Downstream Task-agnostic Transferable Attacks on Language-Image Pre-training Models.
2023 IEEE INTERNATIONAL CONFERENCE ON MULTIMEDIA AND EXPO, ICME(2023)
摘要
Vision-language pre-trained models (e.g., CLIP) trained on large-scale datasets via self-supervised learning, are drawing increasing research attention since they can achieve superior performances on multi-modal downstream tasks. Nevertheless, we find that the adversarial perturbations crafted on vision-language pre-trained models can be used to attack different corresponding downstream task models. Specifically, to investigate such adversarial transferability, we introduce a task-agnostic method named Global and Local Augmentation (GLA) attack to generate highly transferable adversarial examples on CLIP, to attack black-box downstream task models. GLA adopts random crop and resize at both global and local patch levels, to create more diversity and make adversarial noises robust. Then GLA generates the adversarial perturbations by minimizing the cosine similarity between intermediate features from augmented adversarial and benign examples. Extensive experiments on three CLIP image encoders with different backbones and three different downstream tasks demonstrate the superiority of our method compared with other strong baselines. The code is available at https://github.com/yqlvcoding/GLAattack.
更多查看译文
关键词
Transfer-based Adversarial Attack,Task-agnostic,Visual-Language Pre-training model
AI 理解论文
溯源树
样例
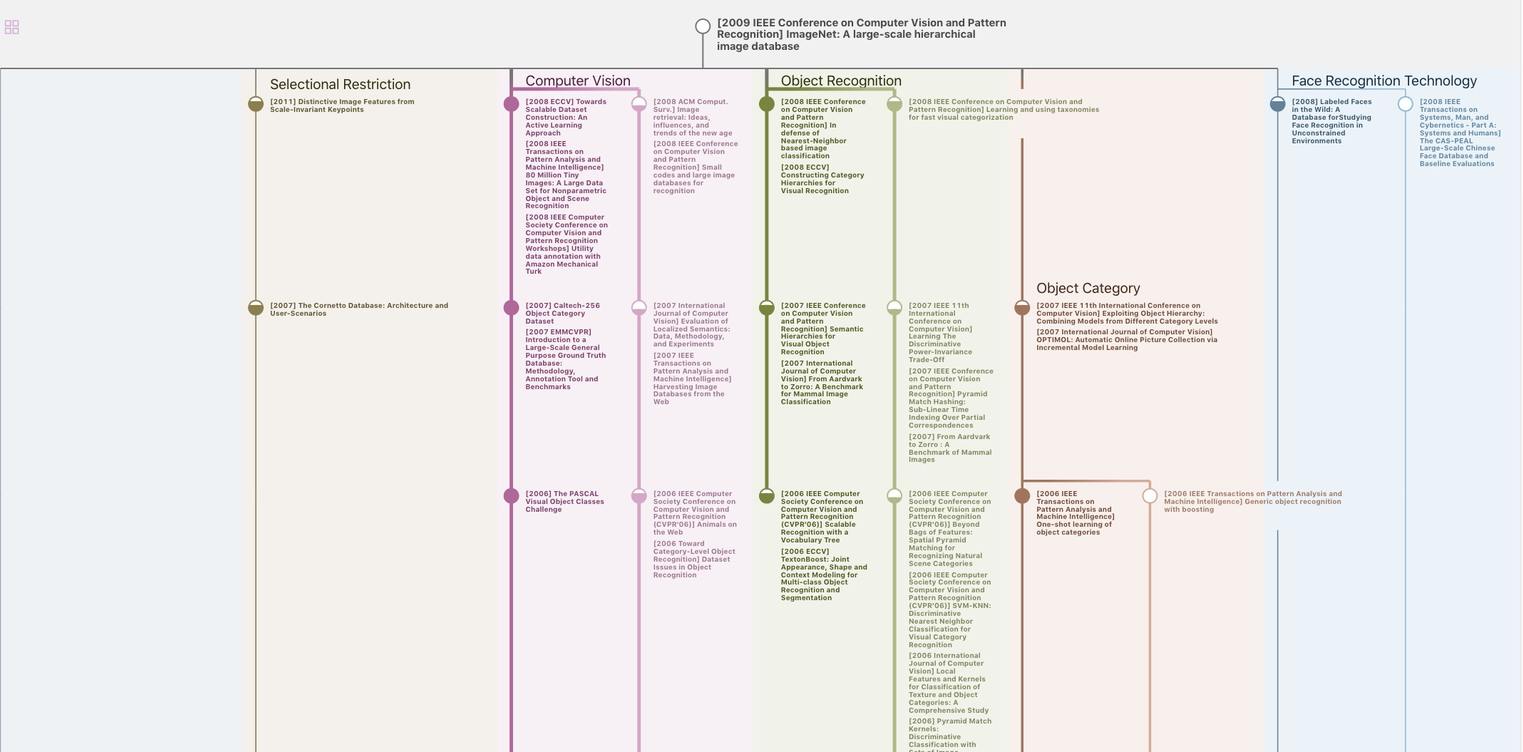
生成溯源树,研究论文发展脉络
Chat Paper
正在生成论文摘要