Assessing the Impact of Differential Privacy on Population Uniques in Geographically Aggregated Data: The Case of the 2020 U.S. Census
POPULATION RESEARCH AND POLICY REVIEW(2023)
摘要
Geographically aggregated demographic, social, and economic data are valuable for research and practical applications, but their use and sharing often compromise individual privacy. The U.S. Census Bureau has responded to this issue by introducing a new privacy protection method, the TopDown Algorithm (TDA), in the 2020 Census. The TDA is based on a privacy definition known as differential privacy and is primarily designed to reduce the risk of reconstruction-abetted disclosure, a type of privacy violation where individual identities can be revealed by reconstructing confidential census responses and linking them to publicly available data. However, there still lacks a systematic exploration of the impact of the TDA on direct disclosure, another common type of privacy violation where individuals can be directly distinguished from public census tables to reveal their identities. To address this gap, this paper examines the effectiveness of the TDA in protecting against direct disclosure by focusing on how information from public census tables can be used to distinguish population uniques, the individuals that can be uniquely distinguished from census tables. Our study reveals that while the TDA provides a reasonable level of differential privacy, it does not necessarily prevent the direct identification of population uniques using public census tables. Our finding is crucial for policymakers to consider when making informed decisions regarding parameter selection for the TDA during its implementation.
更多查看译文
关键词
Census, Disclosure avoidance, Differential privacy, TopDown algorithm
AI 理解论文
溯源树
样例
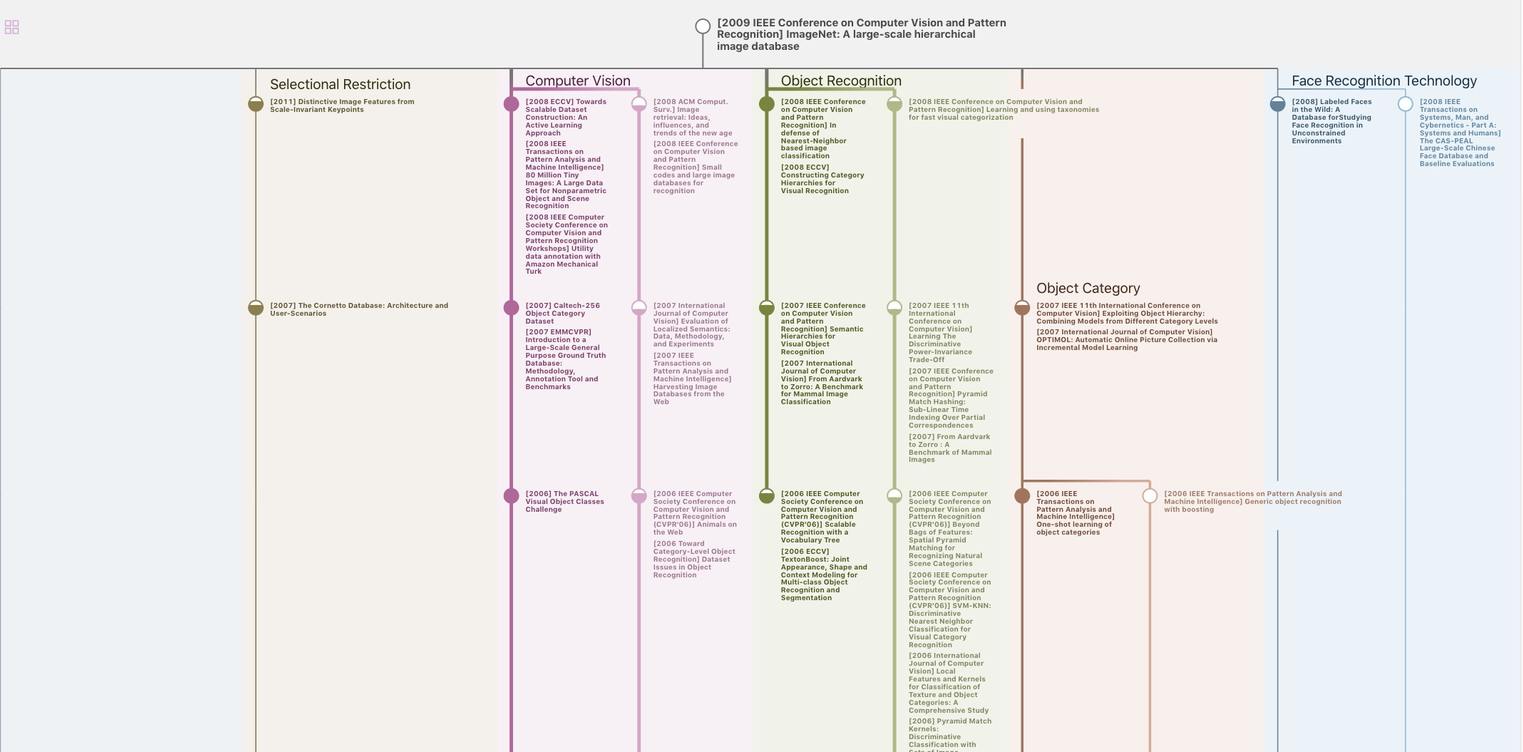
生成溯源树,研究论文发展脉络
Chat Paper
正在生成论文摘要