155 Evaluation of in silico tools and chat-GPT to determine the impact of missense variants of immune-related genes associated with immunotherapy outcomes for solid tumors
Journal for ImmunoTherapy of Cancer(2023)
摘要
BackgroundAdvancements in next-generation sequencing (NGS) technology have revealed a significant number of variants of unknown significance (VUS) in cancer, lacking a clear classification regarding their impact on cancer treatment.1 With the integration of immunotherapy as a standard treatment, understanding the influence of these variants on immunotherapy outcomes has become increasingly imperative. Numerous in silico tools have been developed to categorize these variants based on their pathogenicity and to provide insight into their clinical actionability. Due to algorithmic differences, these tools often generate inconsistent predictions for pathogenicity.2 3 This study evaluates the performance of six widely utilized in silico tools in predicting the pathogenicity of potentially actionable missense variants.MethodsWe conducted the performance assessment of 5 in silico tools plus generative Artifical Intelligence (AI): Chat-GPT; PolyPhen-2, Align-GVGD, MutationTaster2, CADD, and REVEL. We constructed a dataset with the variants of genes known to impact immune therapy responses (POLE, STK11, PTEN, KEAP1, SMAD4, SMARCA4, TP53, PTEN, CDKN2A). The most frequently mentioned variants classified as pathogenic (n=80) or benign (n=80) in various databases (OncoKB, Cancer Hotspots, CIViC, My Cancer Genome, AACR Project GENIE) were selected, and the top ten variants with the highest frequencies were compared with ClinVar. Accuracy, sensitivity, specificity, and Matthews correlation coefficient (MCC) of each in silico tool was calculated.ResultsMutation Taster 2021 showed the highest overall accuracy (0.83) and, MCC (0.69) of the in silico tools analyzed. Chat-GPT and REVEL showed 100% specificity and 100% positive predictive value. Align-GVGD (0.52) had the lowest overall accuracy and the lowest MCC (0.06), with the other in-silico tools ranging from 0.36 to 0.69. CADD demonstrated the highest (1.00) sensitivity and negative predictive value (1.00) and REVEL and chat-GPT performed the lowest (0.22).ConclusionsThe above results demonstrate that no single tool alone is sufficient to adequately determine the pathogenicity of a mutation variant, due to the significant disparity in sensitivity and specificity. High specificity of REVEL and Chat-GPT indicate that these tools are effective in ruling in pathogenic variants accurately while avoiding false positive results. Conversely, CADD could be used to rule out pathogenic variants, preventing false negative results. MutationTaster2021 appeared to be the most reliable as it showed sensitivity above 90 while having the highest MCC and overall accuracy. However, healthcare professionals should be aware of the limitations in currently available in silico tools and chat-GPT when assessing the potential pathogenicity of genetic variants of immune related-genes associated with immunotherapy outcomes.AcknowledgementsWe appreciate our supervisor, Dr. Perez Hermida de Viveiros, for guidance and unwavering support throughout the duration of this study. We would like to thank the members of the research team for their dedicated efforts, collaboration, and fruitful discussions during the course of this project. YC and CJ were responsible for the whole frame of this manuscript. GK, PK, LC thoroughly reviewed the manuscript. Each author has participated sufficiently in the manuscript to take responsibility for appropriate portions of the content. All authors have given final approval of the version to be published.ReferencesFederici G, Soddu S. Variants of uncertain significance in the era of high-throughput genome sequencing: a lesson from breast and ovary cancers. J Exp Clin Cancer Res. 2020 Mar 4;39(1):46. doi: 10.1186/s13046–020-01554–6. PMID: 32127026; PMCID: PMC7055088. Katsonis P, Wilhelm K, Williams A, Lichtarge O. Genome interpretation using in silico predictors of variant impact. Hum Genet. 2022 Oct;141(10):1549–1577. doi: 10.1007/s00439–022-02457–6. Epub 2022 Apr 30. PMID: 35488922; PMCID: PMC9055222. Leichsenring J, Horak P, Kreutzfeldt S, Heining C, Christopoulos P, Volckmar AL, Neumann O, Kirchner M, Ploeger C, Budczies J, Heilig CE, Hutter B, Fröhlich M, Uhrig S, Kazdal D, Allgäuer M, Harms A, Rempel E, Lehmann U, Thomas M, Pfarr N, Azoitei N, Bonzheim I, Marienfeld R, Möller P, Werner M, Fend F, Boerries M, von Bubnoff N, Lassmann S, Longerich T, Bitzer M, Seufferlein T, Malek N, Weichert W, Schirmacher P, Penzel R, Endris V, Brors B, Klauschen F, Glimm H, Fröhling S, Stenzinger A. Variant classification in precision oncology. Int J Cancer. 2019 Dec 1;145(11):2996–3010. doi: 10.1002/ijc.32358. Epub 2019 May 21. PMID: 31008532.
更多查看译文
关键词
immunotherapy outcomes,missense variants,chat-gpt,immune-related
AI 理解论文
溯源树
样例
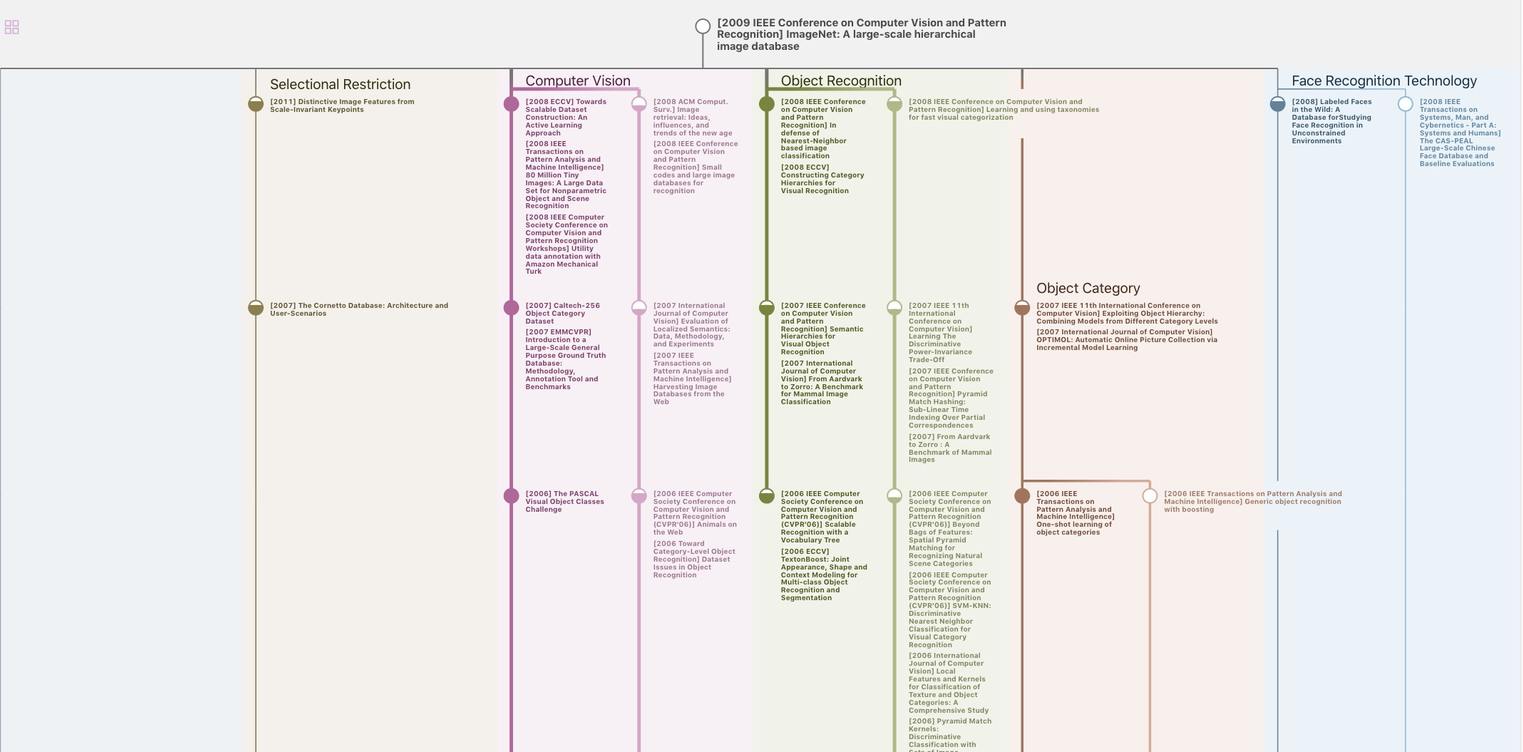
生成溯源树,研究论文发展脉络
Chat Paper
正在生成论文摘要