Machine Learning in Transfusion Medicine: A Scoping Review.
Transfusion(2023)
摘要
Blood transfusion is a routine medical procedure in hospitals with over 2 million blood products transfused in the UK every year at a cost of over £300 million and a median national rate of 34 packed red cells per 1000 population in Europe.1, 2 A blood transfusion can be life-saving but can also cause harm.3 Repeated studies have demonstrated a gap between recommended blood use and clinical practice.4, 5 National challenges with blood stock shortages highlight the need to optimize our current approach to identify who requires and benefits from blood components.6 Recent advances in digital technology offer a wealth of new tools, which can help improve clinical practice as well as improving both the equality and equity of healthcare. Patient Public Involvement groups consistently support better use of data and better understanding of how it might improve efficiencies, prioritizing the need for healthcare professionals to engage with research optimizing use of data. Machine learning (ML) is a subfield of artificial intelligence (AI), which offers the ability to integrate complex and varied data types and could support clinician decision-making, aid personalized care, and, with additional work, improve patient outcomes.7, 8 This field is a rapidly advancing one, which has the potential to revolutionize patient blood management (PBM). Successful implementation of ML to support clinical workflows requires collaboration between computer scientists and clinicians. Key features of ML have been described elsewhere, which support informed interpretation of the literature.9, 10 The majority of work applying ML to healthcare uses supervised learning whereby the model is trained on input features and labeled output features to enable predictions on unseen examples (Figure 1).11 Model performance evaluation uses metrics, which summarize prediction quality, for example, the area under the receiver operating characteristic curve (AUROC) for classification models. The two other main categories of ML approaches are unsupervised learning, which identifies patterns in unlabeled data (e.g., finding clusters of similar patients), and reinforcement learning, an approach to learning how to act through trial and error. To be useful in practice, models need to be validated and integrated into a clinical workflow, where capacity constraints and users ignoring alerts may limit the impact of even a perfectly performing model.12 The purpose of this review is to collate the breadth of literature of ML in transfusion medicine, describing current trends and capturing key methodological approaches, adding to the recognized need for up-to-date discussion of the challenges and potential solutions to the prospective implementation of ML in transfusion medicine.13 The review aimed to report on original research articles, using ML approaches with a focus on transfusion medicine. We followed the approaches of a scoping review used by Cochrane from the Canadian Institutes of Health Research, defined as “exploratory projects that systematically map the literature available on a topic, identifying key concepts, theories, sources of evidence, and gaps in the research.”14 Eligibility for studies was defined by blood transfusion in humans (or the support of transfusion) as the main outcome. There were no restrictions on year of publication, publication status, or language. We excluded studies using linear or logistic regression (LR) primarily for statistical inference and/or to construct a predictive risk score. This exclusion is consistent with a recent systematic review on the impact of ML on patient care.15 When considering inventory management in a hospital blood bank, we focused on recent work using patient data from electronic health records and, therefore, excluded research that predicted future demand based solely on historic demand. As it is common in ML and computer science to submit full-length works to top tier conferences instead of journals, where reports met all other criteria, conference articles were included as full text (n = 3). We searched the Clarivate Web of Science database on January 4, 2023 with the following search terms: [TS = (machine learning OR artificial intelligence OR forecast* OR algorithm OR prediction model OR predictive model OR neural network)] AND TS = {transfus* OR blood product OR blood bank OR [reaction NEAR (blood OR transfus*)]}. We reviewed the lists of publications in the literature and consulted with all authors. Additional citation search was performed and relevant reports were added, which were not captured in the initial Web of Science search. The title and abstract screen were conducted in duplicate (SM and JF). Differences were resolved by consensus or with a third reviewer, to arrive at the final set for full-text review. Where the same work was published in more than one journal (i.e., not a true duplicate, but papers aimed at different audiences), we selected the journal with a medical focus where possible for inclusion in the summary tables and figures. Data were extracted in duplicate (SM and JF), with discrepancies resolved by a third reviewer. Results were presented descriptively. Initial clinical categories were defined and agreed based on understanding of the literature and were further refined following title and abstract screening. We extracted information on clinical applications, data sources, and ML methods. The research team also predefined a range of factors identified as important when considering the methodology and exploring the opportunities and limitations of translating ML models to a healthcare setting.16-18 Meta-analysis of the results was not undertaken due to the wide range of different tasks, variability in definitions for similar tasks, and reporting heterogeneity. A total of 4504 publications were retrieved using the described search strategy performed on January 4, 2023 (Figure 2). Initial screening returned 107 citations, and 93 articles were selected for inclusion in the study following full-text review, including the addition of two articles identified through citation searching. Overall, 16 studies eligible for full-text review were excluded: Three were duplicates captured through alternative journal publications, three did not meet ML criteria, transfusion was not the main outcome for seven, and the full-text article was not available for two studies. One article was removed due to subsequent publication retraction. The temporal distribution of 93 included publications is shown in Figure 3. There is a clear trend toward increasing frequency of publications over time with 56% (52/93) of the articles published in the last 3 years. The majority of studies were focused on prediction of transfusion (58%) with other key areas of ML application identified within transfusion safety (22%), hospital blood bank (10%), and supporting transfusion decisions (10%) (Figure 4A). Within prediction of transfusion (Figure 4B), a significant majority of studies were in the setting of surgery (61% 33/54), followed by trauma (24% 13/54). In the remaining eight studies, ML was deployed in the setting of obstetrics, gastrointestinal bleeding, and hemato/oncology, and in three studies applied more broadly to all inpatients and intensive care, captured as “other hospital settings.” The objectives, sample size, and key findings of all studies within these broad categories of clinical settings are provided in Table 1 and more detailed methodological considerations in Table 2. Overall clinical applications, trends, and a summary of main findings are discussed in more detail under the relevant subheading below. 220 (AAA) c.175 (non-AAA) Overall, the most common countries for identified studies are the United States (44), followed by China (16), Europe (12), and Canada (6). The range of sample sizes reported in the studies varied from 41 to more than 4 million (Table 1). Packed red blood cells (PRBCs) were the focus of approximately half of the studies (46/93), with most of the remainder considering either multiple blood products (22%) or not specifying the blood products (22%). Five studies (5%) considered only platelets, and two studies (2%) considered only plasma. The majority of identified studies employed ML to predict transfusion related to a specific specialty or procedure, notably within orthopedics,19-25 cardiac surgery,26-30 spinal surgery,31-34 and liver transplant,35-37 focusing on a specific procedure or a variety within that specialty (Table 2). A small number of studies consider procedures from multiple specialties38-44 with Walczak and Velanovich43 including 56 different surgeries from the publicly available United States National Surgical Quality Improvement Program (NSQIP). Their use of single models to predict transfusions for a wide variety of surgical procedures could provide a much simpler approach rather than individual models for each surgical procedure. Some researchers interrogated models to identify features to help predict PRBC transfusion22, 32, 34, 36 or the decision to transfuse44 as examples of hypothesis generation from ML. Five studies developed online risk calculators and web apps based on their models.24, 30, 32, 45, 46 Gurm et al.30 highlighted that previous simplified noncomputerized tools need no longer be the limit to what can be utilized in clinical medicine; however, a recent systematic review concluded that the resultant clinical prediction models for blood transfusion in elective surgery are of a high risk of bias and often fail to adhere to reporting standards, emphasizing caution before application to clinical practice.47 There is an extensive body of literature developing risk scores for transfusion in trauma patients, and multiple reviews suggest further model development and/or validation is required.48-51 Two key challenges with trauma are the potentially large requirements of blood for a small proportion of patients52 and the importance of a fast response.53 The activation of the massive transfusion protocol (MTP) is resource intensive and may result in product wastage in cases of false positive activation.54 The ability to predict future transfusion requirements prior to hospital arrival can support triage decisions and help to ensure that blood products are available when required on arrival.55-57 When making predictions using data collected at the hospital, research has focused on four related prediction tasks: predicting transfusion,58, 59 the number of units transfused,60 activation of the MTP,54 and/or massive transfusion.58, 61-63 The model developed by Mina et al.54 to predict MTP activation was integrated into a smartphone application, externally validated and an implementation and prospective validation study was conducted at the initial site. Clinicians informed of the model's prediction made better decisions in the prospective validation study.54, 64, 65 This is a key demonstration of how we expect such models will eventually be used in practice: supplementing rather than replacing clinical judgment. Demand for blood components and associated morbidity and mortality are significant in obstetrics, gastrointestinal bleeding, and hemato/oncology1, 5, 66; however, ML for prediction of transfusion in these settings is underrepresented comprising a total of 5 of 54 studies, none of which have undergone prospective validation or implementation at the time of writing. The studies exploring gastrointestinal bleeding demonstrate benefits of using large, publicly available data sets, able to externally validate models.67, 68 Given the availability of the data, these tasks could be developed into benchmarks, enabling different research teams to compare the performance of new approaches. Interestingly, Levi et al.67 apply their model to support triage: predicting which patients do not require transfusion (suggesting no ongoing bleeding) and, therefore, may avoid admission to intensive care unit (ICU). Shung et al.68 highlight the potential impact of alert fatigue in the context of repeated predictions on a problem with relatively low frequency. Lee et al.69 and Ghassemi et al.70 predict blood transfusion within the ICU, respectively, demonstrating the inadequacy of hemoglobin measurement alone as a determinate of transfusion and that general patient state representations could be used to better predict platelet and plasma transfusions. Review of all studies suggested that task-specific performance of ML for predicting transfusion need is frequently reported with AUROC >0.8 (Table 1). In 13 studies that reported a direct task-matched comparison of ML to LR models, LR matched or outperformed ML in 54% (Table 1). However, in additional seven studies, ML was reported to demonstrate measurable clinical improvements such as cost savings or performance over current scoring systems (Table 1). Beyond prediction of the likelihood of transfusion, ML can identify inappropriate transfusions, recognize patient groups by predicted transfusion outcomes, and enable precise dosing of blood products in efforts to reduce iron overload.71, 72 Through the analysis of existing clinical trials data, ML enabled estimates of the causal effect of preoperative plasma transfusion on perioperative bleeding in patients with a high International Normalized Ratio test result73 and of different ratios of platelets and plasma relative to PRBC on mortality and hemostasis in trauma patients.74 Bruun-Rasmussen et al.75 used ML to emulate a randomized controlled trial in the context of sex-matched transfusion policy. Ngufor et al.76 take a key step toward personalized medicine, clustering patients using unsupervised ML to determine whether they will benefit from plasma transfusion. Models to identify inappropriate transfusions may reduce the labor required for retrospective quality control77 and support local efforts to reduce unnecessary orders and transfusions.78 It may also be possible to identify situations where ongoing transfusion is futile, but this has proved challenging.79 Identified studies in this category were divided into hemovigilance and laboratory support in the Blood Bank. ML has been applied primarily to enhance the ability to detect and predict acute transfusion reactions (ATRs) and adverse transfusion events. Novel information retrieval methods, such as natural language processing (NLP), when applied to electronic health records (EHRs) have demonstrated underreporting by clinicians and the potential to improve detection.80-82 Alternatively, Roubinian et al.83 and Nguyen et al.84 incorporated novel biomarkers into classification models and decision tree analysis, respectively. While the focus of ML is on prediction, and a causal relationship cannot be assumed of the covariates found to have high predictive value, identification of novel risk factors for hypothesis generation and further research can be useful as seen in transfusion-associated lung injury (TRALI)85 and in pediatric transfusion-associated hyperkalemia.86 Recognizing that transparency and accountability are essential for clinicians in generating hypotheses, Zhu et al.87, 88 focus on explainable AI when presenting adverse events during neonatal hyperbilirubinemia exchange transfusion, particularly through use of SHapley Additive exPlanation (SHAP). In a laboratory setting, 63% (six of eight) studies investigated the use of ML to assist blood group identification including two to help classify antibody reactions where ambiguity in human interpretation exists.89, 90 Doan et al.91 and Kim et al.92 introduced image-based deep learning as a novel approach to perform phenotype assessment of red blood cell storage lesions to predict red cell quality prior to transfusion. The availability of EHRs has supported the development of models to forecast blood product demand and recommend order quantities, based on aggregated patient data in addition to historical demand patterns.93-99 A model implemented in a Canadian hospital for PRBC reduced wastage and daily stockholding,93 and it is common for forecasting models to be implemented into simulations to estimate the potential benefits of deploying them.94-97 In addition to studies supporting ordering, two studies investigated the use of ML to directly address wastage in a hospital blood bank by predicting discards100 and identifying transaction patterns associated with wastage.101 Selected characteristics of the methodology of identified studies are summarized in Table 2. Only 41% of all studies were multisite. Source data were derived from EHRs in 70%, defined as hospital data that were collected routinely without research or audit intent. Alternative data sources included research databases and laboratory primary research data. All but two papers (98%) used supervised learning, while only four papers (4%) used unsupervised learning methods. None of the papers used reinforcement learning. In Table 2, we divide supervised ML methods into three broad groups: tree-based methods, neural networks, and other methods. We describe these groups in the Appendix. If a study used an ensemble of techniques from more than one of these categories, then the underlying techniques are counted as having been used in that study. Tree-based models were included in 68% of the studies and neural networks in 43% of the studies. A common approach taken by many of the studies is to compare several different ML methods readily available in software libraries such as Python's scikit-learn. A small number of papers investigate novel ML ideas including the use of a secondary model to provide a confidence level in predictions57 and using weak labels in the form of age information to train a model to classify red cell quality without relying on subjective human expert labels.91 Only eight studies (9%) reported the results of prospective evaluation or deployment. Of these, four evaluated their models on prospectively collected data,35, 37, 90, 102 one conducted a “shadow test” in which predictions were generated in real time for evaluation but not used for decision-making,56 and three describe implementation as part of live decision-making.65, 78, 93 A majority of studies (65%) included an outcome performance comparator, defined as a logistic or linear regression model, a previously reported method for the same problem, or a baseline representing current practice. Where expected, as with individual-level prediction, reference to a reporting framework was infrequent. Recognized reporting frameworks including TRIPOD and STROBE were utilized in only 11/54 studies within predicting transfusion. None of the work predicts transfusion reactions or adverse events within the transfusion safety subgroup of hemovigilance reported in accordance with a recognized framework. Data were stated to be available in 28% of studies and code in only 12%, which will limit future researchers' ability to reproduce and extend the work performed to date. Yamada et al.86 provided their full data analytic protocol as an electronic notebook, an example supporting reproducibility and open science. Research in the field of ML in transfusion is expanding rapidly with exciting applications as evidenced by the number of publications. However, our review also highlights clear challenges surrounding transparency, interpretability, and generalizability of findings. Most studies are single center and have no prospective validation or implementation. ML model code and data are rarely made available for external validation, and there is limited justification of methods, with best performing models often selected from a trial of those commonly available. Where ML performance characteristics are often encouraging, the authors emphasize caution in interpretation of evidence that models can achieve improved performance as compared to current practice. As within predicting transfusion, ML does not always offer advantage over LR when task-specific performance is compared and demonstrates the difficultly in interpreting the clinical potential of ML while tasks, reporting measures, and methodology remain so variable. While challenging, prospective deployment of a model within the clinical workflow and subsequent evaluation of changes in key performance indicators is highly desirable in ML. Our findings of limited prospective testing and deployment are consistent with those of the wider field where translation remains a challenge, and researchers are producing frameworks103 and sharing case studies104 to help close this gap. A simulated workflow, as developed in recent studies,12, 105 as a method for evaluating the potential impact of a model may help to prioritize candidates for prospective testing. Data were extracted from EHRs or from medical devices in three of the four studies where predictions were made in real time, as part of a live workflow or a “shadow test,”56, 78, 93 while the remaining case required data to be entered manually into a smartphone application.65 The latter may face fewer initial barriers to deployment such challenges involved in integrating different systems, but there is a risk of manual data entry errors and a limit on the quantity and types of data that can be quickly and accurately entered. Recent developments in NLP, such as ChatGPT, may lead to the development of systems that can interactively support decision-making and integrate structured data from EHRs with clinical notes, and brief-written (or transcribed verbal) descriptions of the problem at hand.106 Current variation in transfusion practice, particularly in prediction where the outcome “decision to transfuse” remains clinician-dependent, could perpetuate suboptimal practices. Models may embed class imbalance and generate predictions enriched by patient episodes, which are elsewhere considered to be over-transfused. This problem may be reflected in poor external validation of pretrained models if the sites use different guidance or practice.68 Although considered beyond the scope of this review, it may be of interest to review studies where the contribution of physicians, for example, surgeons and anesthesiologists, features as a variable of the model, or where variables behind physicians' decision-making are explored in more detail,44 to address reasons for variation of practice. Such an approach could prompt action to address discrepancies, particularly as the large, multicenter datasets used for ML are also well suited to address physician effects while preserving anonymity. Clear key performance indicators remain an unmet need in transfusion by which to evaluate clinically relevant outcomes following transfusion in a standardized way. While practice variation impacts the generalizability of trained models, underlying methods may still generalize to new sites if retrained on local data. Additionally, successful integration of a model into the workflow may change patterns that have been learned (e.g., which tests are ordered and how often). It is crucial to continuously monitor the predictions of a deployed model to ensure that its predictions remain valid and useful.107 The ability to fine-tune and update models using local108 and/or more recent data109 offers huge potential advantage of ML-based predictive modeling over historic simplified scores and static prediction rules in addressing these challenges, enabling models to accommodate new clinical trends and evaluate performance as compared to current practice in an iterative manner.23 To our knowledge, this review is the first attempt to collate the literature on a wide range of applications of ML in transfusion medicine. Our analysis extends the work of Meier and Tschoellitsch13 who describe 47 articles of ML applied to PBM (including bleeding and anemia) from a 2021 PubMed search. We have captured information on emerging areas of interest to clinicians and researchers, and by review of ongoing challenges faced in the interpretation and translation of ML, we also offer suggested priorities for future reporting and work. Our study has a number of limitations. The heterogeneity of methods and infrequent use of reporting frameworks makes synthesis of results and interpretation challenging, as well as creating barriers for researchers to build upon and validate outcomes. Researchers should be encouraged to provide work as an online open-source repository and share computational tools.30, 110 Developing common task definitions and following established reporting frameworks would make it easier to compare methods and identify candidates for prospective validation and subsequent implementation. Secondly, in setting out to give an overview of the literature, the volume of publications captured while maintaining broad search terms meant it was beyond the scope of this review to extend to multiple databases and we acknowledge that relevant studies may have been missed. Citation review was performed in efforts to minimize this. Further studies might benefit from more focused reviews on selected themes in transfusion medicine. Lastly, while we apply the “main outcome of transfusion” to identify studies to support PBM, we recognize that the concept of PBM goes well beyond this such as optimization of anemia (e.g., erythropoietin therapy and iron therapy) and that these areas deserve exploration in future studies. As the body of literature of ML in transfusion and PBM grows, so will the potential for more focused systematic reviews. This review adds to the continuously evolving, contemporaneous studies and reviews essential to engage clinicians new to the idea of ML.15, 111 There has been a major expansion of the literature in recent years, reflecting the interest and enthusiasm toward the application of ML in transfusion medicine. However, many challenges and limitations remain to include data quality and access, adherence to (and existence of) appropriate reporting frameworks, and generalizability of findings. Emphasis should be on consistent reporting, sharing of code, and prospective validation with comparison to current practice of future studies. This study was supported by the NIHR Blood and Transplant Research Unit in Data Driven Transfusion Practice (NIHR203334), UKRI Training Grant No. EP/S021612/1, the Centre for Doctoral Training in AI-enabled Healthcare Systems, and the NIHR University College London Hospitals Biomedical Research Centre. The views expressed are those of the author(s) and not necessarily those of the NIHR or the Department of Health and Social Care. The authors have disclosed no conflicts of interest. In Table 2 of the main paper, we divide supervised machine learning methods into three broad groups: tree-based methods, neural networks, and other methods. Tree-based methods include classification and regression tree (CART), a single decision tree, and methods that train an ensemble of trees in parallel (e.g., random forests [RF]) or sequentially (e.g., gradient boosting machines, XGBoost, and CatBoost). Neural networks consist of layers of “neurons” inspired by biologic neurons and include fully connected neural networks (FCNNs), in which each “neuron” receives input from every “neuron” in the preceding layer, and alternative architectures that have been developed for different types of input data including convolutional neural networks (CNNs) for images and recurrent neural networks (e.g., the long short-term memory [LSTM] network) for sequences. Our final category includes any methods that are not based on decision trees and are not neural networks including generalized linear models (e.g., logistic regression and linear regression), support vector machines, naïve Bayes, K-nearest neighbors, and Markov chains.
更多查看译文
关键词
Blood Transfusion,Medical Imaging
AI 理解论文
溯源树
样例
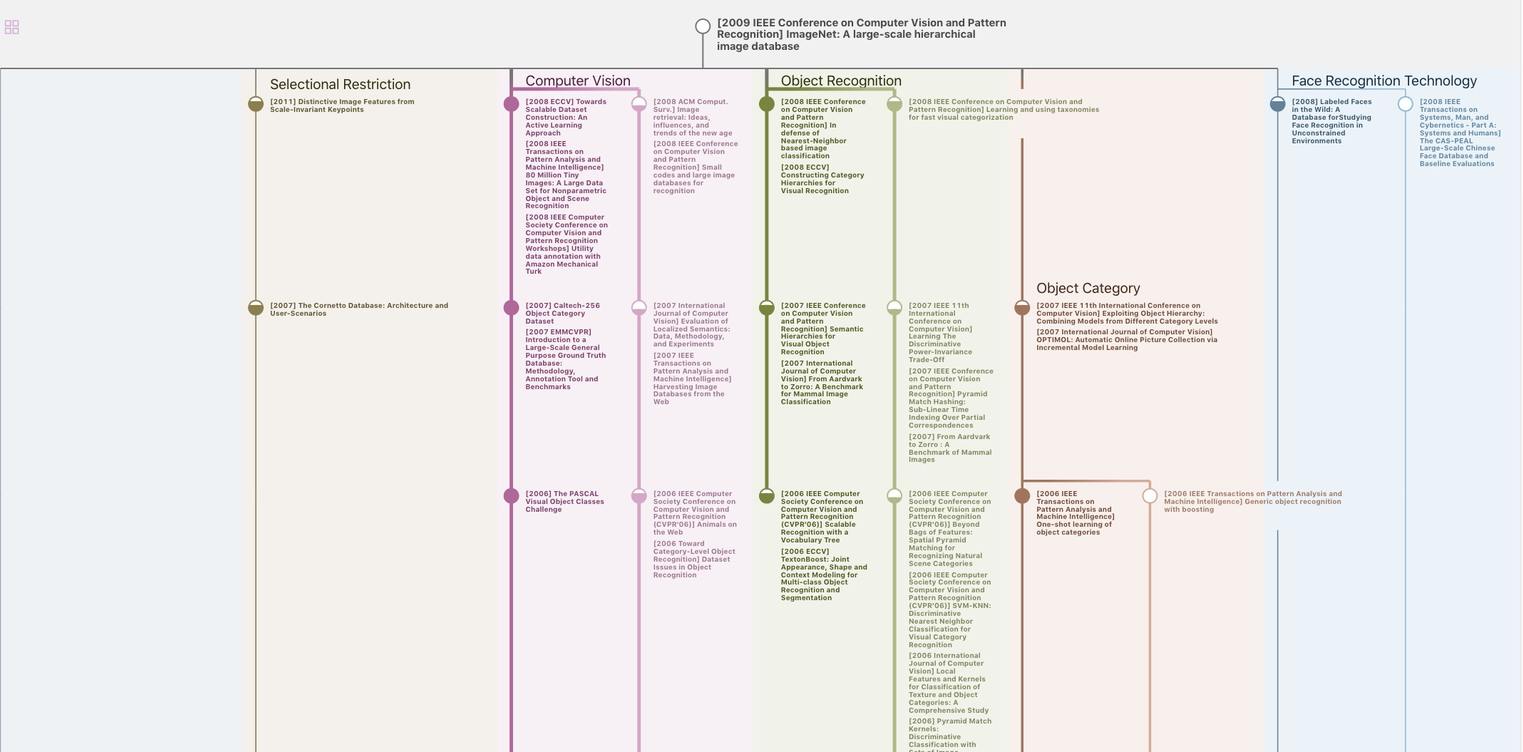
生成溯源树,研究论文发展脉络
Chat Paper
正在生成论文摘要