Long Short-Term Memory (LSTM) Based Model for Flood Forecasting in Xiangjiang River
KSCE JOURNAL OF CIVIL ENGINEERING(2023)
摘要
Due to rapid development, the occurrence of flood has become more and more frequent. However, due to the complex nature and limited knowledge, the conventional hydrological model for flood forecasting purposes faces drawbacks in terms of technical difficulties. Hence, it is essential to have a model which can provide a considerably high level of accuracy for flood forecasting. This study selected the area between the Xiangtan station and Changsha station, located in Xiangjiang River as the study area. Recurrent neural network (RNN), gated recurrent unit (GRU) and long short-term memory (LSTM) were applied for model development purposes. The models were used to forecast the flood disasters occurring in 2017, 2019, 2020 and 2022 for 1h, 6h and 12h in advance. A series of statistical analyses have been conducted to evaluate the model performance. The LSTM model outperformed than the RNN and GRU models. In terms of the overall analysis, the LSTM model achieved relatively good accuracy with a NSE of above 0.98, and RMSE of lower than 0.2 m. In addition, the model with a 6h in advance is the most suitable model to predict the peak flood arrival time with an absolute error of less than 3 hours.
更多查看译文
关键词
Flood forecasting,Gated recurrent unit,Long short-term memory,Neural network,Recurrent neural network,Water level prediction
AI 理解论文
溯源树
样例
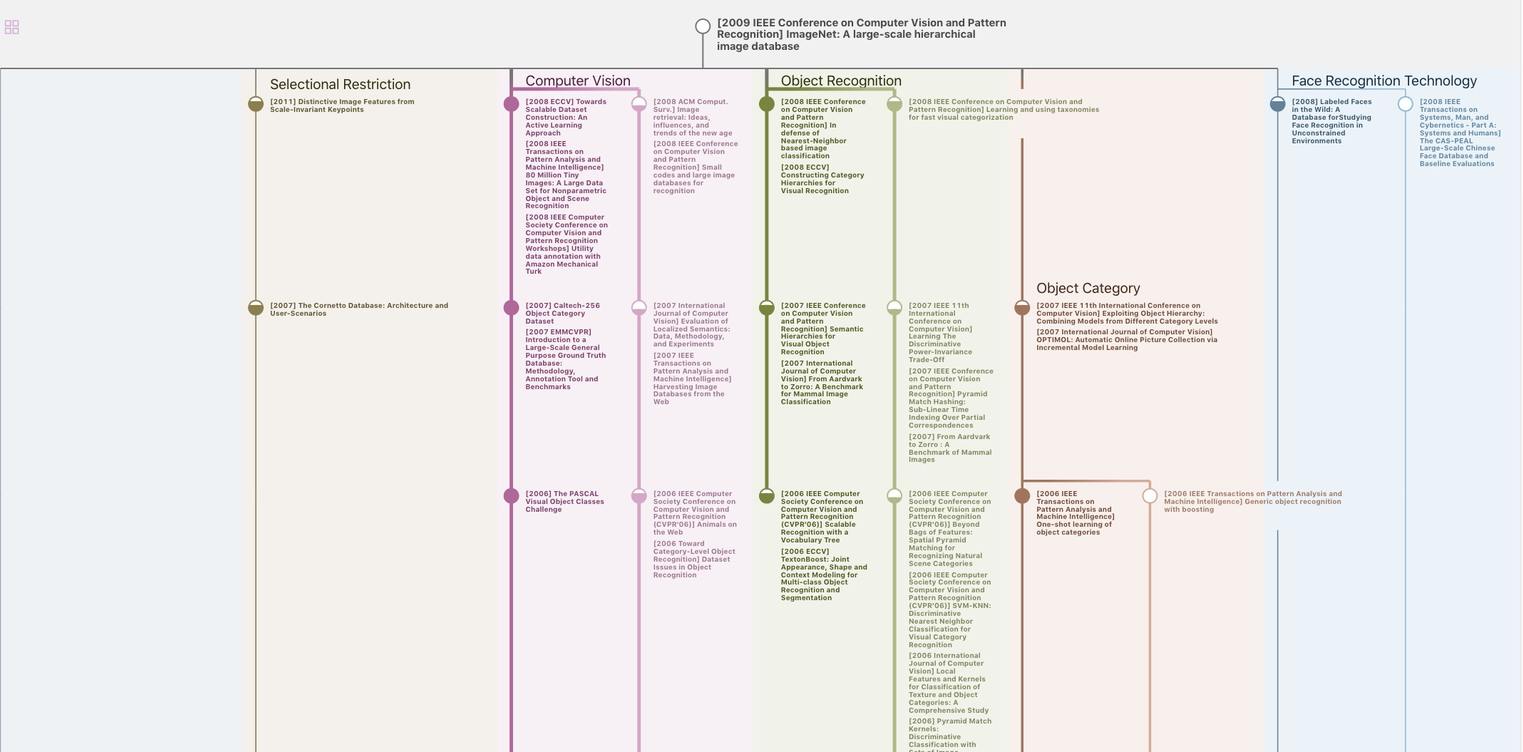
生成溯源树,研究论文发展脉络
Chat Paper
正在生成论文摘要