DeepMapScaler: a Workflow of Deep Neural Networks for the Generation of Generalised Maps
CARTOGRAPHY AND GEOGRAPHIC INFORMATION SCIENCE(2024)
摘要
The automation of map generalization has been an important research subject for decades but is not fully solved yet. Deep learning techniques are designed for various image generation tasks, so one may think that it would be possible to apply these techniques to cartography and train a holistic model for end-to-end map generalization. On the contrary, we assume that map generalization is a task too complex to be learnt with a unique model. Thus, in this article, we propose to resort to past research on map generalization and to separate map generalization into simpler sub-tasks, each of which can be more easily resolved by a deep neural network. Our main contribution is a workflow of deep models, called DeepMapScaler, which achieves a step-by-step topographic map generalization from detailed topographic data. First, we implement this workflow to generalize topographic maps containing roads, buildings, and rivers at a medium scale (1:50k) from a detailed dataset. The results of each step are quantitatively and visually evaluated. Then the generalized images are compared with the generalization performed using a holistic model for an end-to-end map generalization and a traditional semi-automatic map generalization process. The experiment shows that the workflow approach is more promising than the holistic model, as each sub-task is specialized and fine-tuned accordingly. However, the results still do not reach the quality level of the semi-automatic traditional map generalization process, as some sub-tasks are more complex to handle with neural networks.
更多查看译文
关键词
Map generalization,generative adversarial network,deep learning,workflow,cartography
AI 理解论文
溯源树
样例
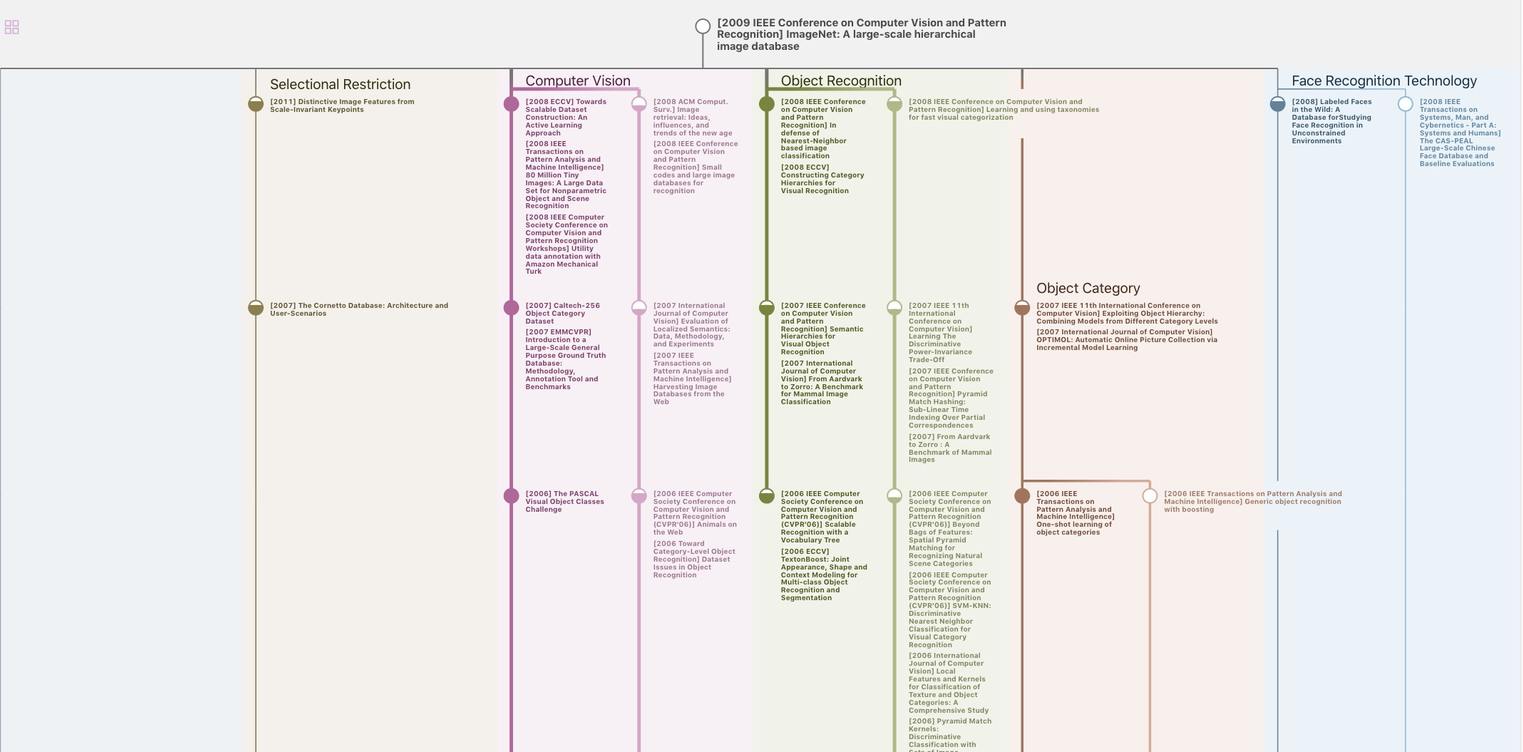
生成溯源树,研究论文发展脉络
Chat Paper
正在生成论文摘要