An Online Training Procedure for Rain Detection Models Applied to Satellite Microwave Links
IEEE Geoscience and Remote Sensing Letters(2023)
摘要
In recent years, opportunistic rainfall sensing exploiting commercial microwave links (CMLs) has become popular for real-time monitoring of rainfall events. Among such systems, satellite-to-Earth microwave links (SMLs) are gaining great interest. An important information to extract from the collected data is whether or not an SML observation corresponds to a rain condition. This letter proposes an online training procedure to keep the algorithms that classify this condition up to date, overcoming the problem of data seasonality. Specifically, we propose to retrain the algorithms after one day of observations by applying a forgetting mechanism, where the oldest data are removed from the training dataset. Data were collected by the Smart Rainfall System (SRS) SML-type sensors located in the city of Genoa (Italy). We compare three classification algorithms: one based on an anomaly detection method and two based on machine learning (ML) algorithms. The outcomes of this analysis pave the way for implementing artificial intelligence models on embedded systems with limited resources such as the electronic units of SML sensors, hence reducing the data flow from the peripheral sensor network to the central data acquisition and integration server.
更多查看译文
关键词
Machine learning (ML),rainfall prediction,satellite microwave links,Smart Rainfall System (SRS)
AI 理解论文
溯源树
样例
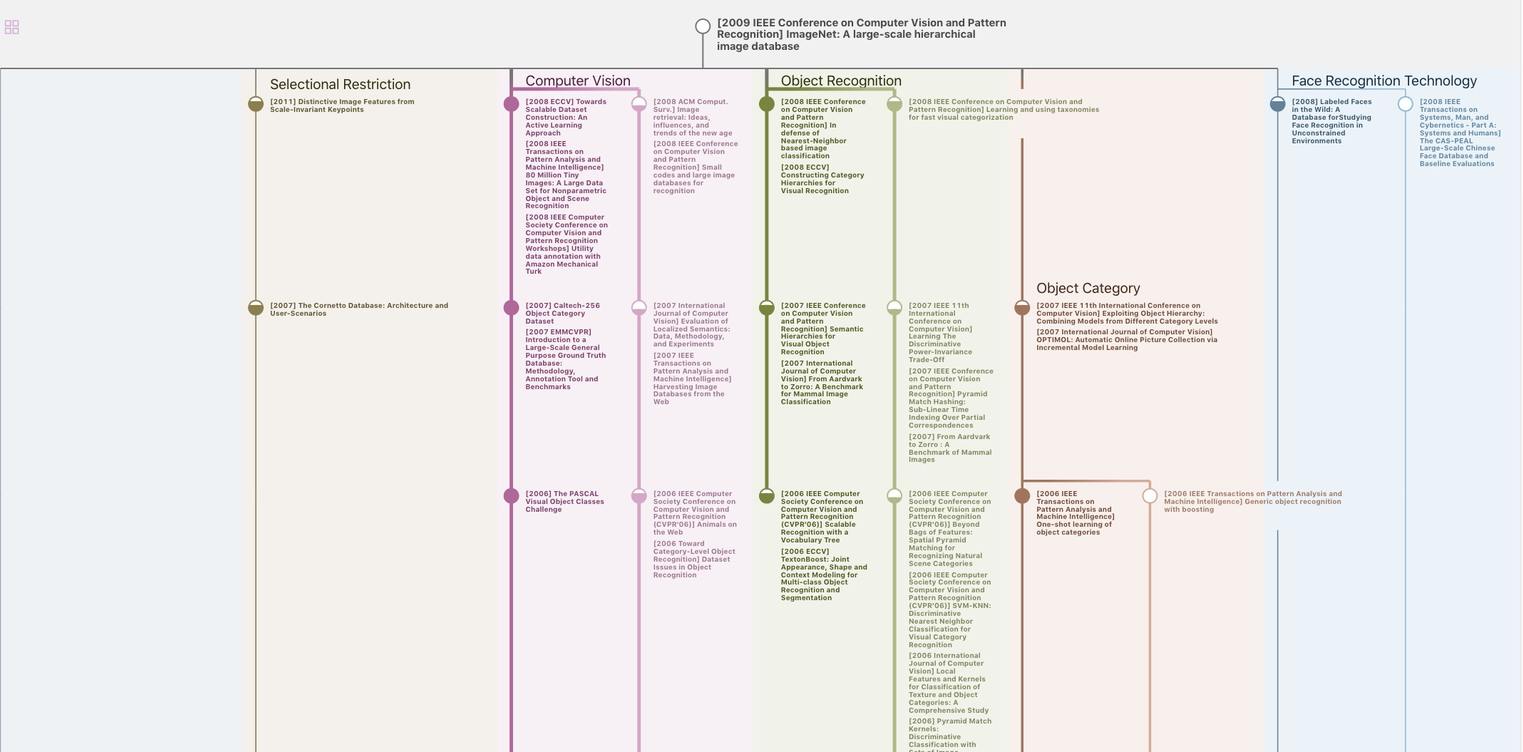
生成溯源树,研究论文发展脉络
Chat Paper
正在生成论文摘要