Descent Property in Sequential Second-Order Cone Programming for Nonlinear Trajectory Optimization
JOURNAL OF GUIDANCE CONTROL AND DYNAMICS(2023)
摘要
Sequential second-order cone programming (SSOCP) is commonly used in aerospace applications for solving nonlinear trajectory optimization problems. The SSOCP possesses good real-time performance. However, one long-standing challenge is its unguaranteed convergence. In this paper, we theoretically analyze the descent property of the L1 penalty function in the SSOCP. Using Karush-Kuhn-Tucker conditions, we obtain two important theoretical results: 1) the L1 penalty function of the original nonlinear problem always descends along the iteration direction; 2) a sufficiently small trust region can decrease the L1 penalty function. Based on these two results, we design an improved trust region shrinking algorithm with theoretically guaranteed convergence. In numerical simulations, we verify the proposed algorithm using a reentry trajectory optimization problem.
更多查看译文
关键词
nonlinear trajectory optimization,cone,second-order second-order
AI 理解论文
溯源树
样例
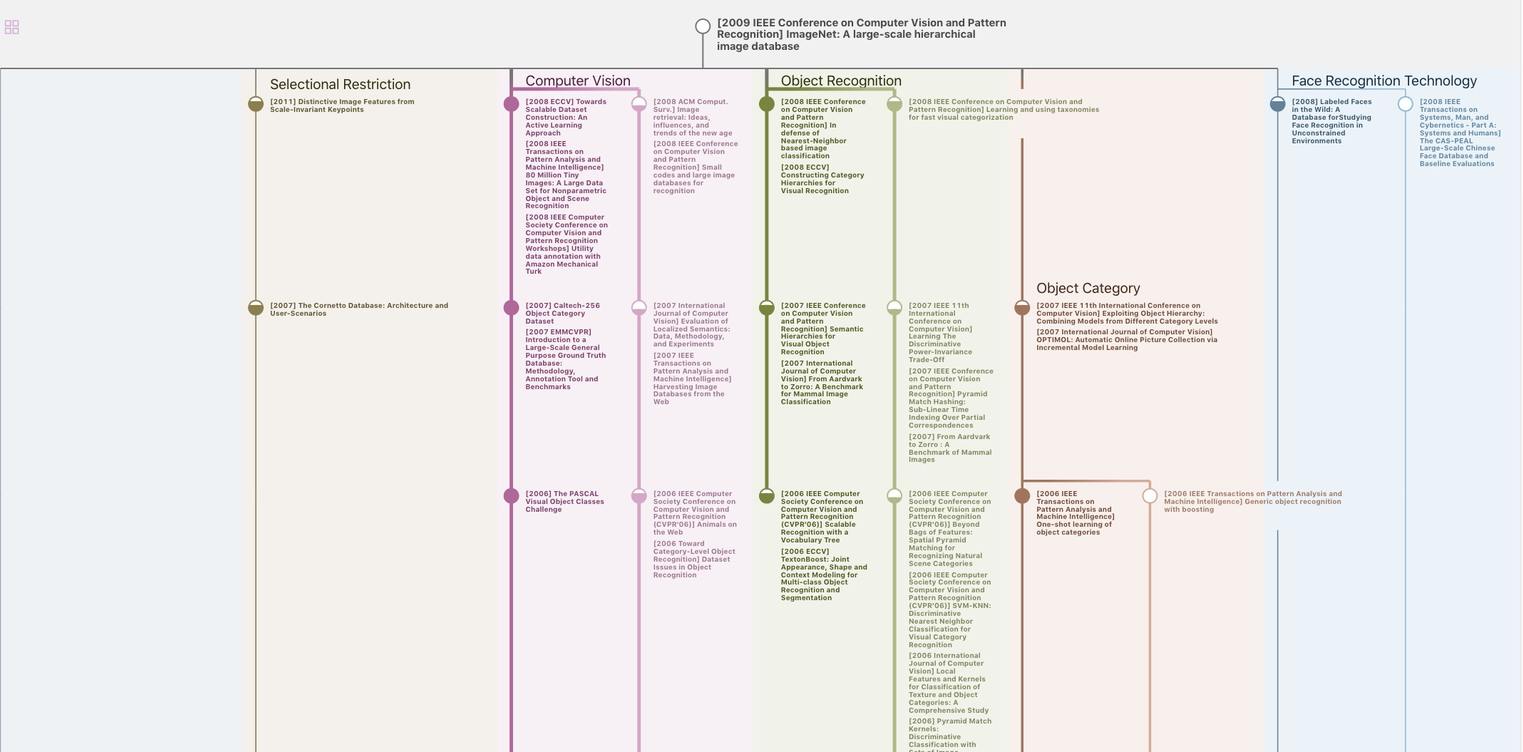
生成溯源树,研究论文发展脉络
Chat Paper
正在生成论文摘要