FusionDiff: Multi-focus image fusion using denoising diffusion probabilistic models
EXPERT SYSTEMS WITH APPLICATIONS(2024)
摘要
Multi-focus image fusion (MFIF) is an image enhancement technology with broad application prospects that can effectively extend the depth-of-field of optical lenses. This paper presents a novel MFIF method, named FusionDiff, based on denoising diffusion probabilistic models (DDPM). FusionDiff uses DDPM to fuse two source images by iteratively performing multiple denoising operations. To predict noise accurately and train the model efficiently, a lightweight U-Net architecture is designed as the conditional noise predictor. FusionDiff does not depend on any specific activity level measurement method, fusion rule or complex feature extraction network. It overcomes many algorithm design and training difficulties in existing image fusion methods. FusionDiff is noise-resistant and can still produce outstanding fusion results from source images with noise interference. In addition, FusionDiff is a few-shot learning method, which makes it suitable for image fusion tasks where training samples are relatively scarce. Experiments show that FusionDiff outperforms representative state-of-the-art methods in both visual perception and quantitative metrics. The code is available at https://github.com/lmn-ning/ImageFusionhttps://github.com/lmn-ning/ImageFusion
更多查看译文
关键词
Multi-focus image fusion,Denoising diffusion probabilistic models,Few-shot learning,Noise-robust method
AI 理解论文
溯源树
样例
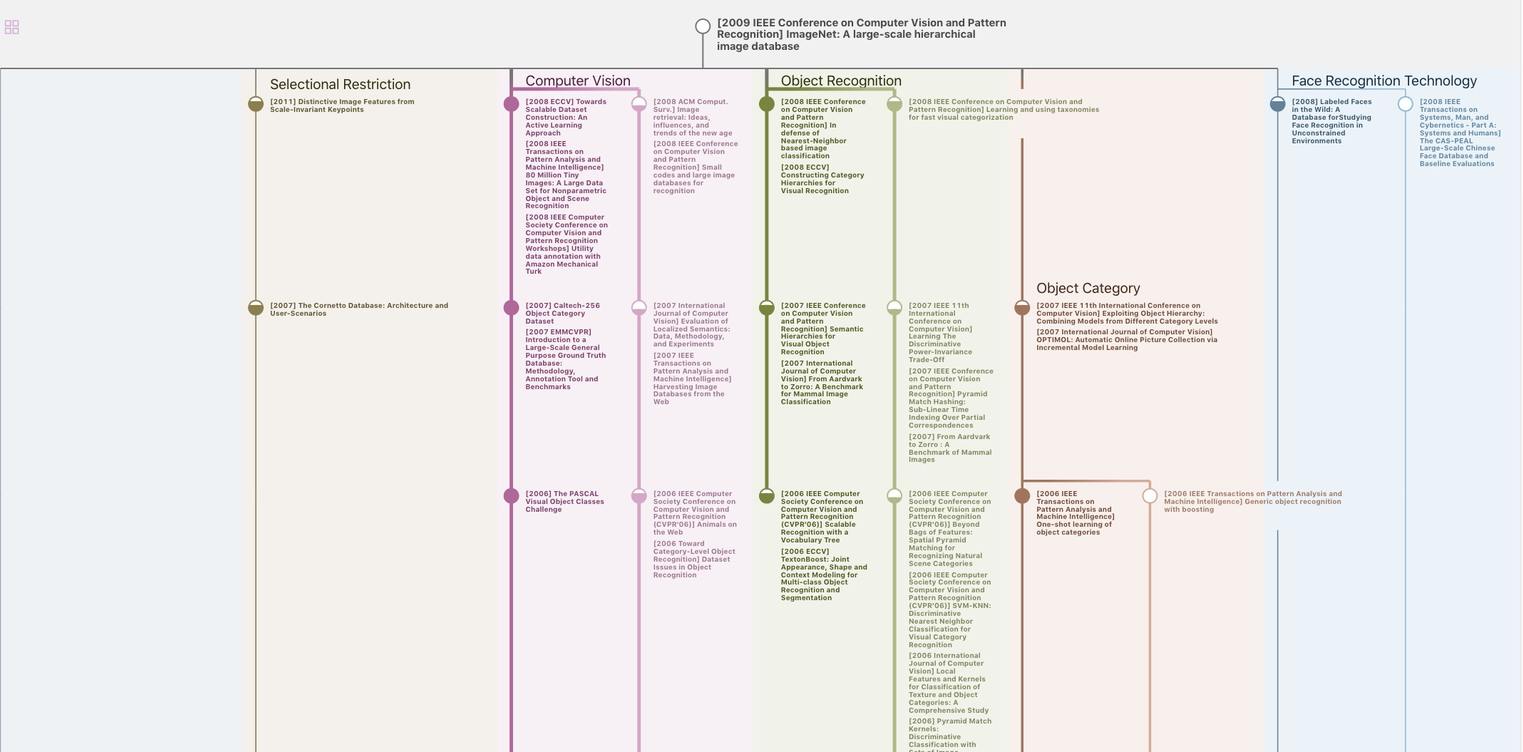
生成溯源树,研究论文发展脉络
Chat Paper
正在生成论文摘要