Making decisions with evidential probability and objective Bayesian calibration inductive logics
INTERNATIONAL JOURNAL OF APPROXIMATE REASONING(2023)
摘要
Calibration inductive logics are based on accepting estimates of relative frequencies, which are used to generate imprecise probabilities. In turn, these imprecise probabilities are intended to guide beliefs and decisions — a process called “calibration”. Two prominent examples are Henry E. Kyburg's system of Evidential Probability and Jon Williamson's version of Objective Bayesianism. There are many unexplored questions about these logics. How well do they perform in the short-run? Under what circumstances do they do better or worse? What is their performance relative to traditional Bayesianism?
更多查看译文
关键词
Agent-based modelling,Decision under uncertainty,Frequentist statistics,Imprecise probability,Machine learning,Objective Bayesianism
AI 理解论文
溯源树
样例
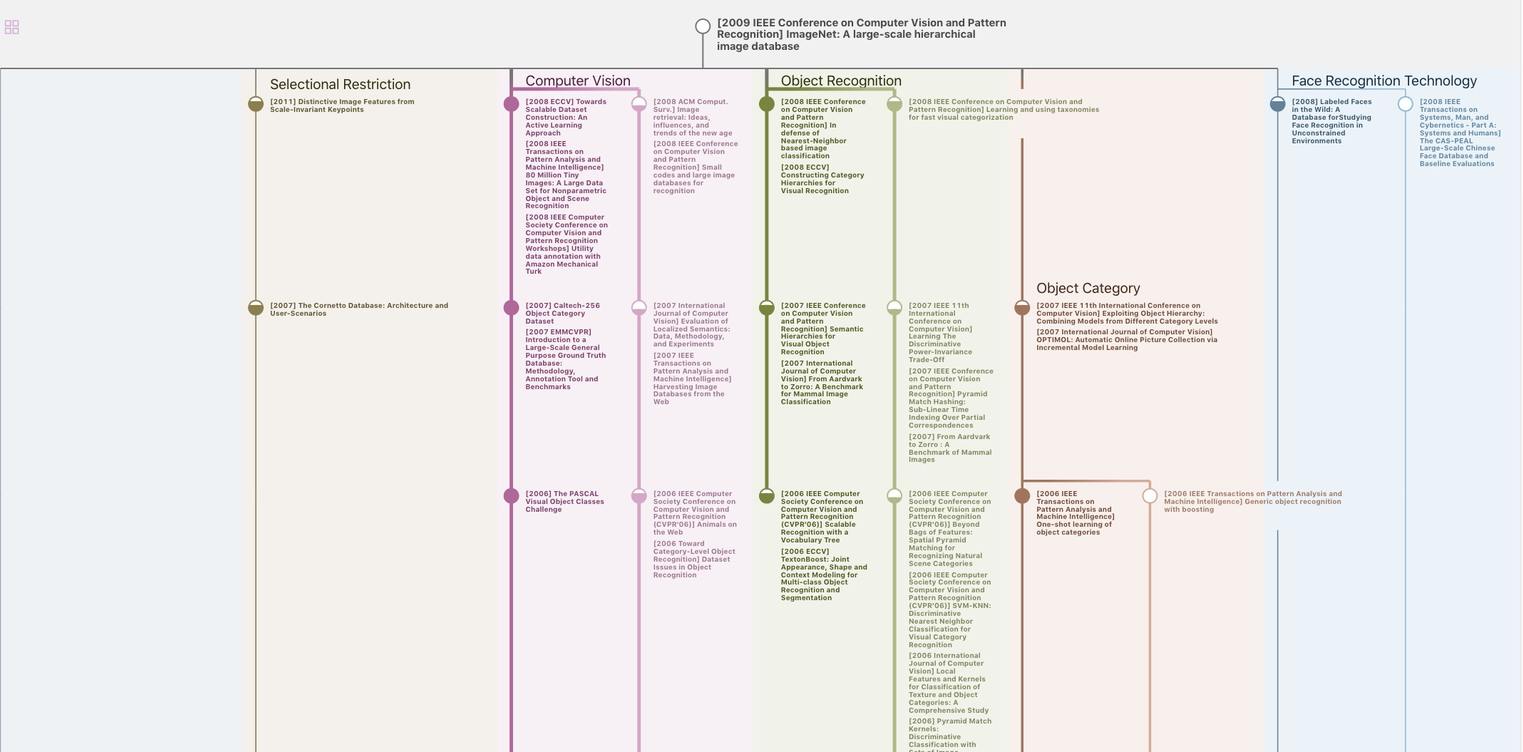
生成溯源树,研究论文发展脉络
Chat Paper
正在生成论文摘要