BioEdge: Accelerating Object Detection in Bioimages with Edge-Based Distributed Inference
Electronics(2023)
摘要
Convolutional neural networks (CNNs) have enabled effective object detection tasks in bioimages. Unfortunately, implementing such an object detection model can be computationally intensive, especially on resource-limited hardware in a laboratory or hospital setting. This study aims to develop a framework called BioEdge that can accelerate object detection using Scaled-YOLOv4 and YOLOv7 by leveraging edge computing for bioimage analysis. BioEdge employs a distributed inference technique with Scaled-YOLOv4 and YOLOv7 to harness the computational resources of both a local computer and an edge server, enabling rapid detection of COVID-19 abnormalities in chest radiographs. By implementing distributed inference techniques, BioEdge addresses privacy concerns that can arise when transmitting biomedical data to an edge server. Additionally, it incorporates a computationally lightweight autoencoder at the split point to reduce data transmission overhead. For evaluation, this study utilizes the COVID-19 dataset provided by the Society for Imaging Informatics in Medicine (SIIM). BioEdge is shown to improve the inference latency of Scaled-YOLOv4 and YOLOv7 by up to 6.28 times with negligible accuracy loss compared to local computer execution in our evaluation setting.
更多查看译文
关键词
object detection,bioimages,edge-based
AI 理解论文
溯源树
样例
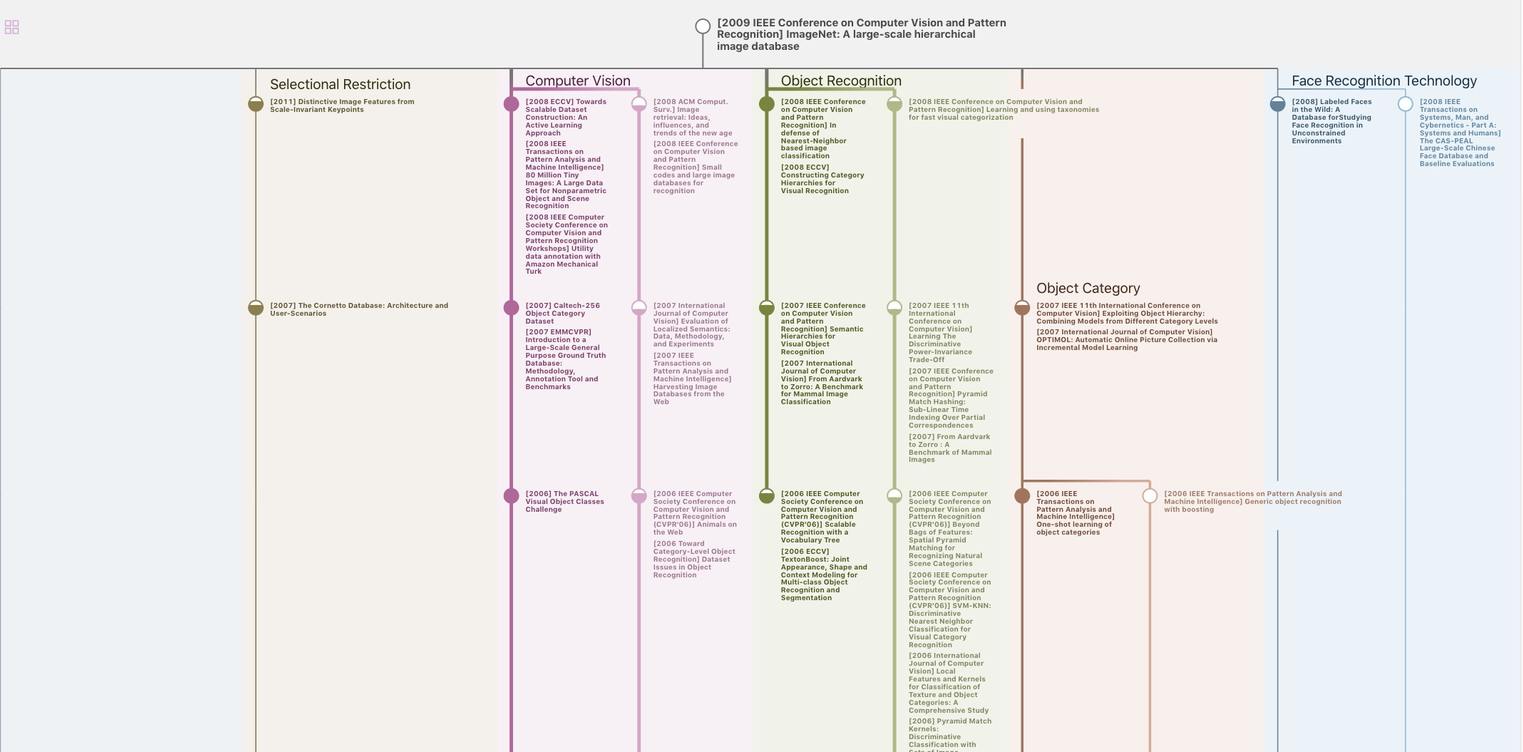
生成溯源树,研究论文发展脉络
Chat Paper
正在生成论文摘要