Comparison of genomic prediction models for general combining ability in early stages of hybrid breeding programs
CROP SCIENCE(2023)
摘要
This study evaluates the impact of genomic prediction models on selecting inbred lines as parents in hybrid breeding programs. New parents in a hybrid breeding program are typically selected from early-stage yield trials based on general combining ability (GCA) from testcrosses. Genomic studies have largely focused on predicting hybrid performance in the late stages of the breeding pipeline and largely ignored the selection of inbred lines as parents of the subsequent breeding cycles. Here, we used stochastic simulations of a maize (Zea mays L.) hybrid breeding program for 20 years to evaluate the performance of genomic prediction models for selecting parents based on their predicted GCA. Five genomic prediction models were evaluated in terms of achieved genetic gain and heterosis under two different single nucleotide polymorphism (SNP) marker densities and the true quantitative trait loci genotypes. The results show that using high-density SNP markers generated more genetic gain and heterosis than the low-density SNP markers. The relative performance of genomic prediction models differed across marker scenarios. For genetic gain, we observed more differences between the models at low than high marker density. For heterosis, we observed the opposite, more differences between the models at high than low marker density. Overall, models that fitted the average or additive effects specific to each heterotic pool and dominance effects provide a better fit and hence higher genetic gain in hybrid breeding programs. Genomic prediction models for GCA enable early selection of inbreds as parents of subsequent breeding cyclesHigh-marker density is important to maximize prediction accuracy and long-term hybrid genetic gain and heterosisModeling specific average or additive effects and dominance effects present advantages over other models
更多查看译文
关键词
hybrid breeding programs,genomic prediction models,general combining ability,prediction models
AI 理解论文
溯源树
样例
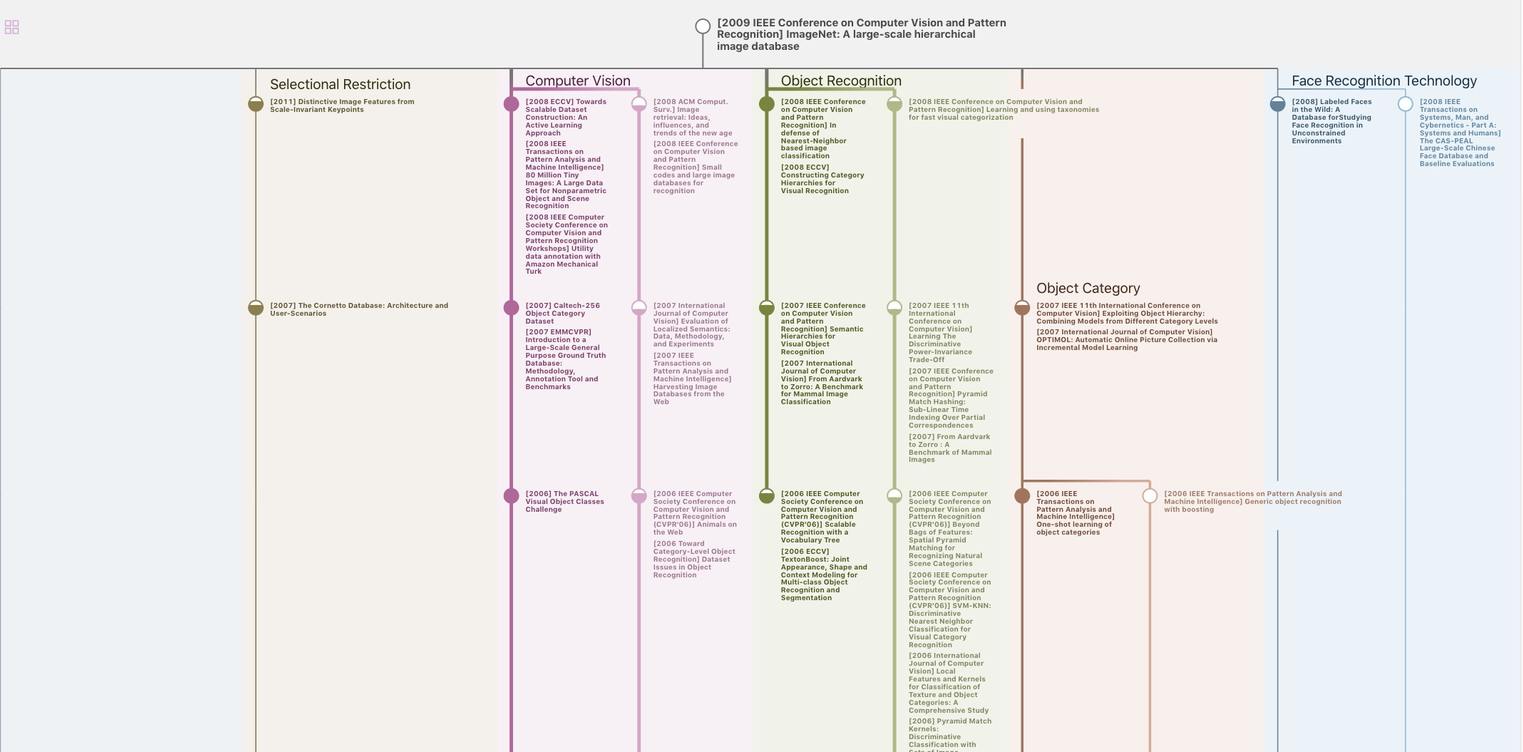
生成溯源树,研究论文发展脉络
Chat Paper
正在生成论文摘要