Towards Rapid Autonomous Electron Microscopy with Active Meta-Learning.
SC-W '23: Proceedings of the SC '23 Workshops of The International Conference on High Performance Computing, Network, Storage, and Analysis(2023)
摘要
We introduce a novel approach, Active Meta-learning, to improve computational control across various scientific experiments. It's particularly valuable for spectral reconstruction in STEM EELS nanoparticle plasmonic images. Traditionally, separate AI models were trained for each experiment via active learning, but this approach could face scalability issues with high-resolution data and the need for complex AI models due to intricate structure-property relationships. In this work we demonstrate the feasibility of learning AI structural representations across multiple experiments. We train a meta model from 10 prior experiments carried out such that the model can adapt to new unseen conditions in considerably less time than when trained from scratch. We utilize the Reptile algorithm, a first-order, model-agnostic meta-learning approach. To enhance and expand the meta-training dataset, conventional computer vision methods are applied to augment images from previous experiments. We observe up to ∼30-40% reduction in the number of training epochs for active learning exploration. The approach will be extended to distributed meta-learning workflows; meta-model trained in HPC datacenter using data from different microscopy sites and pushed to individual sites for active learning.
更多查看译文
AI 理解论文
溯源树
样例
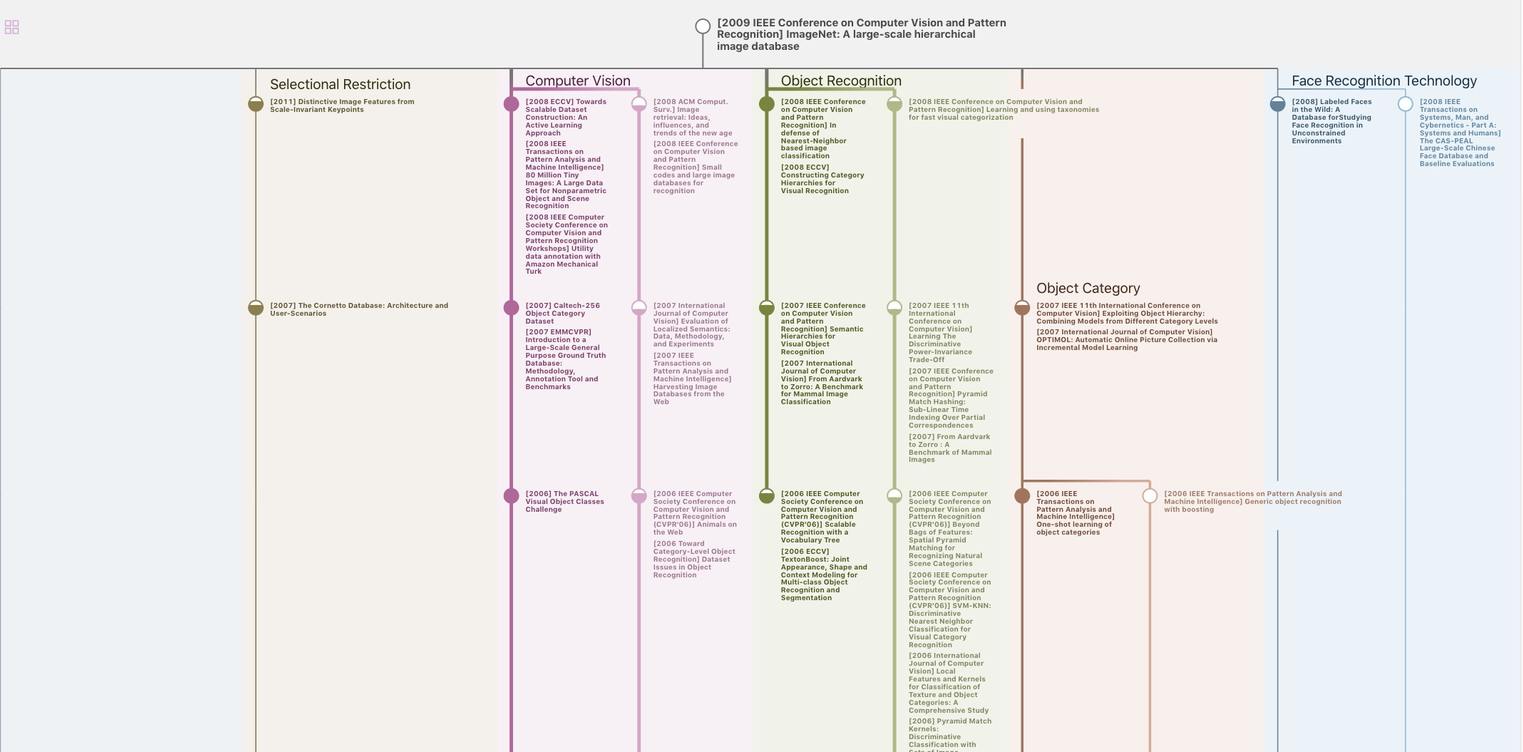
生成溯源树,研究论文发展脉络
Chat Paper
正在生成论文摘要