Lung-EffNet: Lung cancer classification using EfficientNet from CT-scan images
ENGINEERING APPLICATIONS OF ARTIFICIAL INTELLIGENCE(2023)
摘要
Lung cancer (LC) remains a leading cause of death worldwide. Early diagnosis is critical to protect innocent human lives. Computed tomography (CT) scans are one of the primary imaging modalities for lung cancer diagnosis. However, manual CT scan analysis is time-consuming and prone to errors/not accurate. Considering these shortcomings, computational methods especially machine learning and deep learning algorithms are leveraged as an alternative to accelerate the accurate detection of CT scans as cancerous, and non-cancerous. In the present article, we proposed a novel transfer learning-based predictor called, Lung-EffNet for lung cancer classification. Lung-EffNet is built based on the architecture of EfficientNet and further modified by adding top layers in the classification head of the model. Lung-EffNet is evaluated by utilizing five variants of EfficientNet i.e., B0–B4. The experiments are conducted on the benchmark dataset “IQ-OTH/NCCD” for lung cancer patients grouped as benign, malignant, or normal based on the presence or absence of lung cancer. The class imbalance issue was handled through multiple data augmentation methods to overcome the biases. The developed model Lung-EffNet attained 99.10% of accuracy and a score of 0.97 to 0.99 of ROC on the test set. We compared the efficacy of the proposed fine-tuned pre-trained EfficientNet with other pre-trained CNN architectures. The predicted outcomes demonstrate that EfficientNetB1 based Lung-EffNet outperforms other CNNs in terms of both accuracy and efficiency. Moreover, it is faster and requires fewer parameters to train than other CNN based models, making it a good choice for large-scale deployment in clinical settings and a promising tool for automated lung cancer diagnosis from CT scan images.
更多查看译文
关键词
Lung cancer,Deep learning,EfficientNetB1,Transfer learning,Medical imaging
AI 理解论文
溯源树
样例
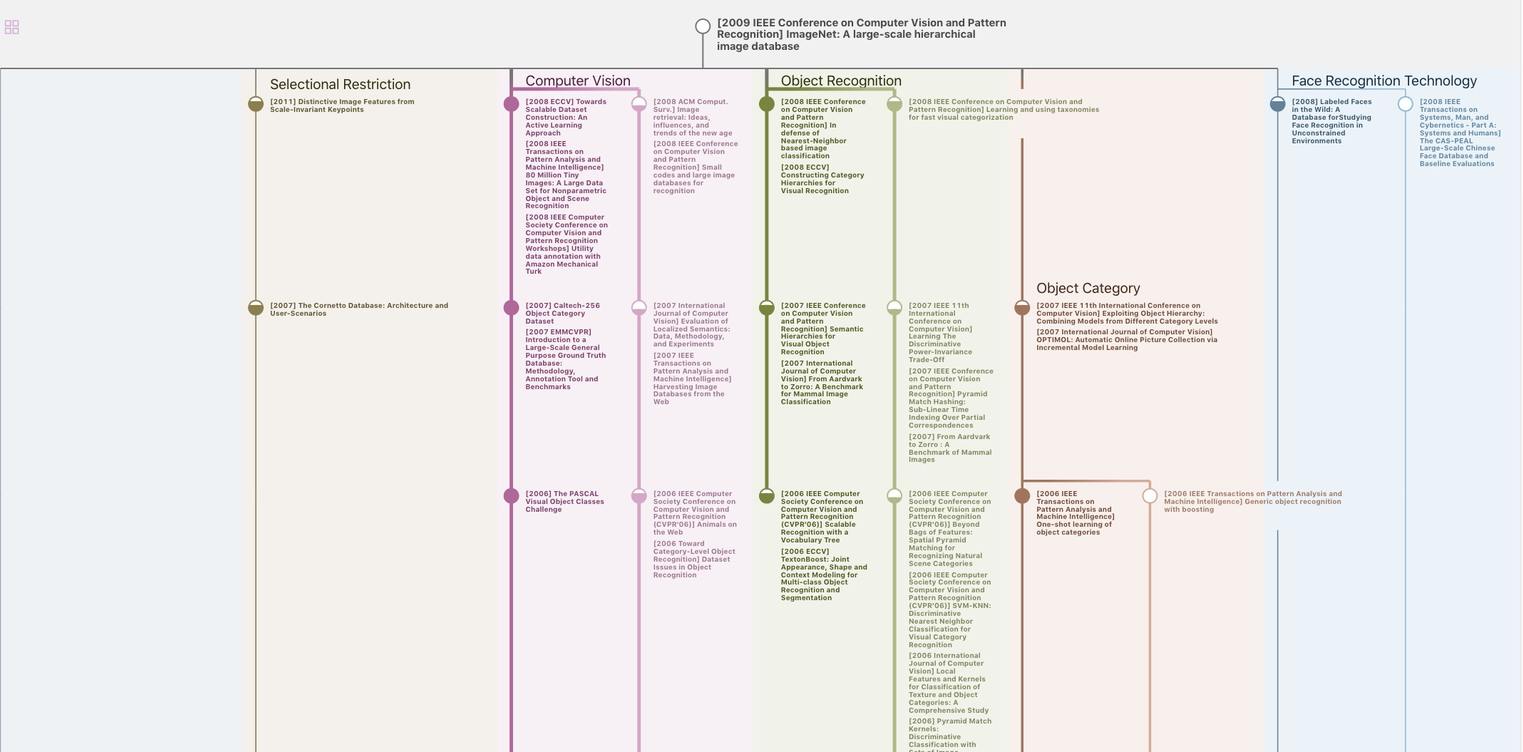
生成溯源树,研究论文发展脉络
Chat Paper
正在生成论文摘要