Multi-task-based spatiotemporal generative inference network: A novel framework for predicting the highway traffic speed
EXPERT SYSTEMS WITH APPLICATIONS(2024)
摘要
Accurately predicting the highway traffic speed can reduce traffic accidents and transit time, and it also provides valuable reference data for traffic control in advance. Three essential elements should be considered in highway traffic speed prediction: specialIntscript dynamic spatiotemporal correlation, specialIntscript prediction error propagation elimination, and specialIntscript traffic speed heterogeneity on the highway network. A multi-task-based spatiotemporal generative inference network (MT-STGIN) is proposed to address the above challenges. 1 MT-STGIN consists of three parts: an encoder based on spatiotemporal correlation extraction block (ST-Block), a decoder based on masked ST-Block and generative inference, and multi-task learning. The encoder is first used to extract the dynamic spatiotemporal correlation of the highway network. The decoder concentrates on correlating historical and -target sequences and generates the target hidden outputs rather than a dynamic step-by-step decoding way. Finally, a multi-task learning method is used to predict traffic speed on different types of road segments because of heterogeneity and shares the underlying network parameters. The evaluation experiments use the monitoring data of the highway in Yinchuan City, Ningxia Province, China. The experimental results demonstrate that the performance of the proposed prediction model is better than that of the baselines, and it can efficiently solve the problem of long-term highway speed prediction.
更多查看译文
关键词
Long-term highway traffic speed prediction,Dynamic spatiotemporal correlation,Graph neural networks,Fusion gate mechanism,Generative inference,Multi-task learning
AI 理解论文
溯源树
样例
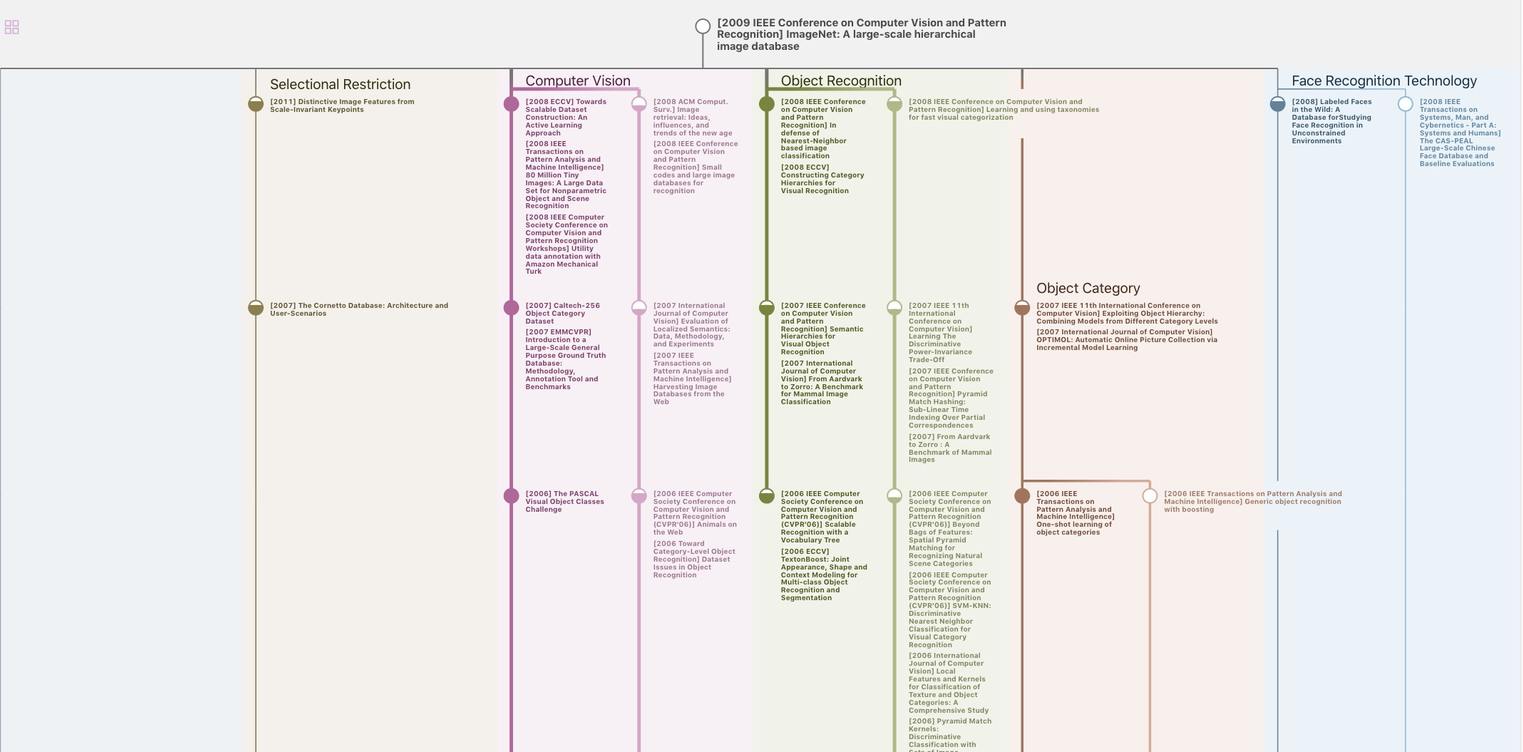
生成溯源树,研究论文发展脉络
Chat Paper
正在生成论文摘要