Design of Anti‐jamming Decision‐making for Cognitive Radar
IET RADAR SONAR AND NAVIGATION(2024)
Abstract
With the development of electronic warfare, anti-jamming measure becomes more and more complex. There have been certain research results on jamming strategies, but only a few research materials on anti-jamming strategies. It is difficult to simulate the real jamming environment, and there is no appropriate anti-jamming decision-making model for research. Cognitive radar can perceive the environment and receive feedback, which provides the possibility to solve the problem of anti-jamming decision-making. This article regards the anti-jamming measure as a kind of interaction behaviour and establishes the cognitive radar antagonistic environment model and uses the reinforcement learning algorithm to solve the problem of anti-jamming decision-making. Finally, this article verifies the feasibility of applying reinforcement learning theory on making anti-jamming decision in the radar antagonistic environment model. The performance of different reinforcement learning algorithms is compared, and their advantages and disadvantages are discussed. This work proposes an intelligent anti-jamming decision-making scheme designed for the cognitive jamming to an anti-jamming interaction mode. This scheme is suitable for scenarios with varying jamming types and the capability to predict jamming behaviour.image
MoreTranslated text
Key words
cognitive radio,decision making,jamming,markov processes,radar,radar signal processing,radiofrequency interference
求助PDF
上传PDF
View via Publisher
AI Read Science
AI Summary
AI Summary is the key point extracted automatically understanding the full text of the paper, including the background, methods, results, conclusions, icons and other key content, so that you can get the outline of the paper at a glance.
Example
Background
Key content
Introduction
Methods
Results
Related work
Fund
Key content
- Pretraining has recently greatly promoted the development of natural language processing (NLP)
- We show that M6 outperforms the baselines in multimodal downstream tasks, and the large M6 with 10 parameters can reach a better performance
- We propose a method called M6 that is able to process information of multiple modalities and perform both single-modal and cross-modal understanding and generation
- The model is scaled to large model with 10 billion parameters with sophisticated deployment, and the 10 -parameter M6-large is the largest pretrained model in Chinese
- Experimental results show that our proposed M6 outperforms the baseline in a number of downstream tasks concerning both single modality and multiple modalities We will continue the pretraining of extremely large models by increasing data to explore the limit of its performance
Upload PDF to Generate Summary
Must-Reading Tree
Example
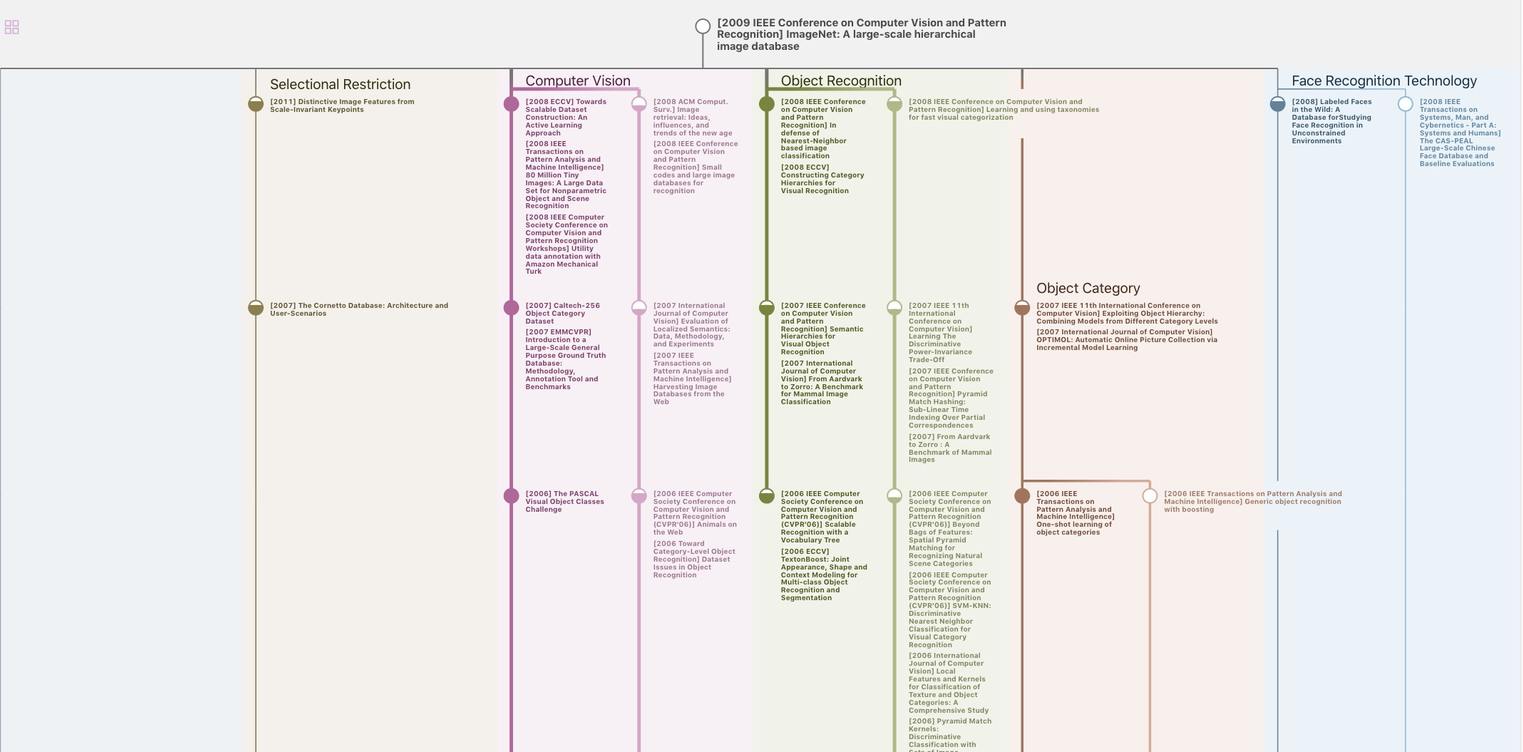
Generate MRT to find the research sequence of this paper
Related Papers
2010
被引用218 | 浏览
2007
被引用65 | 浏览
2018
被引用45 | 浏览
2017
被引用3 | 浏览
2020
被引用20 | 浏览
2021
被引用4 | 浏览
2022
被引用6 | 浏览
2023
被引用13 | 浏览
2023
被引用8 | 浏览
Data Disclaimer
The page data are from open Internet sources, cooperative publishers and automatic analysis results through AI technology. We do not make any commitments and guarantees for the validity, accuracy, correctness, reliability, completeness and timeliness of the page data. If you have any questions, please contact us by email: report@aminer.cn
Chat Paper