Frequency-Domain Multi-Exposure HDR Imaging Network With Representative Image Features
IEEE Access(2023)
摘要
Constructing a high dynamic range (HDR) image from multi-exposure low dynamic range (LDR) images is challenging mainly due to two major problems. One is a large misalignment between the LDR images taken at different moments due to camera and object motions. The other is missing content in over- or under-exposed areas, which can be very large in the LDR images with long or short exposure times. These problems lead to ghosting artifacts or saturated regions in the reconstructed HDR image. In this paper, we propose a method using convolutional neural networks (CNNs) to address these problems. Specifically, each LDR image is fed to a two-branch CNN that extracts two kinds of features separately, named content features and global features. Then, another CNN aligns and merges the features to create the HDR output. The content features are used to create spatial attention masks, which are used to align the features in the time domain with exposure-invariant attributes. Further, Fourier coefficient vectors of global features are utilized to modulate intermediate features in the frequency domain during the reconstruction process. Experimental results show that our method achieves state-of-the-art HDR reconstruction performances on several benchmarks. Our code is available at https://github.com/KeuntekLee/FDM-HDR.
更多查看译文
关键词
Feature extraction,Image reconstruction,Image processing,Cameras,Optical flow,Brightness,Frequency-domain analysis,Convolutional neural networks,frequency domain feature modulating,high dynamic range imaging,multi-exposure fusion
AI 理解论文
溯源树
样例
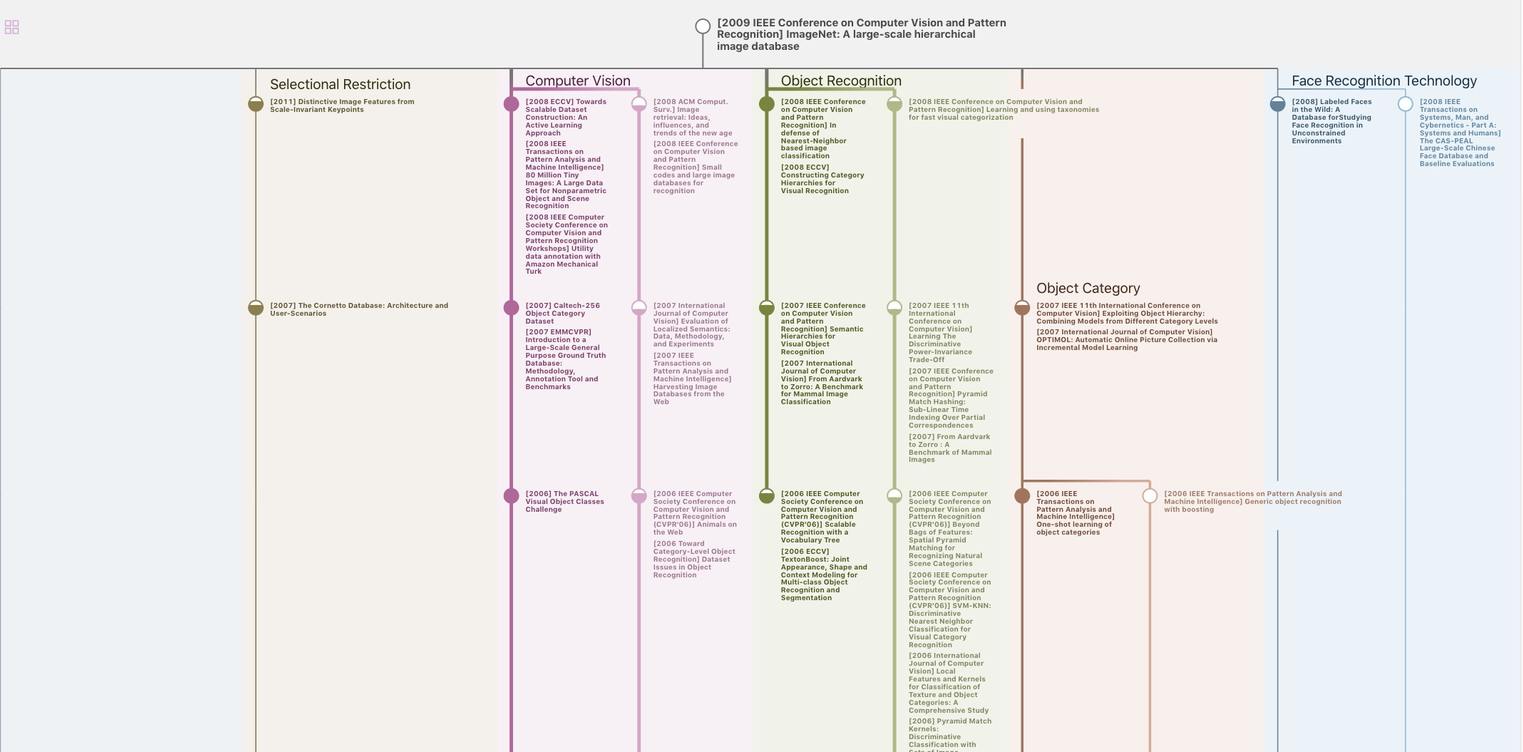
生成溯源树,研究论文发展脉络
Chat Paper
正在生成论文摘要