Penalized estimating equations for generalized linear models with multiple imputation
ANNALS OF APPLIED STATISTICS(2023)
摘要
Missing values among variables present a challenge in variable selection in the generalized linear model. Common strategies that delete observations with missing information may cause serious information loss. Multiple imputation has been widely used in recent years because it provides unbiased statistical results given a correctly specified imputation model and considers the uncertainty of the missing data. However, variable selection methods in the generalized linear model with multiply-imputed data have not yet been studied widely. In this study, we introduce penalized estimating equations for generalized linear models with multiple imputation (PEE-MI), which incorporates the correlation of multiple imputed observations into the objective function. The theoretical performance of the proposed PEE-MI depends on the penalized function adopted. We use the adaptive least absolute shrinkage and selection operator (adaptive LASSO) as an illustrating example. Simulations show that PEE-MI outperforms the alternatives. The proposed method is shown to select variables with clinical relevance when applied to a database of laboratory-diagnosed A/H7N9 patients in the Zhejiang province, China.
更多查看译文
关键词
generalized linear models,multiple imputation,linear models
AI 理解论文
溯源树
样例
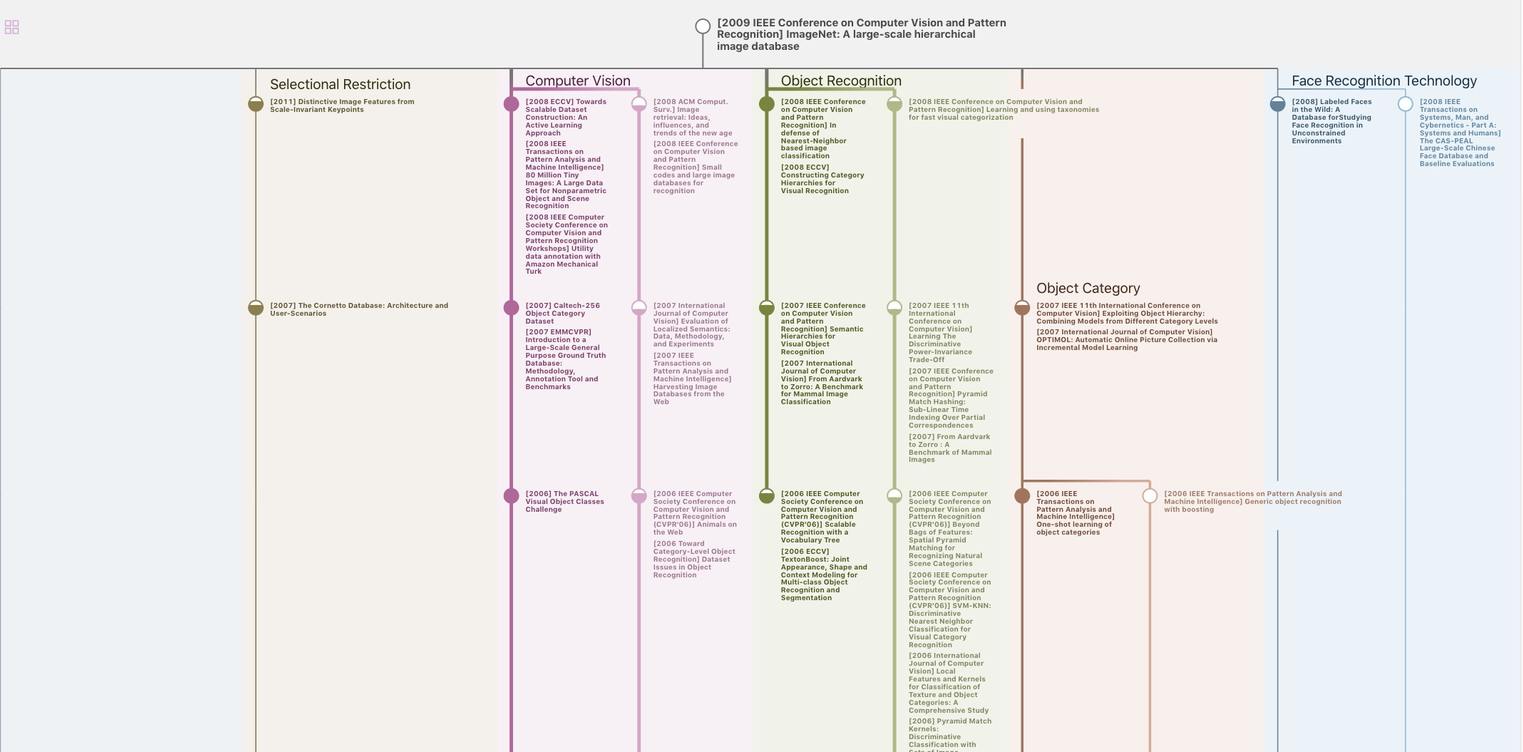
生成溯源树,研究论文发展脉络
Chat Paper
正在生成论文摘要