Reduced Operational Inhomogeneities in a Reconfigurable Parallelly-Connected Battery Pack Using DQN Reinforcement Learning Technique
2023 IEEE TRANSPORTATION ELECTRIFICATION CONFERENCE & EXPO, ITEC(2023)
摘要
Battery cells that are placed in parallel in order to increase capacity are commonly considered single-series cells. In reality, there exist unavoidable variations between cells due to manufacturing processes as well as operational conditions that create current and State of Charge (SOC) inhomogeneities. If these inhomogeneities are not taken into consideration, accelerated degradation may occur causing early decommissioning of battery packs. Literature review reveals that reconfigurable battery packs are capable of dealing with these inhomogeneities, however, that a lack of demonstrated intelligent control methods exists. Thus in this work, a novel reconfigurable battery pack topology for reducing SOC and current inhomogeneities in a parallelly connected battery pack using a Reinforcement Learning (RL) Deep Q-Network (DQN) is presented. Results show that the RL-DQN based switch controller can reduce both current and SOC imbalances over time between parallel battery cells, especially in lower degradation variation battery packs and under lower operational current rates.
更多查看译文
关键词
Machine Learning,Reconfigurable Battery Pack,Battery Management Systems,Reinforcement Learning,Battery Inhomogeneities
AI 理解论文
溯源树
样例
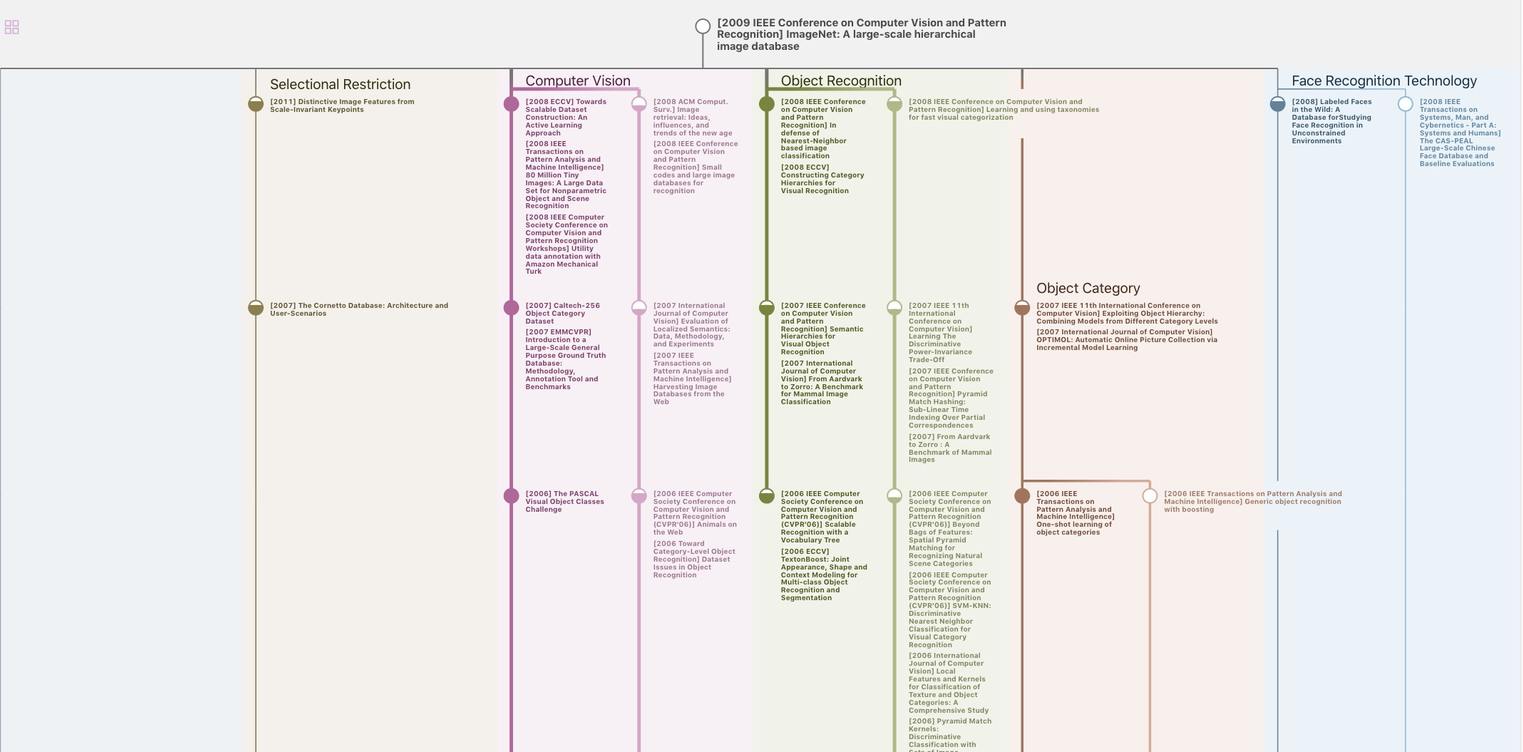
生成溯源树,研究论文发展脉络
Chat Paper
正在生成论文摘要