Self-Supervised Non-Uniform Low-Light Image Enhancement Combining Image Inversion and Exposure Fusion
Electronics(2023)
摘要
Low-light image enhancement is a challenging task in non-uniform low-light conditions, often resulting in local overexposure, noise amplification, and color distortion. To obtain satisfactory enhancement results, most models must resort to carefully selected paired or multi-exposure data sets. In this paper, we propose a self-supervised framework for non-uniform low-light image enhancement to address these issues, only requiring low-light images on their own for training. We first design a robust Retinex model-based image exposure enhancement network (EENet) to obtain global brightness enhancement and noise removal of images by carefully designing the loss function of each decomposition map. Then, to correct overexposed areas in the enhanced image, we incorporate the inverse image of the low-light image for enhancement using EENet. Furthermore, a three-branch asymmetric exposure fusion network (TAFNet) is designed. The two enhanced images and the original image are used as the TAFNet inputs to obtain a globally well-exposed and detail-rich image. Experimental results demonstrate that our framework outperforms some state-of-the-art methods in visual and quantitative comparisons.
更多查看译文
关键词
unsupervised learning, non-uniform low-light image, image enhancement, multi-exposure image fusion
AI 理解论文
溯源树
样例
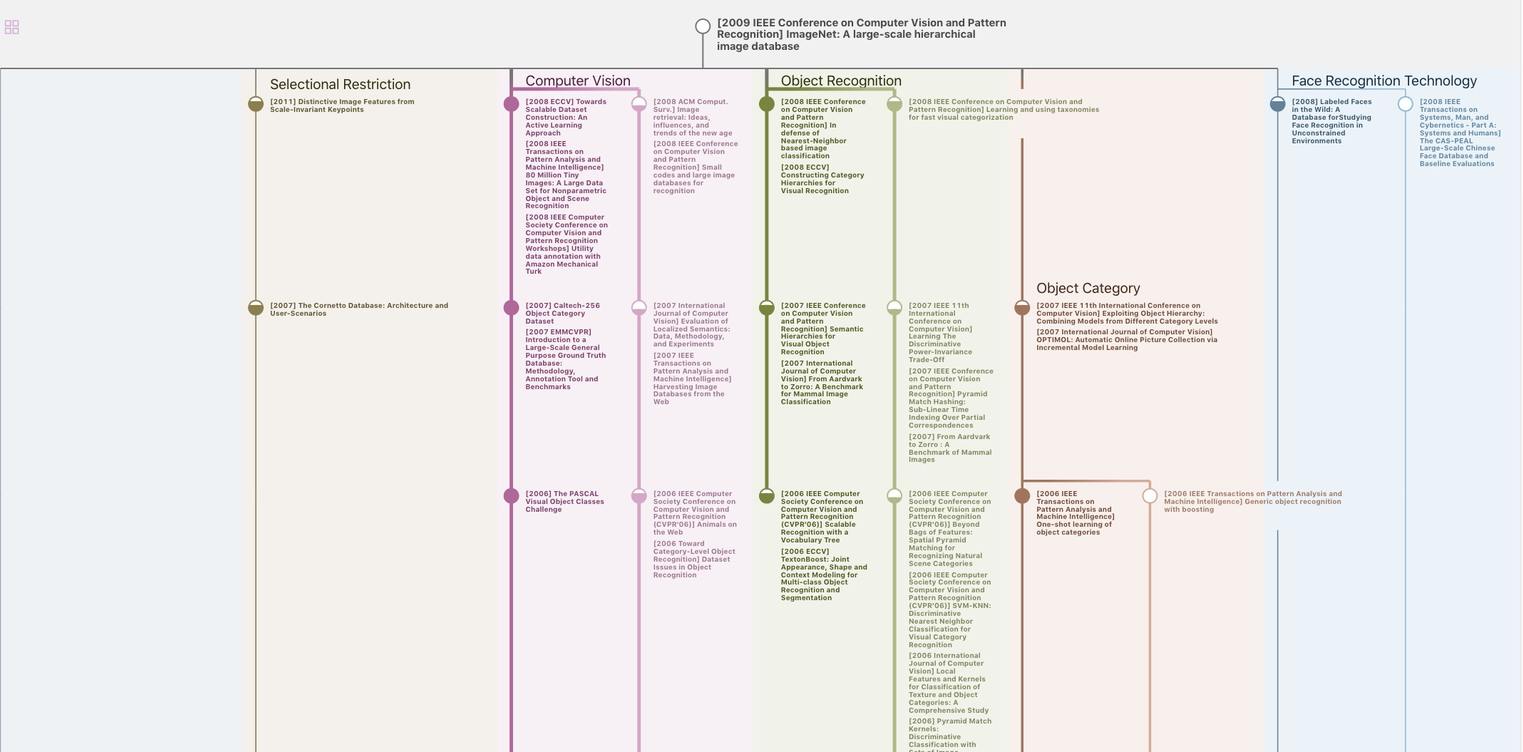
生成溯源树,研究论文发展脉络
Chat Paper
正在生成论文摘要