Review of the current status and the potential of machine learning tools in boiling heat transfer
NUMERICAL HEAT TRANSFER PART B-FUNDAMENTALS(2023)
摘要
Boiling is a liquid-to-vapor phase change process that involves complex and high dimensional processes spanning multiple length- and time-scales. Most correlations apply to a narrow range of conditions and the development of mechanistic models has been limited. Machine learning (ML) provides tools to uncover fundamental principles governing such challenging problems. It uses a variety of techniques to analyze large datasets and identify patterns and relationships that are otherwise challenging to establish using the traditional methods. Attempts have already been made to leverage the power of ML to provide new insights and make more accurate predictions in boiling heat transfer. We here report a comprehensive review of such studies, put in perspective their findings with the existing knowledgebase obtained using traditional approaches, and identify the key advantages and challenges of ML in this domain. The review suggests that it is important to pay attention to data collection, feature selection and extraction, choice of algorithm, and performance metrics to improve the accuracy, reliability, and robustness of ML models. While the traditional analytical and empirical models are more interpretable and easier to visualize, ML models are noted to enable more accurate predictions over a wider range of operating conditions. The issues of interpretability can be addressed by reducing the complexity of the data via dimensionality reduction and clustering techniques. Moreover, physics-inspired techniques can be used to align predictions with physical principles and improve generalizability. The ability of ML to swiftly process alternative forms of data such as optical images, thermal maps, and acoustics in real-time opens new frontiers for investigations. Finally, we close the discussion by emphasizing the need for high-quality data collection and standardization for increasing the impact of ML in phase change heat transfer.
更多查看译文
关键词
heat transfer,machine learning tools,machine learning
AI 理解论文
溯源树
样例
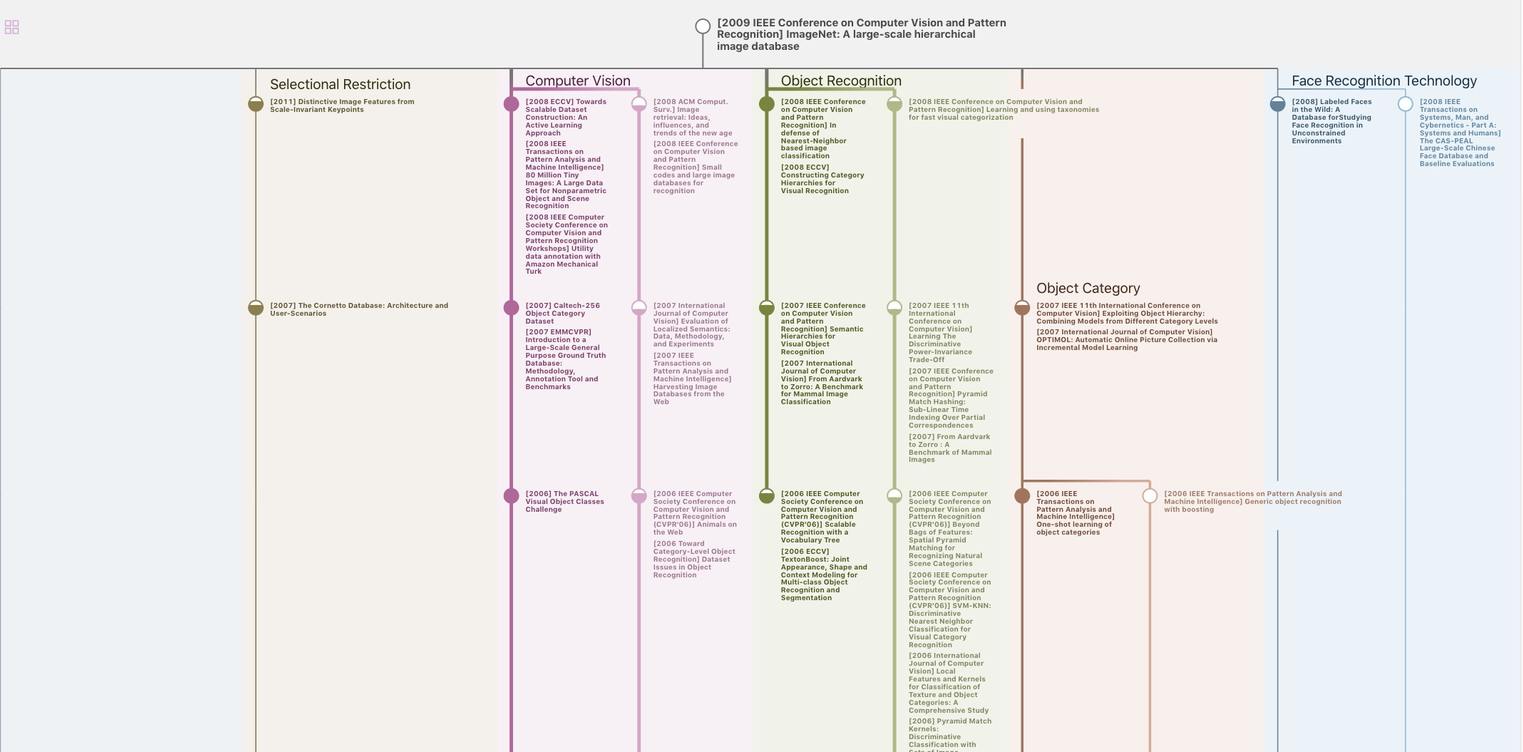
生成溯源树,研究论文发展脉络
Chat Paper
正在生成论文摘要