Overcomplete graph convolutional denoising autoencoder for noisy skeleton action recognition
IET IMAGE PROCESSING(2024)
摘要
Current skeleton-based action recognition methods usually assume the input skeleton is complete and noise-free. However, it is inevitable that the captured skeletons are incomplete due to occlusions or noisy due to changes in the environment. When dealing with these data, even State Of The Art (SOTA) recognition backbones experience significant degradation in recognition accuracy. Though a few methods have been proposed to address this issue, they still lack flexibility, efficiency and interpretability. In this work, an overcomplete Graph Convolutional Denoising Autoencoder (GCDAE) is proposed which can act as a flexible preprocessing module for pretrained recognition backbones and improve their robustness. Taking advantages of the overcomplete and fully graph convolutional structure, GCDAE is able to rectify noisy joints while keeping information of unspoiled details efficiently. On two large scale skeleton datasets NTU RGB+D 60 and 120, the introducing of GCDAE brings significant robustness improvements to SOTA backbones towards different types of noises. To deal with incomplete and noisy skeletons in real-world action recognition, an overcomplete Graph Convolutional Denoising Autoencoder (GCDAE) is proposed. The overcomplete and fully graph convolutional structure allows it to rectify noisy joints while preserving unspoiled details, making it possible to combine with any pretrained recognition backbones and improve their robustness in an efficient way.image
更多查看译文
关键词
computer vision,convolutional neural nets,graph theory,signal denoising
AI 理解论文
溯源树
样例
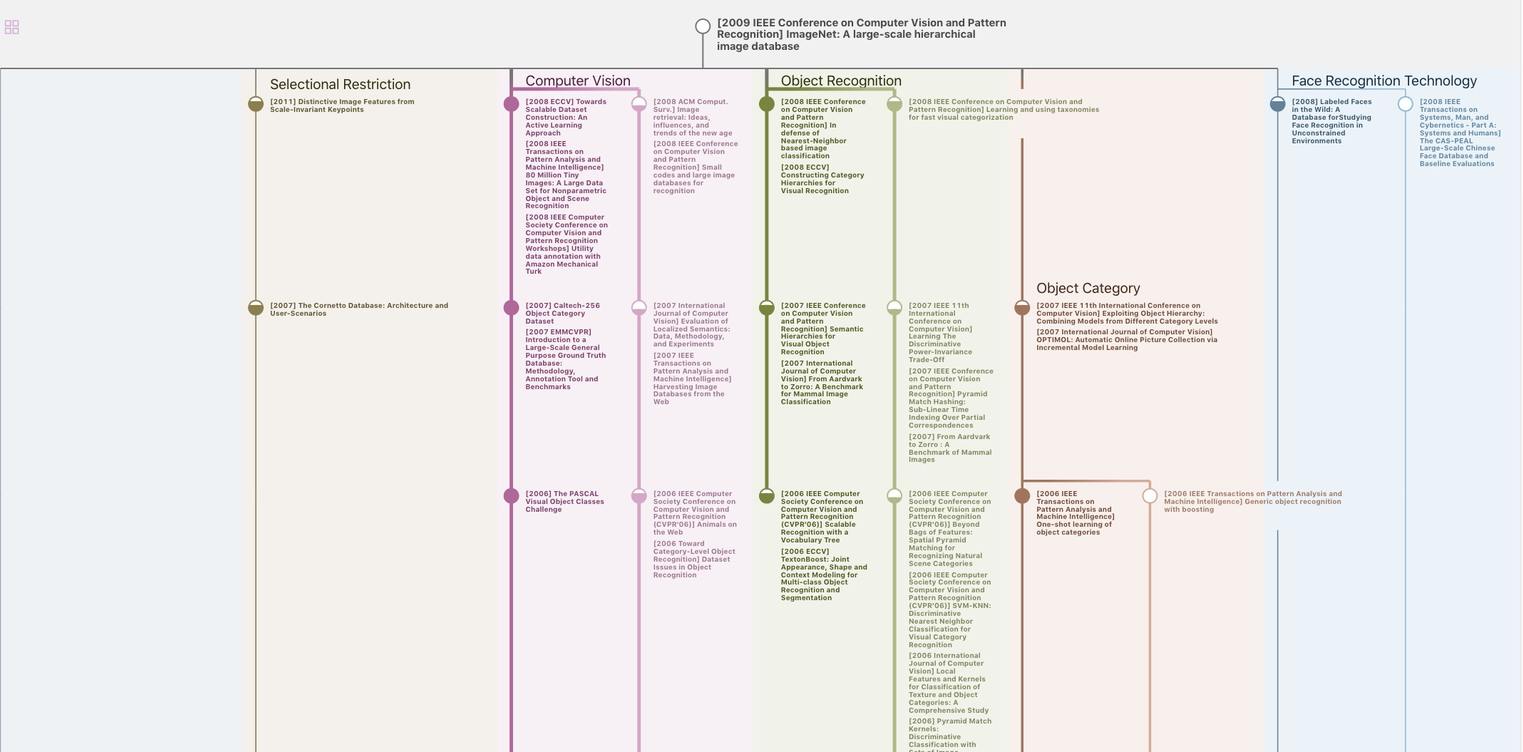
生成溯源树,研究论文发展脉络
Chat Paper
正在生成论文摘要