AI 理解论文
溯源树
样例
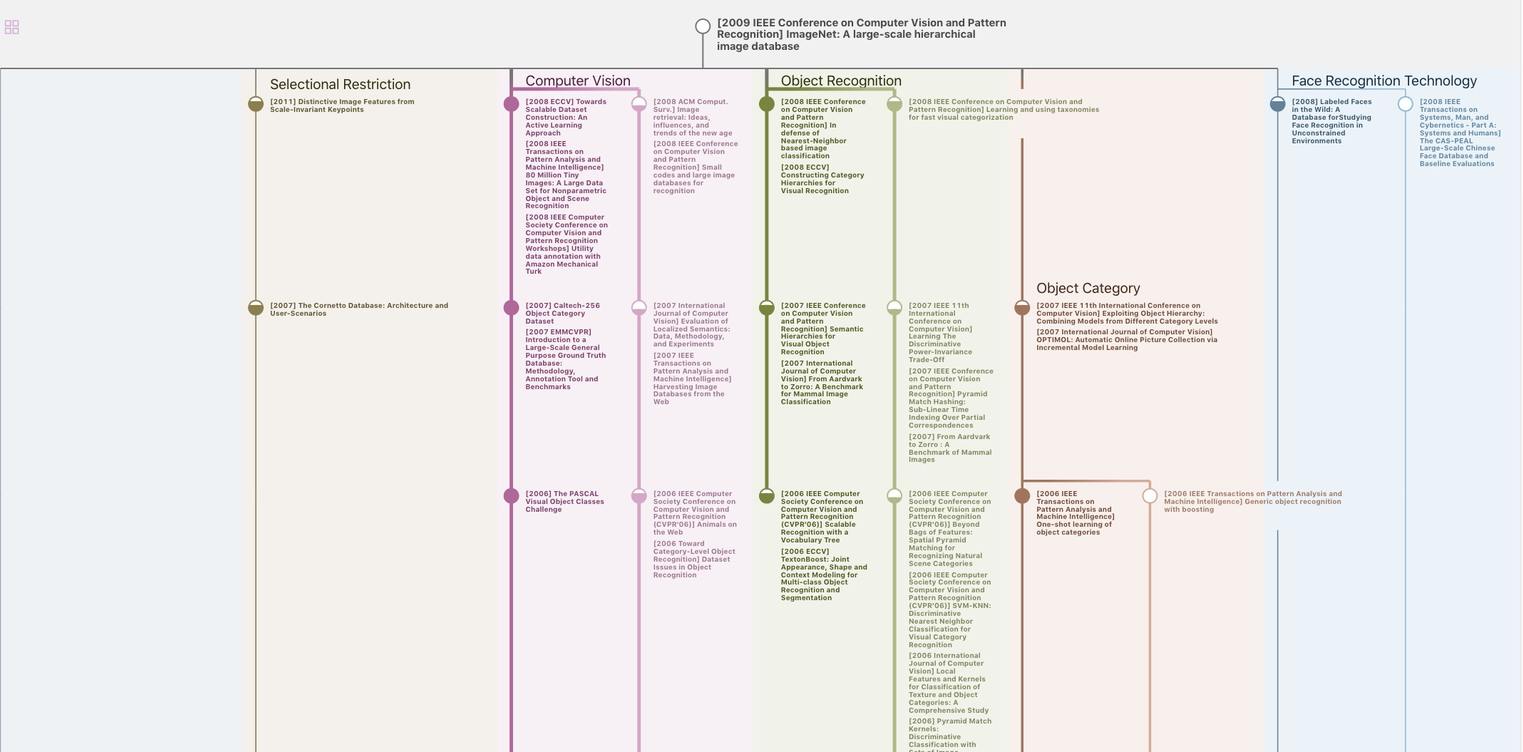
生成溯源树,研究论文发展脉络
Chat Paper
正在生成论文摘要