Orthogonal Autoencoders for Long-Term State-of-Charge Forecasting of Li-ion Battery Cells
2023 IEEE TRANSPORTATION ELECTRIFICATION CONFERENCE & EXPO, ITEC(2023)
摘要
This paper proposes an Orthogonal Autoencoded Long-Short-Term Memory (OALSTM) network for long-term the State-Of-Charge (SOC) forecasting in Lithium-ion (Li-ion) battery cells. By leveraging the use of LSTMs in capturing temporal trends and orthogonal Autoencoder for extracting non-trivial robust latent features, OALSTM can achieve precise and accurate long-term SOC estimations near the end-of-life. One key contribution is learning orthogonal temporal encodings that generalize for long-term forecasting because it reduces the likelihood of false multicollinearity. Our results show that OALSTM outperforms other benchmark models for long-term SOC estimation of Li-ion battery cells under varying charging and discharging conditions.
更多查看译文
关键词
Li-ion Battery,State-of-Charge,Autoencoders,LSTMs,long-term forecasting
AI 理解论文
溯源树
样例
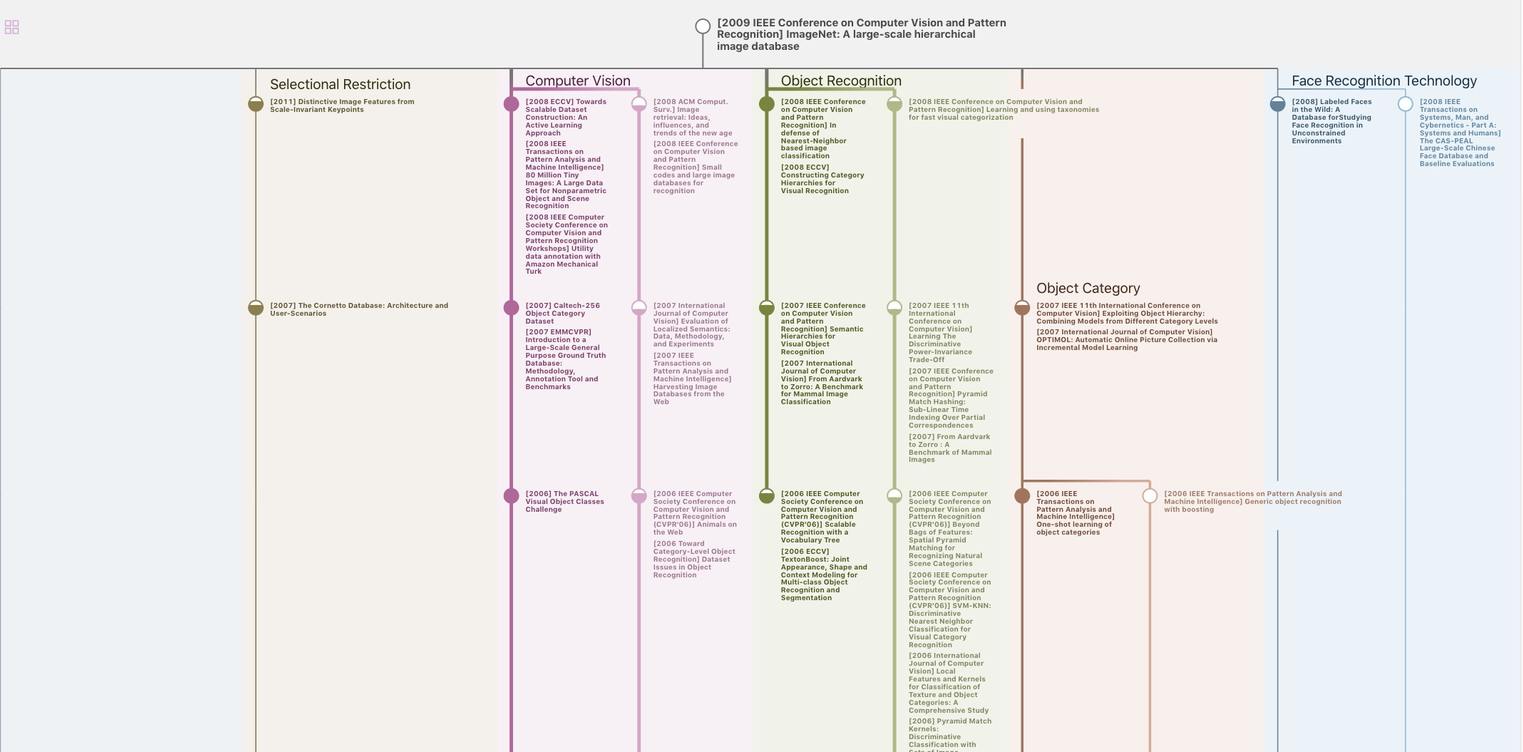
生成溯源树,研究论文发展脉络
Chat Paper
正在生成论文摘要