Bridging Many-Body Quantum Physics and Deep Learning via Tensor Networks
MATHEMATICAL ASPECTS OF DEEP LEARNING(2023)
摘要
Deep network architectures have exhibited an unprecedented ability to encompass the convoluted dependencies which characterize hard learning tasks such as image classification or speech recognition. However, some key questions regarding deep learning architecture design have no adequate theoretical answers. In the seemingly unrelated field of many-body physics, there is a growing need for highly expressive computational schemes that are able to efficiently represent highly entangled many-particle quantum systems. In this chapter, we describe a tensor network (TN) based common language that has been established between the two disciplines, which allows for bidirectional contributions. By showing that many-body wave functions are structurally equivalent to mappings of convolutional and recurrent networks, we can construct their TN descriptions and bring forth quantum entanglement measures as natural quantifiers of dependencies modeled by such networks. Accordingly, we propose a novel entanglement-based deep learning design scheme that sheds light on the success of popular architectural choices made by deep learning practitioners and suggests new practical prescriptions. In the other direction, we identify the fact that an inherent re-use of information in prominent deep learning architectures is a key trait distinguishing them from standard TN-based wave function representations. Therefore, we employ a TN manifestation of information re-use and construct TNs corresponding to deep recurrent networks and overlapping convolutional networks. This allows us to demonstrate theoretically that these architectures are powerful enough to represent highly entangled quantum systems polynomially more efficiently than the previously employed restricted Boltzmann machines. We thus provide theoretical motivation to shift trending neural-network-based wave function representations closer to state-of-the-art deep learning architectures.
更多查看译文
AI 理解论文
溯源树
样例
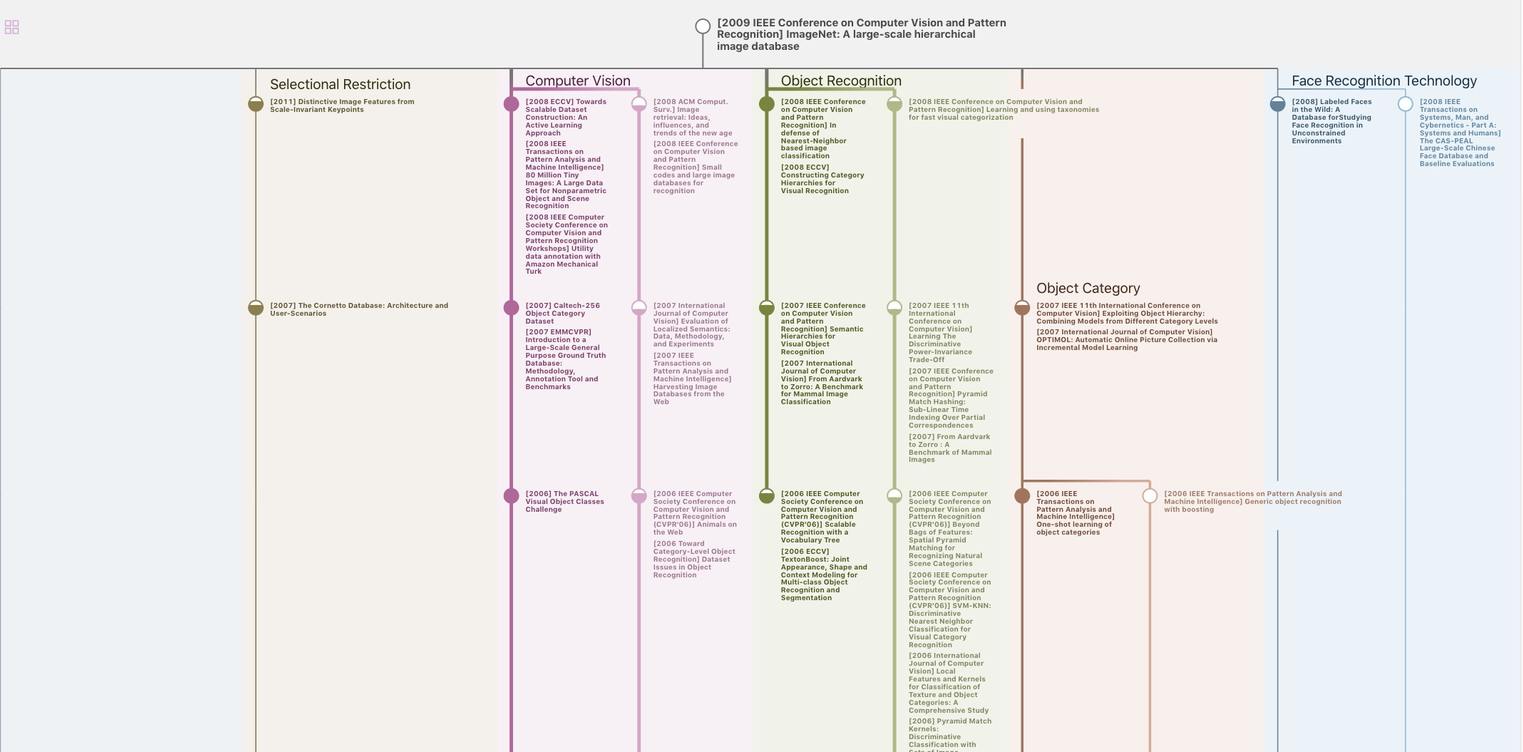
生成溯源树,研究论文发展脉络
Chat Paper
正在生成论文摘要