FT-Net: Learning Failure Recovery and Fault-Tolerant Locomotion for Quadruped Robots
IEEE ROBOTICS AND AUTOMATION LETTERS(2023)
摘要
Quadruped robots, in recent years, have been increasingly used in extremely harsh and dangerous conditions. Consequently, diverse severe hardware failures may occur at any time during the working cycle of the robots. In this work, we propose a fault-tolerant (FT) control pipeline based on model-free reinforcement learning - FT-Net, which is guided by a variable-height inverted pendulum model and support polygon. This pipeline allows the robot to dynamically and autonomously adapt to both partial and complete motor failures. Unlike conventional FT control methods that need to pinpoint the failed location, our controller identifies the fault implicitly with a neural network-based adaptor. Furthermore, we achieve a unified policy that is capable of switching from four-legged to three-legged walking mode when complete motor failure occurs. It is shown by both extensive simulation and hardware experiments that FT-Net learns to effectively perform recovery behaviors. The fault-tolerant locomotion can even be executed in various dynamic tasks and terrains.
更多查看译文
关键词
Quadruped robots,fault-tolerant control,deep reinforcement learning,self-adaptation
AI 理解论文
溯源树
样例
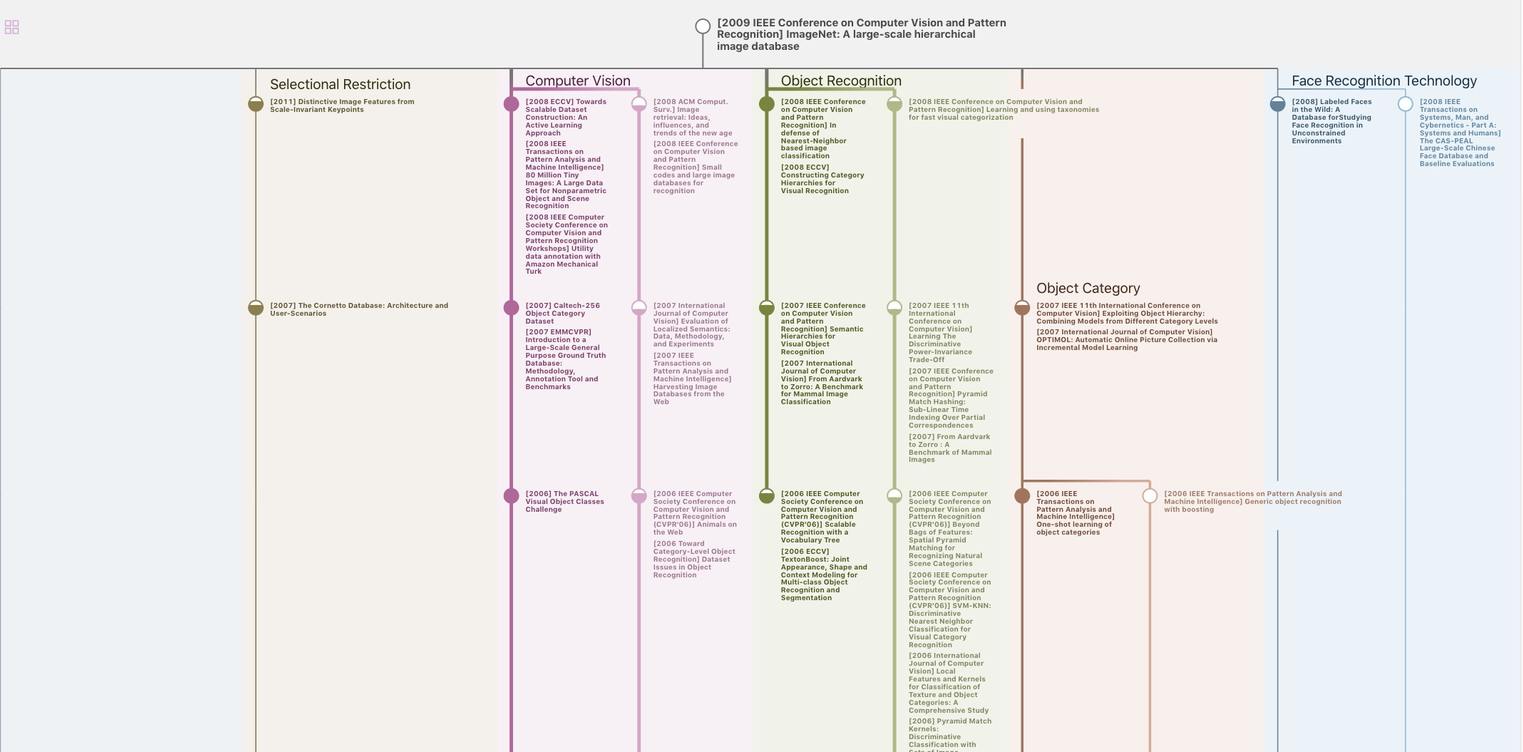
生成溯源树,研究论文发展脉络
Chat Paper
正在生成论文摘要