Counting and Locating Anything: Class-agnostic Few-shot Object Counting and Localization
2023 IEEE INTERNATIONAL CONFERENCE ON MULTIMEDIA AND EXPO, ICME(2023)
摘要
We focus on a new task for class-agnostic object localization (CAOL), which not only counts objects of any class but also outputs their point locations. Given one or more examples of objects of interest, the CAOL model should output the count and point locations of objects of interest in the query image. We propose two solutions: (1) a post-processing method for class-agnostic object counting models based on density maps, called Maximum Search Strategy (MSS), which converts density maps into specific point coordinates without the need for a threshold. (2) a point-based Class-Agnostic Counting and Localization (CACL) model that directly predicts points for each object of interest, providing an end-to-end solution without the need for post-processing; While MSS obtains promising localization results as long as good quality density map is available, CACL achieves state-of-the-art results for both counting and localization tasks. Our experiments on the CARPK dataset demonstrate the strong generalization performance of CACL model.
更多查看译文
关键词
class-agnostic counting and localization,class-agnostic counting,few-shot object counting
AI 理解论文
溯源树
样例
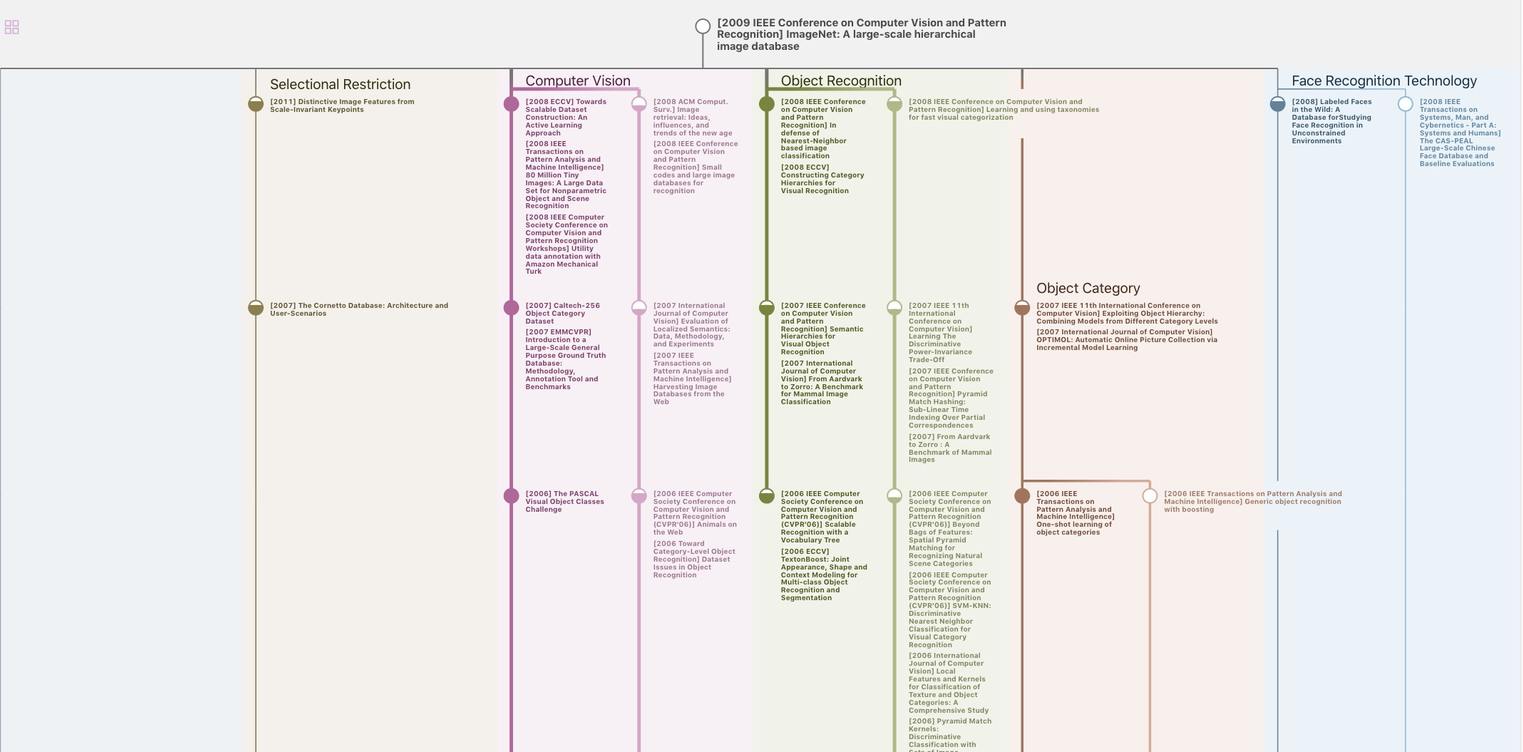
生成溯源树,研究论文发展脉络
Chat Paper
正在生成论文摘要