Deep learning, numerical, and experimental methods to reveal hydrodynamics performance and cavitation development in centrifugal pump
EXPERT SYSTEMS WITH APPLICATIONS(2024)
摘要
The hydrodynamic performance and cavitation development in centrifugal pump have a decisive impact on its energy conversion and performance. However, there are still bottlenecks when using current experimental methods and simulation algorithms in the real-time measurement and visual display of flow fields, and the high experimental and computational cost cannot be ignored. Here, we integrated computational fluid dynamics (CFD) and experimental platform with our customized framework based on a multi-attribute point cloud dataset and advanced deep learning network. This combination is made possible by our workflow to generate simulated data of flow characteristics of cavitation in the pump as the training/ test dataset, complete the deep learning algorithm process and check the consistency with the experimental results. Deep learning models the multiphase flow system of centrifugal pump and completes the mapping from the structure of pump and working conditions to the cavitation, pressure, and velocity field. The statistical analysis shows that predictions results are in agreement with the CFD method, but the calculation time is greatly reduced. Compared to the prevalent methods, the proposed deep learning framework shows superior performance in accuracy, computational cost, visual display and has the potential of generality to model the interaction between different fluids and impellers.
更多查看译文
关键词
Centrifugal pump,Hydrodynamics,Cavitation,Multiphase flow,Deep learning
AI 理解论文
溯源树
样例
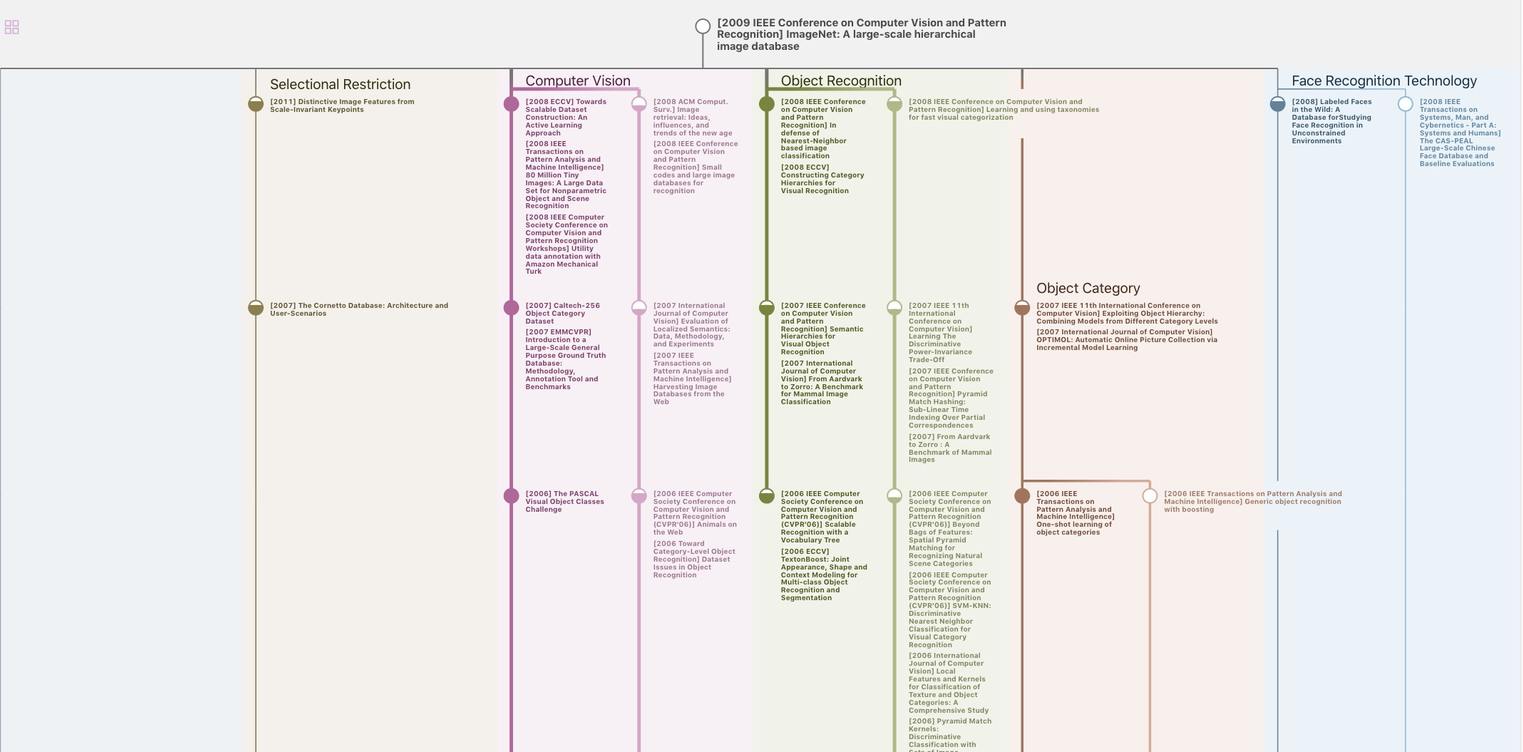
生成溯源树,研究论文发展脉络
Chat Paper
正在生成论文摘要