Long short-term memory model for predicting groundwater level in Alabama
JOURNAL OF THE AMERICAN WATER RESOURCES ASSOCIATION(2024)
摘要
Groundwater serves as a primary source of public-water and agricultural supply in many areas of Alabama, in particular during drought periods. Long-term climatic models for the southeastern United States indicate that the region will be subjected to more intense and more frequent precipitation events, with no overall change in the amount of precipitation, resulting in increased runoff and reduced aquifer recharge. Reliable prediction of groundwater levels would be beneficial to water resources decision makers and stakeholders especially for time-sensitive decisions. This paper uses a compound application of continuous wavelet transform (CWT) analysis and long short-term memory (LSTM) framework to address the major question with regards to groundwater level: "how long does it take for groundwater to respond to major precipitation events and what is the magnitude of the response?" CWT analysis is used to answer the "how long" part in this question, while the LSTM is used to answer the "what is the magnitude" part of the question. The insights from CWT analysis related to the short-term and long-term response in groundwater level were used to set the parameters of the LSTM model. The LSTM model uses daily groundwater levels, precipitation, and maximum/minimum temperatures as input data. The model was able to provide predictions within a 95% confidence interval of actual groundwater levels. The findings of this study suggest a workflow for groundwater level forecasting in the wells of Alabama given a minimum amount of easy-to-measure and widely available data.
更多查看译文
关键词
groundwater level prediction,recurrent neural network,long short-term memory,continuous wavelet transform analysis
AI 理解论文
溯源树
样例
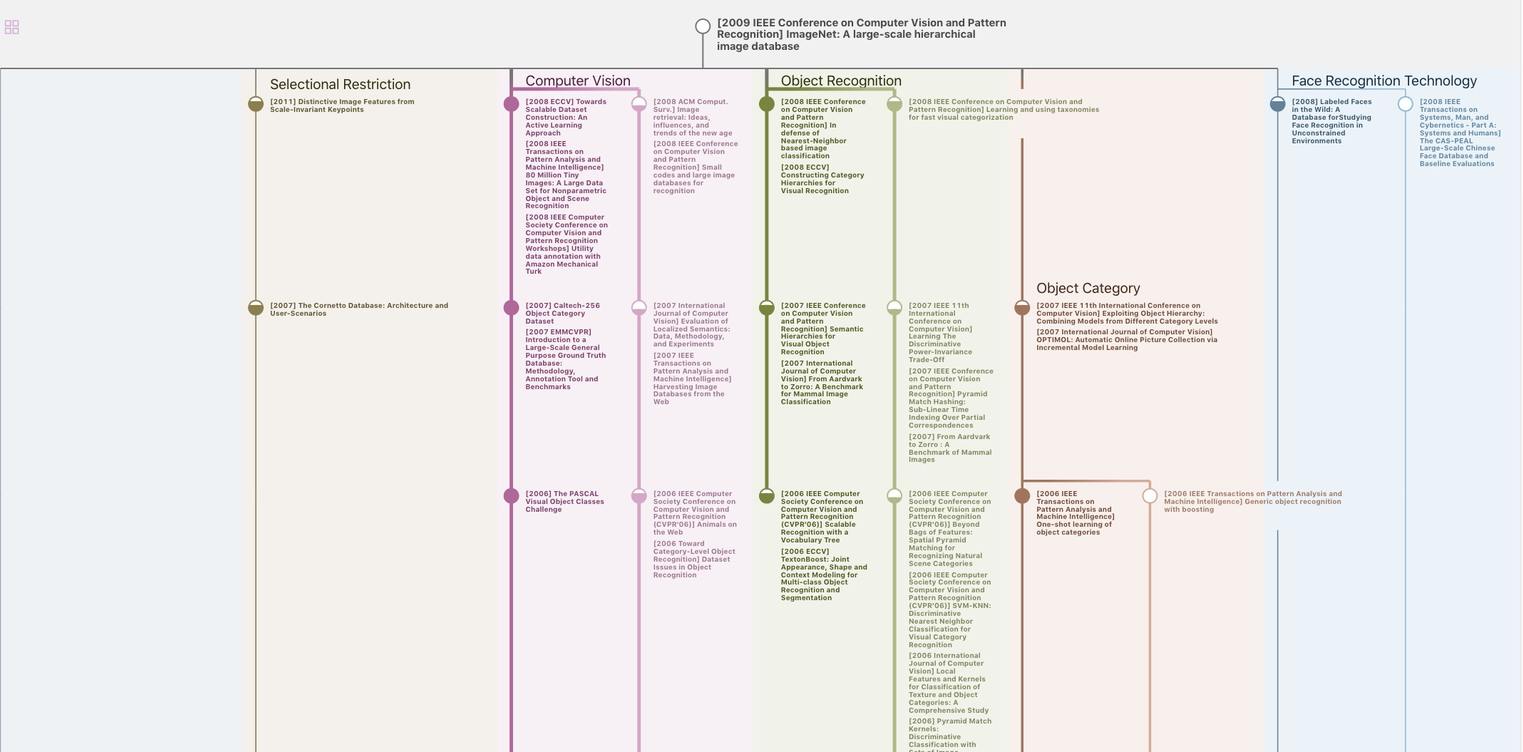
生成溯源树,研究论文发展脉络
Chat Paper
正在生成论文摘要