A Customized ADMM Approach for Large-Scale Nonconvex Semidefinite Programming
Mathematics(2023)
摘要
We investigate a class of challenging general semidefinite programming problems with extra nonconvex constraints such as matrix rank constraints. This problem has extensive applications, including combinatorial graph problems, such as MAX-CUT and community detection, reformulated as quadratic objectives over nonconvex constraints. A customized approach based on the alternating direction method of multipliers (ADMM) is proposed to solve the general large-scale nonconvex semidefinite programming efficiently. We propose two reformulations: one using vector variables and constraints, and the other further reformulating the Burer-Monteiro form. Both formulations admit simple subproblems and can lead to significant improvement in scalability. Despite the nonconvex constraint, we prove that the ADMM iterates converge to a stationary point in both formulations, under mild assumptions. Additionally, recent work suggests that in this matrix form, when the matrix factors are wide enough, the local optimum with high probability is also the global optimum. To demonstrate the scalability of our algorithm, we include results for MAX-CUT, community detection, and image segmentation.
更多查看译文
关键词
semidefinite optimization,symmetric matrix factorization,nonconvex optimization,large-scale graph problems
AI 理解论文
溯源树
样例
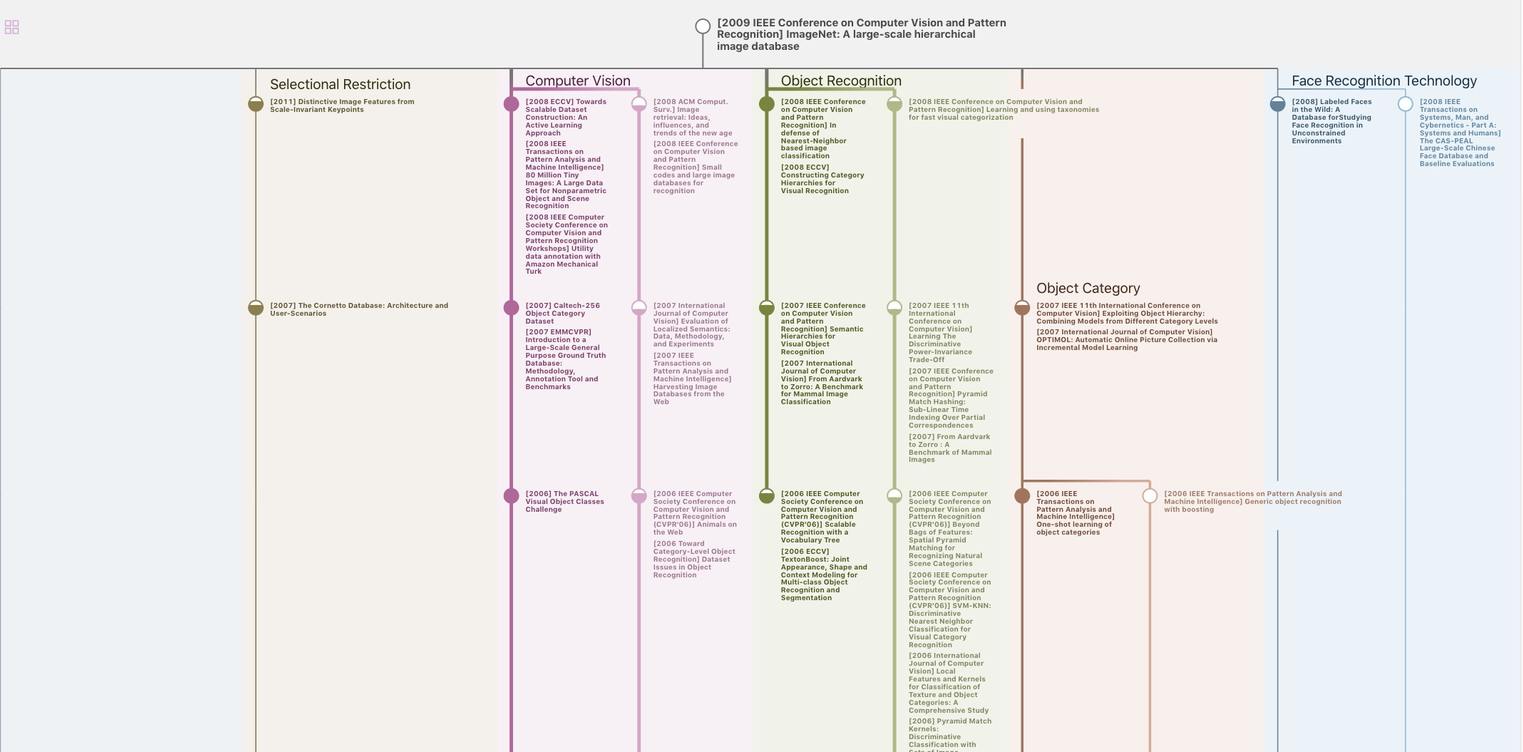
生成溯源树,研究论文发展脉络
Chat Paper
正在生成论文摘要