A Cost-Sensitive Machine Learning Model With Multitask Learning for Intrusion Detection in IoT
IEEE TRANSACTIONS ON INDUSTRIAL INFORMATICS(2024)
摘要
A problem with machine learning (ML) techniques for detecting intrusions in the Internet of Things (IoT) is that they are ineffective in the detection of low-frequency intrusions. In addition, as ML models are trained using specific attack categories, they cannot recognize unknown attacks. This article integrates strategies of cost-sensitive learning and multitask learning into a hybrid ML model to address these two challenges. The hybrid model consists of an autoencoder for feature extraction and a support vector machine (SVM) for detecting intrusions. In the cost-sensitive learning phase for the class imbalance problem, the hinge loss layer is enhanced to make a classifier strong against low-distributed intrusions. Moreover, to detect unknown attacks, we formulate the SVM as a multitask problem. Experiments on the UNSW-NB15 and BoT-IoT datasets demonstrate the superiority of our model in terms of recall, precision, and F1-score averagely 92.2%, 96.2%, and 94.3%, respectively, over other approaches.
更多查看译文
关键词
Internet of Things,Support vector machines,Intrusion detection,Costs,Training,Task analysis,Mathematical models,Deep learning (DL),Internet of things (IoT),intrusion detection,multitask learning,support vector machine (SVM)
AI 理解论文
溯源树
样例
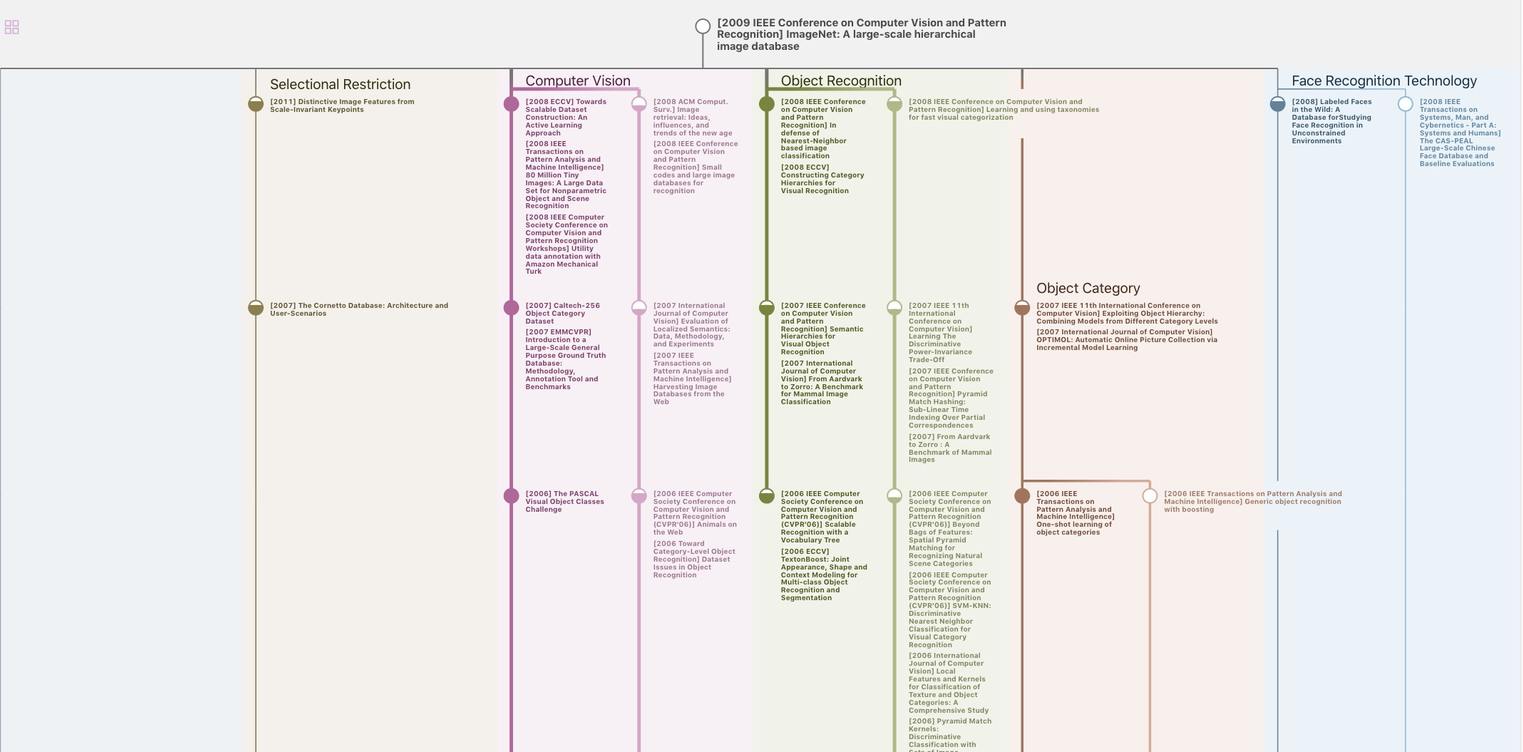
生成溯源树,研究论文发展脉络
Chat Paper
正在生成论文摘要