Distribution and Evolution of Chorus Waves Modeled by a Neural Network: the Importance of Imbalanced Regression
SPACE WEATHER-THE INTERNATIONAL JOURNAL OF RESEARCH AND APPLICATIONS(2023)
摘要
Whistler-mode chorus waves play an essential role in the acceleration and loss of energetic electrons in the Earth's inner magnetosphere, with the more intense waves producing the most dramatic effects. However, it is challenging to predict the amplitude of strong chorus waves due to the imbalanced nature of the data set, that is, there are many more non-chorus data points than strong chorus waves. Thus, traditional models usually underestimate chorus wave amplitudes significantly during active times. Using an imbalanced regressive (IR) method, we develop a neural network model of lower-band (LB) chorus waves using 7-year observations from the EMFISIS instrument onboard Van Allen Probes. The feature selection process suggests that the auroral electrojet index alone captures most of the variations of chorus waves. The large amplitude of strong chorus waves can be predicted for the first time. Furthermore, our model shows that the equatorial LB chorus's spatiotemporal evolution is similar to the drift path of substorm-injected electrons. We also show that the chorus waves have a peak amplitude at the equator in the source MLT near midnight, but toward noon, there is a local minimum in amplitude at the equator with two off-equator amplitude peaks in both hemispheres, likely caused by the bifurcated drift paths of substorm injections on the dayside. The IR-based chorus model will improve radiation belt prediction by providing chorus wave distributions, especially storm-time strong chorus. Since data imbalance is ubiquitous and inherent in space physics and other physical systems, imbalanced regressive methods deserve more attention in space physics. Whistler-mode chorus waves are essential in accelerating radiation belt electrons. However, predicting the amplitude of strong chorus waves is difficult because of their imbalanced nature. In other words, there are many more observations of no-chorus waves than strong chorus waves. A consequence is that these no-chorus wave data dominate traditional models, so these models usually predict values that are too small for strong waves. Using an imbalanced regressive method, we developed a machine learning (ML) model of lower-band chorus wave amplitude. For the first time, the ML-chorus model can predict the amplitude of strong chorus waves. The ML-chorus model shows the evolution of the chorus wave at the equator, similar to the drift path of injected electrons, which brings electron anisotropy that generates chorus waves. The ML-chorus model shows that the chorus waves are stronger at the equator near midnight, the source region of plasma injection. Away from midnight, the chorus waves have an equatorial minimum instead. Our chorus model will improve the forecast of the radiation belt environment by providing chorus wave distributions, especially large-amplitude strong chorus during geomagnetic storms. Because data imbalance is commonly seen in space physics and other physical systems, imbalanced regressive methods require more attention. A neural network model of lower-band chorus wave amplitude is developed using imbalanced regressionFor the first time, a chorus model can predict the large amplitude of strong chorus wavesChorus' evolution is consistent with electron injection drift paths, peak equatorial amplitude near midnight, and off-equator peaks at noon
更多查看译文
关键词
chorus waves,imbalanced regression,neural network,machine learning,radiation belt,Van Allen Probe
AI 理解论文
溯源树
样例
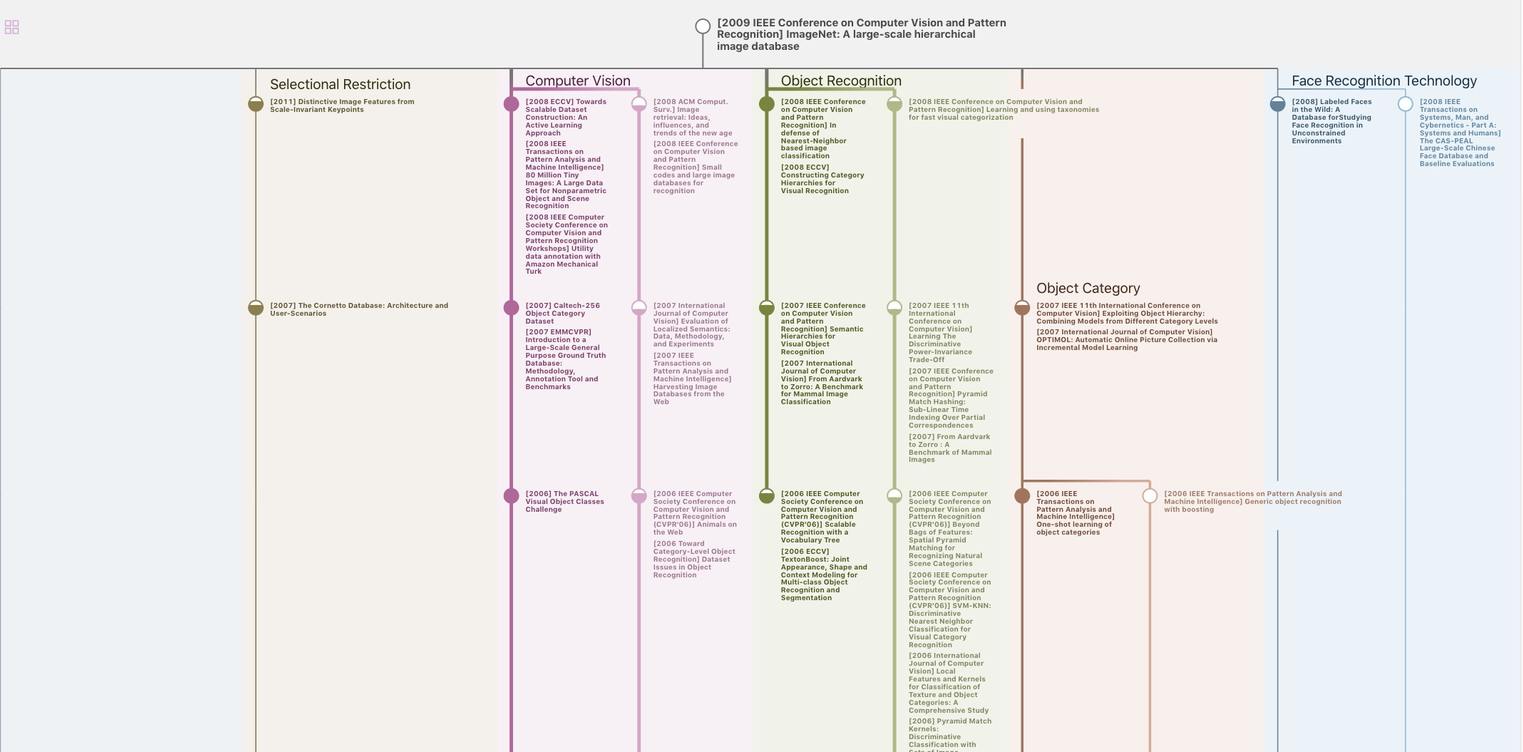
生成溯源树,研究论文发展脉络
Chat Paper
正在生成论文摘要