A Simple Yet Effective Data Augmentation for Self-Supervised Multi-stage Centrifugal Pump (MCP) Anomaly Detection
2023 IEEE Seventh Ecuador Technical Chapters Meeting (ECTM)(2023)
摘要
Anomaly detection tasks benefit from self-supervised learning techniques, where models predict or reconstruct parts of augmented input data without relying on explicit human-provided labels. However, many existing self-supervised learning methods employ data augmentation techniques without sufficient justification for their effectiveness. In this study, we present a simple but novel data augmentation method under the context of anomaly detection, obviating the requirement for additional unjustified augmentation techniques. In this method, we leverage data from other operating conditions as augmentation for a specific operating condition, aiming to cluster normal samples across diverse operating conditions. Our approach incorporates the BYOL method as the self-supervised learning framework, coupled with our proposed data augmentation technique for pretraining. Subsequently, we employ the one-class support vector machine (OCSVM) in an anomaly detection experiment involving a Multi-stage Centrifugal Pump (MCP). The evaluation of our method yields promising results, achieving an accuracy of over 83% in two tested operating conditions. This highlights the effectiveness of our approach in accurately detecting anomalies in different operating conditions.
更多查看译文
关键词
anomaly detection,self-supervised learning,data augmentation
AI 理解论文
溯源树
样例
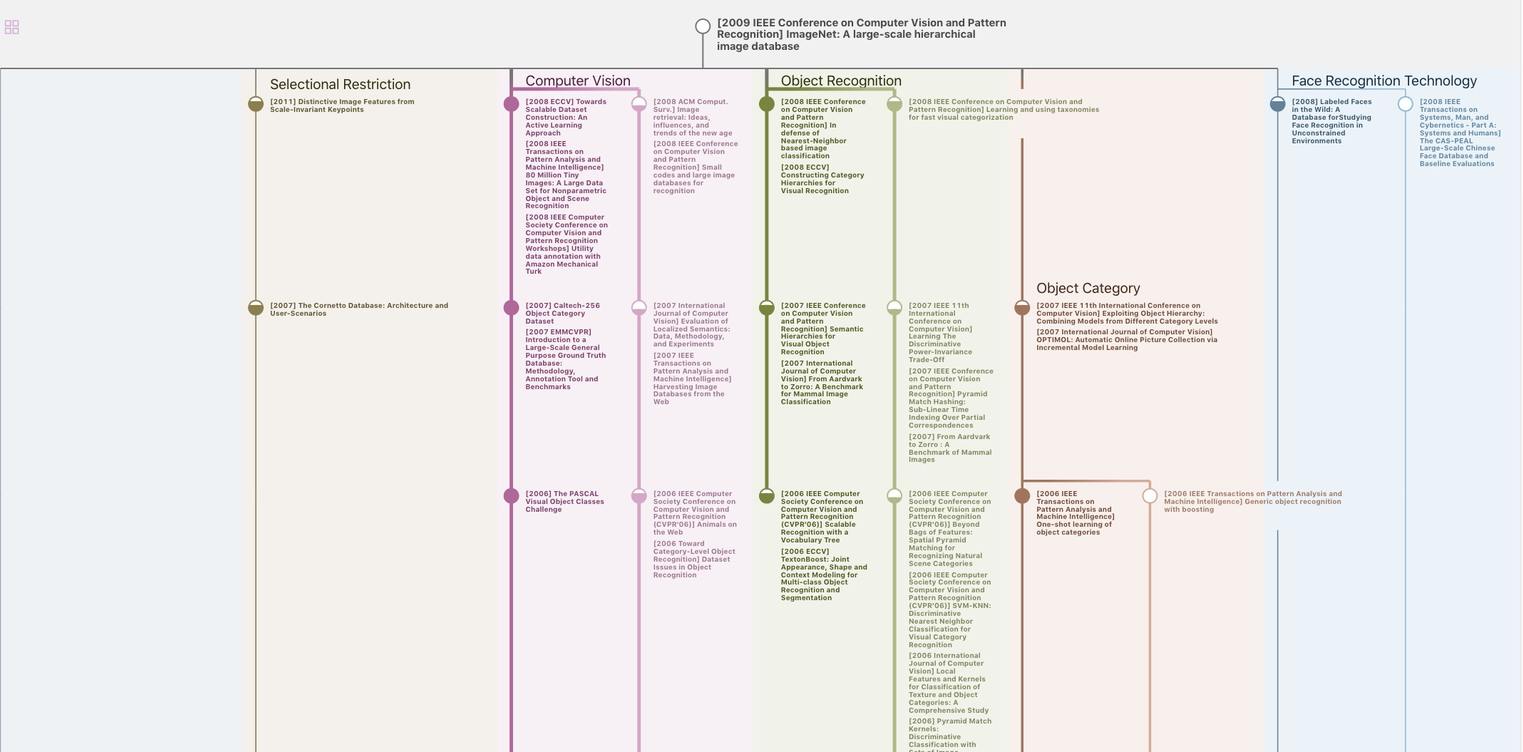
生成溯源树,研究论文发展脉络
Chat Paper
正在生成论文摘要