TCCC Decision Support With Machine Learning Prediction of Hemorrhage Risk, Shock Probability
MILITARY MEDICINE(2023)
摘要
Introduction Expected future delays in evacuation during near-peer conflicts in remote locales are expected to require extended care including prolonged field care over hours to days. Such delays can increase potential complications, such as insufficient blood flow (shock), bloodstream infection (sepsis), internal bleeding (hemorrhage), and require more complex treatment beyond stabilization. The Trauma Triage Treatment and Training Decision Support (4TDS) system is a real-time decision support system to monitor casualty health and identify such complications. The 4TDS software prototype operates on an Android smart phone or tablet configured for use in the DoD Nett Warrior program. It includes machine learning models to evaluate trends in six vital signs streamed from a sensor placed on a casualty to identify shock probability, internal hemorrhage risk, and need for a massive transfusion. Materials and Methods The project team used a mixed methods approach to create and evaluate the system including literature review, rapid prototyping, design requirements review, agile development, an algorithm '' silent test,'' and usability assessments with novice to expert medics from all three services. Results Both models, shock (showing an accuracy of 0.83) and hemorrhage/massive transfusion protocol, were successfully validated using externally collected data. All usability assessment participants completed refresher training scenarios and were able to accurately assess a simulated casualty's condition using the phone prototype. Mean responses to statements on evaluation criteria [e.g., fit with Tactical Combat Casualty Care (TCCC), ease of use, and decision confidence] fell at five or above on a 7-point scale, indicating strong support. Conclusions Participatory design ensured 4TDS and machine learning models reflect medic and clinician mental models and work processes and built support among potential users should the system transition to operational use. Validation results can support 4TDS readiness for FDA 510k clearance as a Class II medical device.
更多查看译文
关键词
hemorrhage risk,machine learning prediction,machine learning
AI 理解论文
溯源树
样例
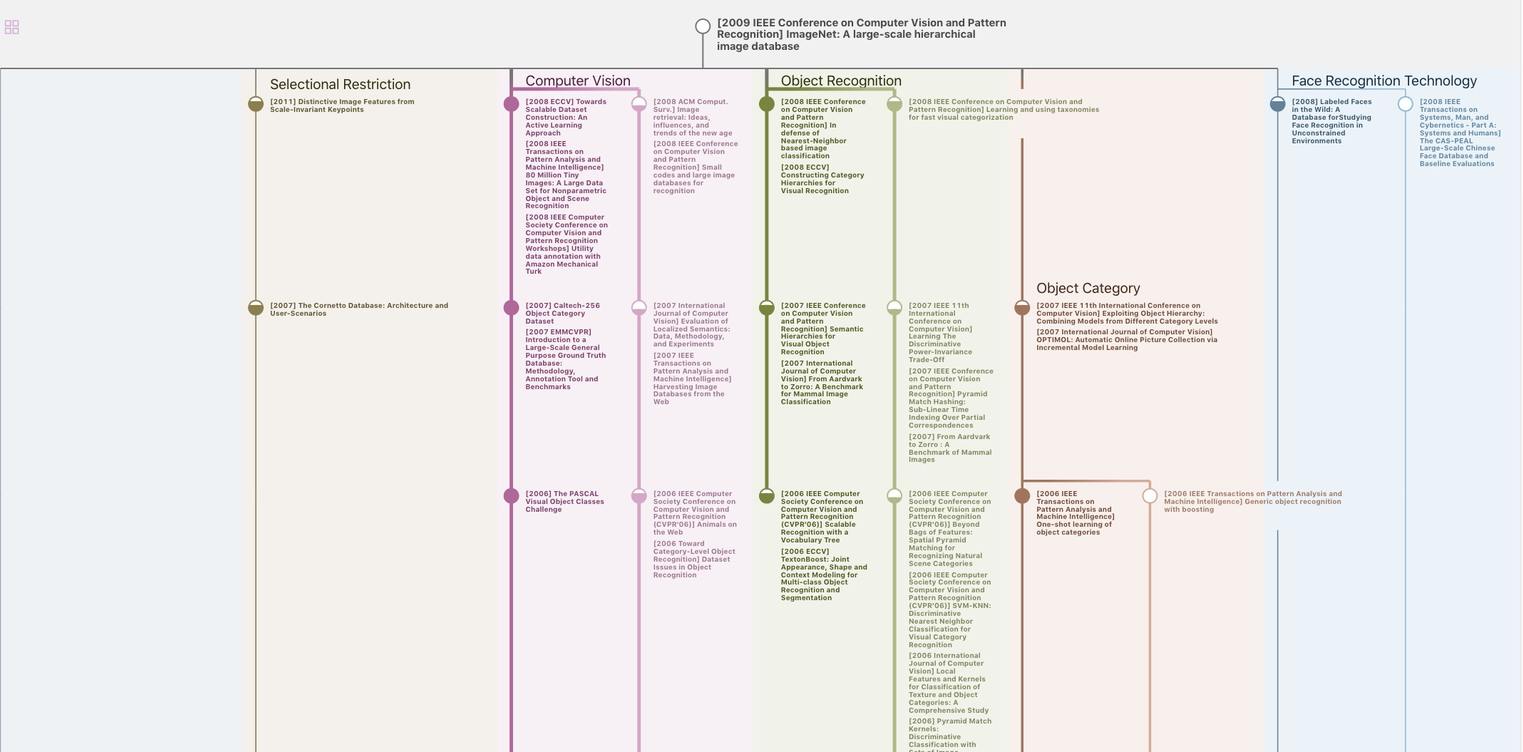
生成溯源树,研究论文发展脉络
Chat Paper
正在生成论文摘要