Leveraging Sparse Approximation for Monaural Overlapped Speech Separation From Auditory Perspective
IEEE ACCESS(2023)
摘要
Neuroscience suggests that the sparse behavior of a neural population underlies the mechanisms of the auditory system for monaural overlapped speech separation. This study investigates leveraging sparse approximation to improve speech separation in a conventional deep learning algorithm. We develop a combined model that embeds a sparse approximation algorithm, a multilayered iterative soft thresholding algorithm (ML-ISTA), into a conventional time-domain-based speech separation algorithm, Conv-TasNet. Adopting ML-ISTA is a crucial enabler for the embedding process and helps avoid solving a bi-level optimization problem comprising sparse approximation and speech separation. ML-ISTA performs sparse approximation through forward calculations, thereby eliminating the optimization of sparse approximation. The combined model is trained with WSJ0-2mix, the Wall Street Journal English corpus for two-speaker mixed speech without noisy or reverberant interference, to clarify the proposed method's performance. The model demonstrates that sparse approximation improves separation performance regardless of the approximation setting. The peak performance of the model exceeds that of Conv-TasNet by 1.1% to 4.7% in four speech quality criteria. Moreover, sparse approximation accelerates the combined model performance gain at the early stages of learning relative to Conv-TasNet. The primary novelty of the study is embedding the sparse approximation algorithm, ML-ISTA, into a deep-learning-based speech separation framework and the experimental proof of improved separation performance in the proposed algorithm.
更多查看译文
关键词
Sparse representation,Approximation algorithms,Behavioral sciences,Auditory system,Speech enhancement,Optimization,Neurons,Neuroscience,Deep learning,Speech processing,sparse approximation,sparsity,speech separation
AI 理解论文
溯源树
样例
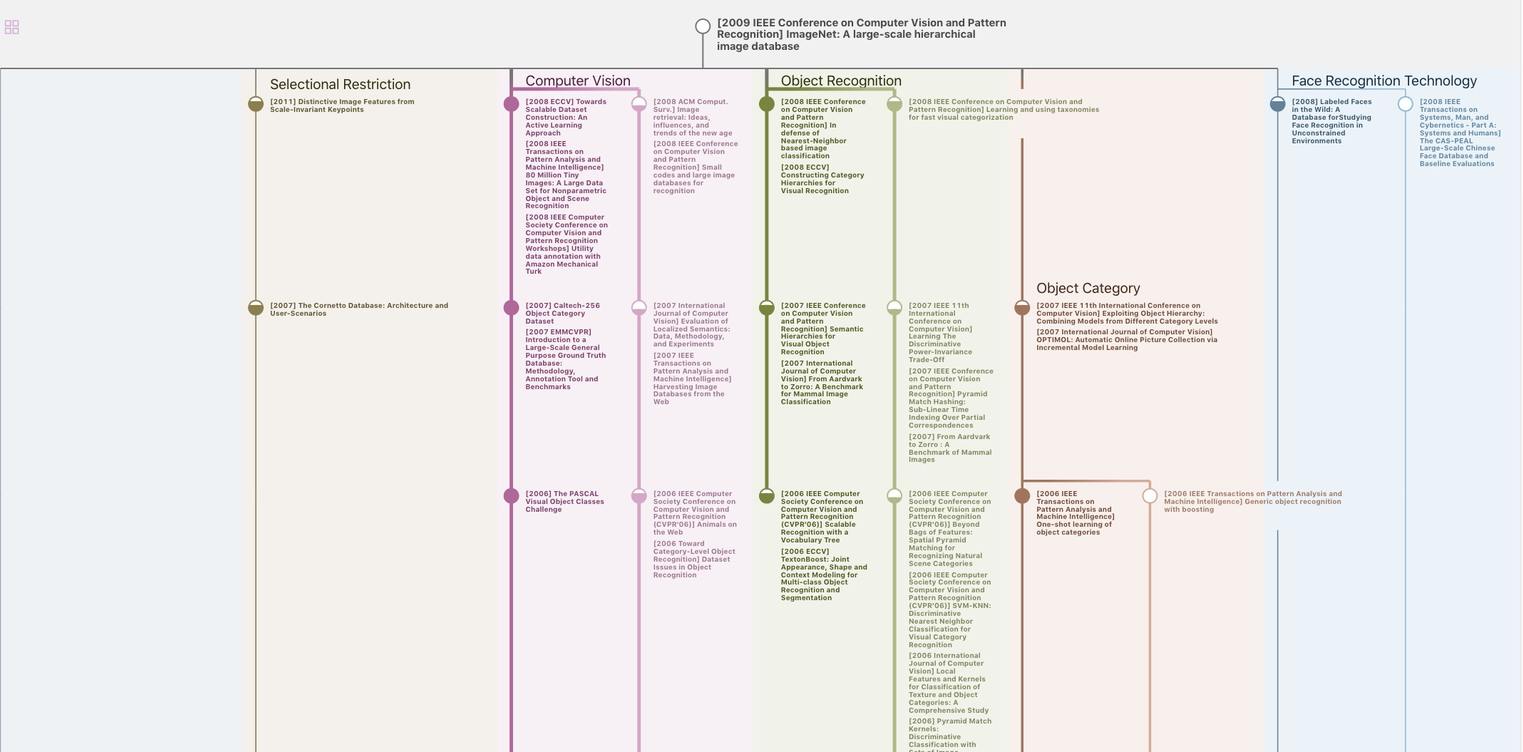
生成溯源树,研究论文发展脉络
Chat Paper
正在生成论文摘要