Attribute-wise reasoning reinforcement learning for pedestrian attribute retrieval
INTERNATIONAL JOURNAL OF MULTIMEDIA INFORMATION RETRIEVAL(2023)
摘要
Pedestrian attribute retrieval (PAR) aims at retrieving soft-biometric attributes of pedestrian images from video surveillance. Despite advancements, PAR grapples with challenges, notably the concern of attribute imbalanced distribution. Within this context, we highlight a critical observation: this challenge encompasses both inter-attribute and overlooked intra-attribute imbalanced data distribution. To address the overlooked intra-attribute imbalance problem, we introduce an attribute-wise reasoning reinforcement learning framework (AwRL). AwRL formulates PAR as a Markov decision process (MDP), orchestrating attribute retrieval individually within reinforcement learning episodes. By traversing the entire PAR dataset, each attribute retrieval is calibrated with distinct reward scales, thereby ameliorating the intra-attribute imbalance. Additionally, we develop a novel supervised reinforcement loss function (SR-Loss) to enhance the robustness of the retrieval model. SR-Loss mitigates reinforcement learning’s inherent training instability in the trial-and-error interactions with the environment. The experimental results on three benchmark datasets of PETA, RAP and PA100K demonstrate the effectiveness of our approach, underscoring its capacity to surmount the intra-attribute imbalanced problem.
更多查看译文
关键词
Pedestrian attribute retrieval, Reinforcement learning, Intelligent surveillance
AI 理解论文
溯源树
样例
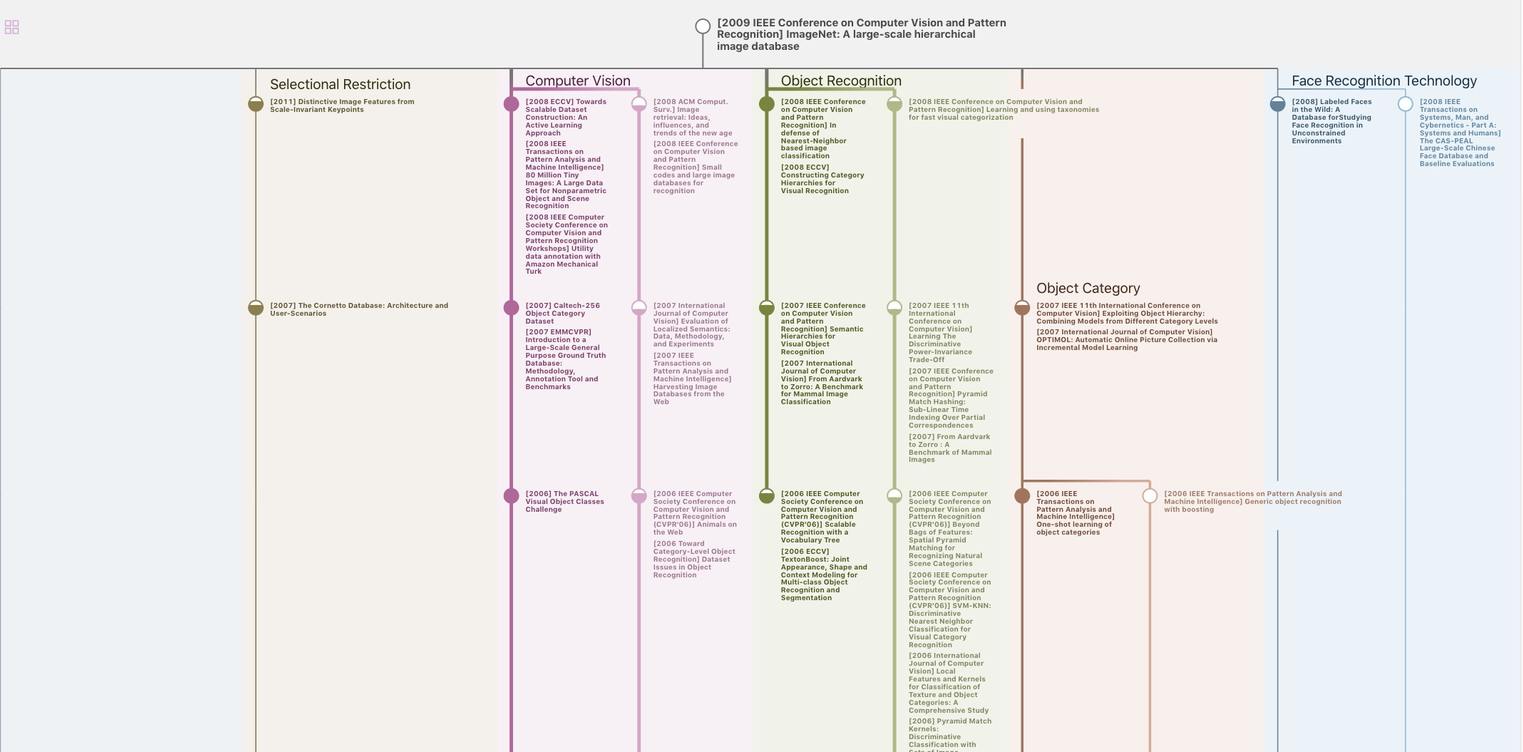
生成溯源树,研究论文发展脉络
Chat Paper
正在生成论文摘要